Identifying and Compensating for Feature Deviation in Imbalanced Deep Learning
arxiv(2022)
摘要
Classifiers trained with class-imbalanced data are known to perform poorly on test data of the "minor" classes, of which we have insufficient training data. In this paper, we investigate learning a ConvNet classifier under such a scenario. We found that a ConvNet significantly over-fits the minor classes, which is quite opposite to traditional machine learning algorithms that often under-fit minor classes. We conducted a series of analysis and discovered the feature deviation phenomenon -- the learned ConvNet generates deviated features between the training and test data of minor classes -- which explains how over-fitting happens. To compensate for the effect of feature deviation which pushes test data toward low decision value regions, we propose to incorporate class-dependent temperatures (CDT) in training a ConvNet. CDT simulates feature deviation in the training phase, forcing the ConvNet to enlarge the decision values for minor-class data so that it can overcome real feature deviation in the test phase. We validate our approach on benchmark datasets and achieve promising performance. We hope that our insights can inspire new ways of thinking in resolving class-imbalanced deep learning.
更多查看译文
关键词
imbalanced deep
AI 理解论文
溯源树
样例
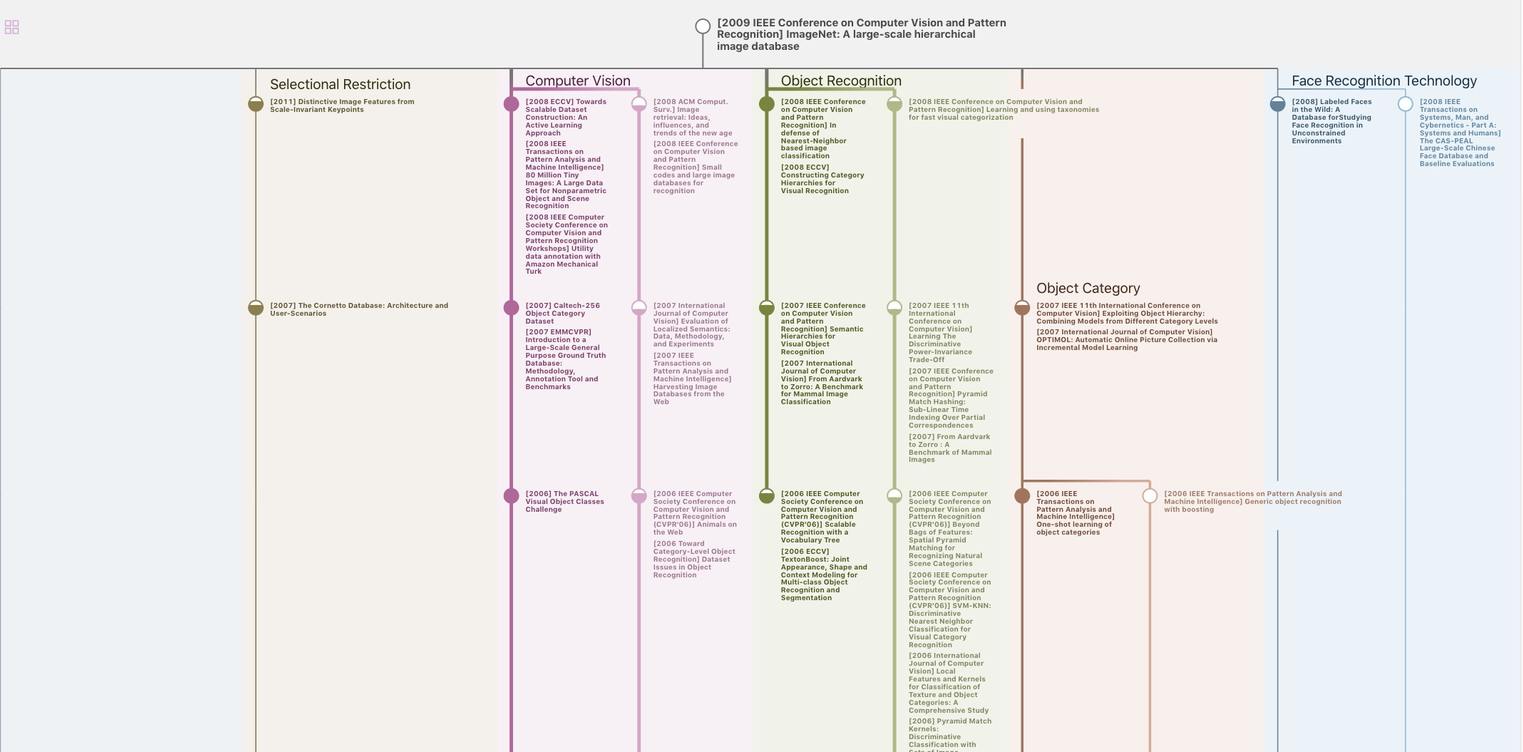
生成溯源树,研究论文发展脉络
Chat Paper
正在生成论文摘要