A Nonparametric Off-Policy Policy Gradient
INTERNATIONAL CONFERENCE ON ARTIFICIAL INTELLIGENCE AND STATISTICS, VOL 108(2020)
摘要
Hein orcernent, learning' algorithms still suffer from high sample complexity despite outstanding recent successes. The need for intensive interactions with the environment is especially observed in many widely popular policy gradient algorithms that perform updates using on-policy samples. The price of such inefficiency becomes evident in real world scenarios such as interaction-dri yell robot learning, where the success been rather limited. We address this by building on the general sample eflcienc Of off-policy algorithms. With nottparanwtric regression and density estimation methods we construct a nonparametric Bellman equation in a principled manner, which allows us to obtain closed-form estimates of the value function, and to analytically express the ruff policy gradient. a theoretical analysis of our estimate to show that it is consistent, under mild smoothness assumptions and empirically show that our approach has better sample efficiency than stato-ol'-the-art policy gradient methods.
更多查看译文
关键词
gradient,off-policy
AI 理解论文
溯源树
样例
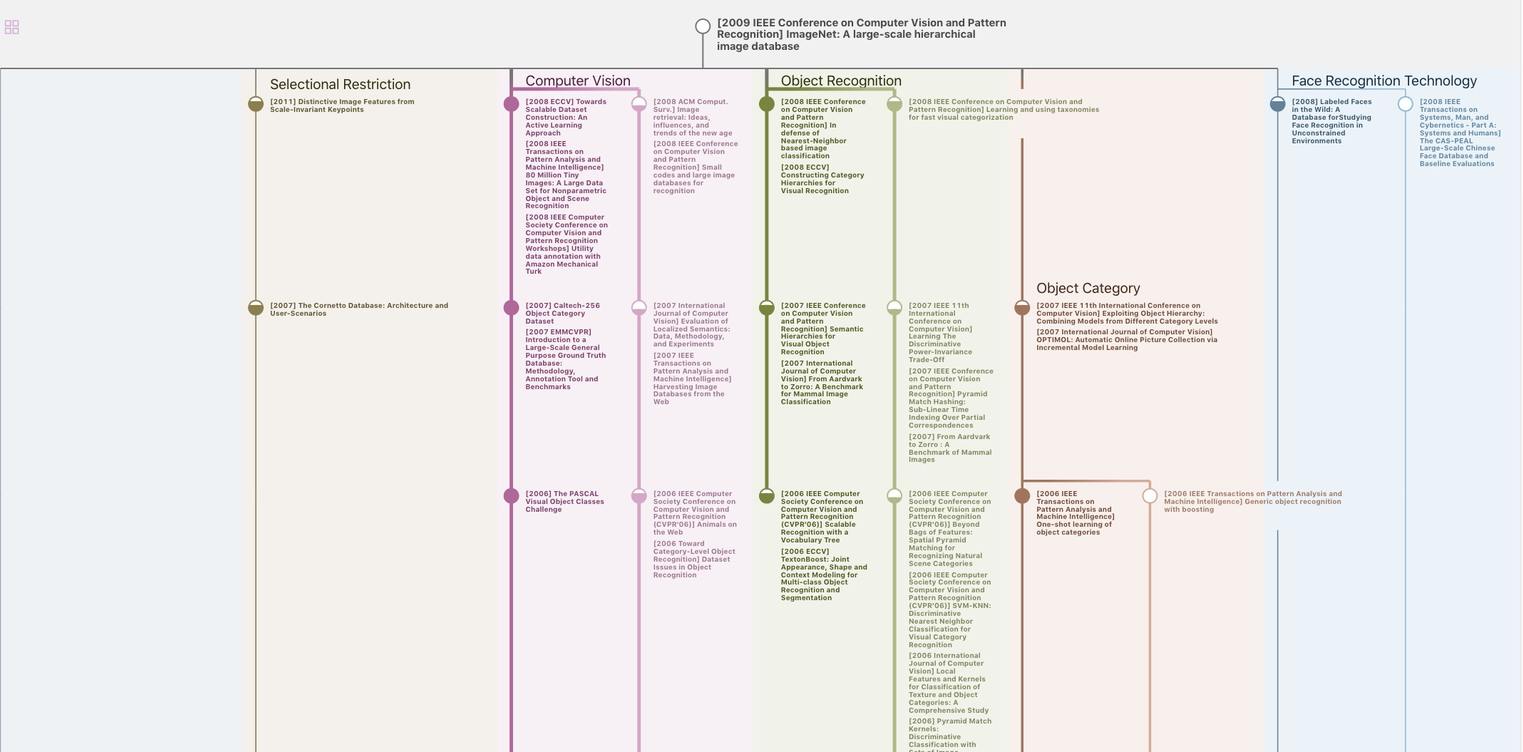
生成溯源树,研究论文发展脉络
Chat Paper
正在生成论文摘要