Stagioni: Temperature management to enable near-sensor processing for energy-efficient high-fidelity imaging
arxiv(2019)
摘要
Vision processing on traditional architectures is inefficient due to energy-expensive off-chip data movement. Many researchers advocate pushing processing close to the sensor to substantially reduce data movement. However, continuous near-sensor processing raises the sensor temperature, impairing the fidelity of imaging/vision tasks. We characterize the thermal implications of using 3D stacked image sensors with near-sensor vision processing units. Our characterization reveals that near-sensor processing reduces system power but degrades image quality. For reasonable image fidelity, the sensor temperature needs to stay below a threshold, situationally determined by application needs. Fortunately, our characterization also identifies opportunities -- unique to the needs of near-sensor processing -- to regulate temperature based on dynamic visual task requirements and rapidly increase capture quality on demand. Based on our characterization, we propose and investigate two thermal management strategies -- stop-capture-go and seasonal migration -- for imaging-aware thermal management. We present parameters that govern the policy decisions and explore the trade-offs between system power and policy overhead. Our evaluation shows that our novel dynamic thermal management strategies can unlock the energy-efficiency potential of near-sensor processing. For our evaluated tasks, our strategies save up to 53% of system power with negligible performance impact and sustained image fidelity.
更多查看译文
AI 理解论文
溯源树
样例
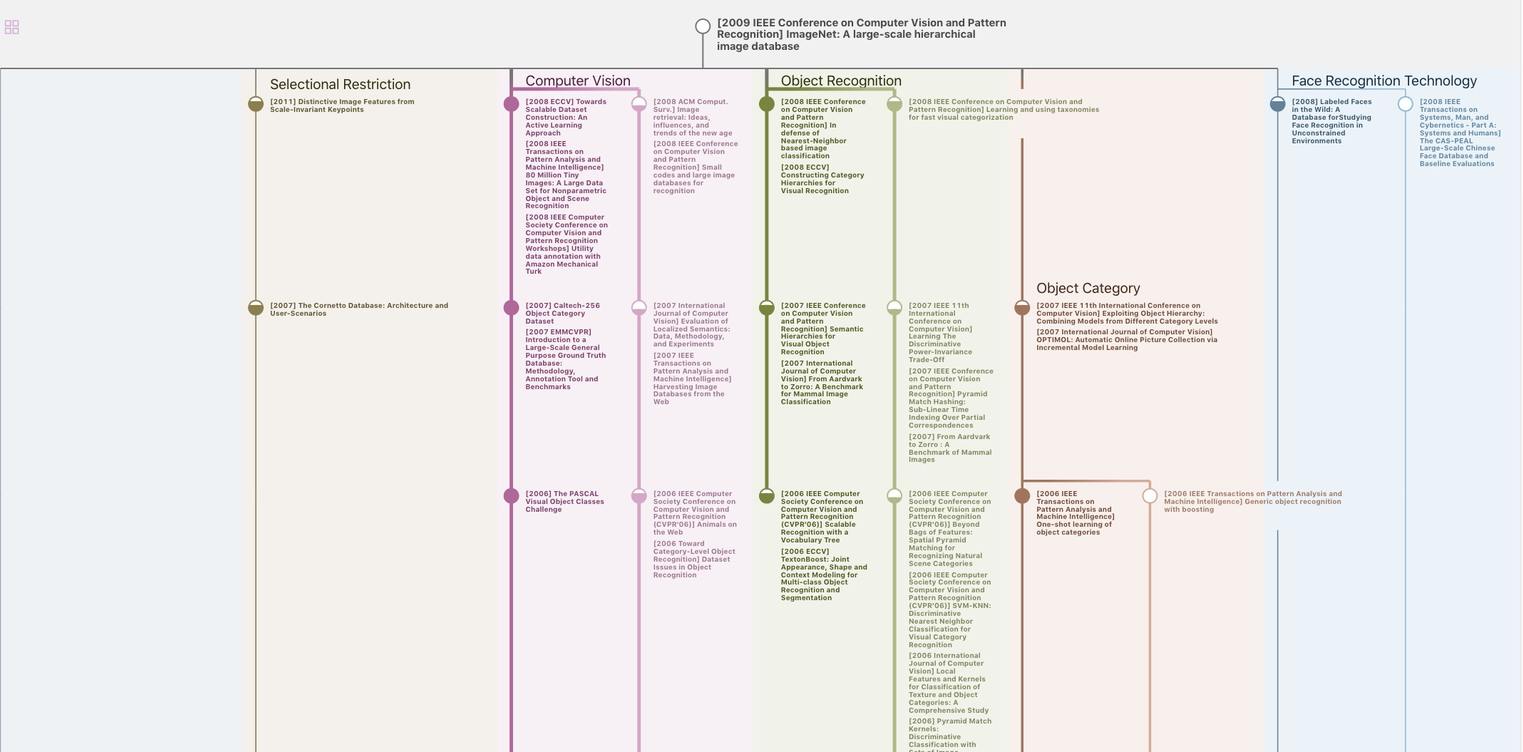
生成溯源树,研究论文发展脉络
Chat Paper
正在生成论文摘要