Streaming automatic speech recognition with the transformer model
ICASSP(2020)
摘要
Encoder-decoder based sequence-to-sequence models have demonstrated state-of-the-art results in end-to-end automatic speech recognition (ASR). Recently, the transformer architecture, which uses self-attention to model temporal context information, has been shown to achieve significantly lower word error rates (WERs) compared to recurrent neural network (RNN) based system architectures. Despite its success, the practical usage is limited to offline ASR tasks, since encoder-decoder architectures typically require an entire speech utterance as input. In this work, we propose a transformer based end-to-end ASR system for streaming ASR, where an output must be generated shortly after each spoken word. To achieve this, we apply time-restricted self-attention for the encoder and triggered attention for the encoder-decoder attention mechanism. Our proposed streaming transformer architecture achieves 2.7% and 7.0% WER for the ``clean'' and ``other'' test data of LibriSpeech, which to the best of our knowledge is the best published streaming end-to-end ASR result for this task.
更多查看译文
关键词
automatic speech recognition,speech recognition,transformer model
AI 理解论文
溯源树
样例
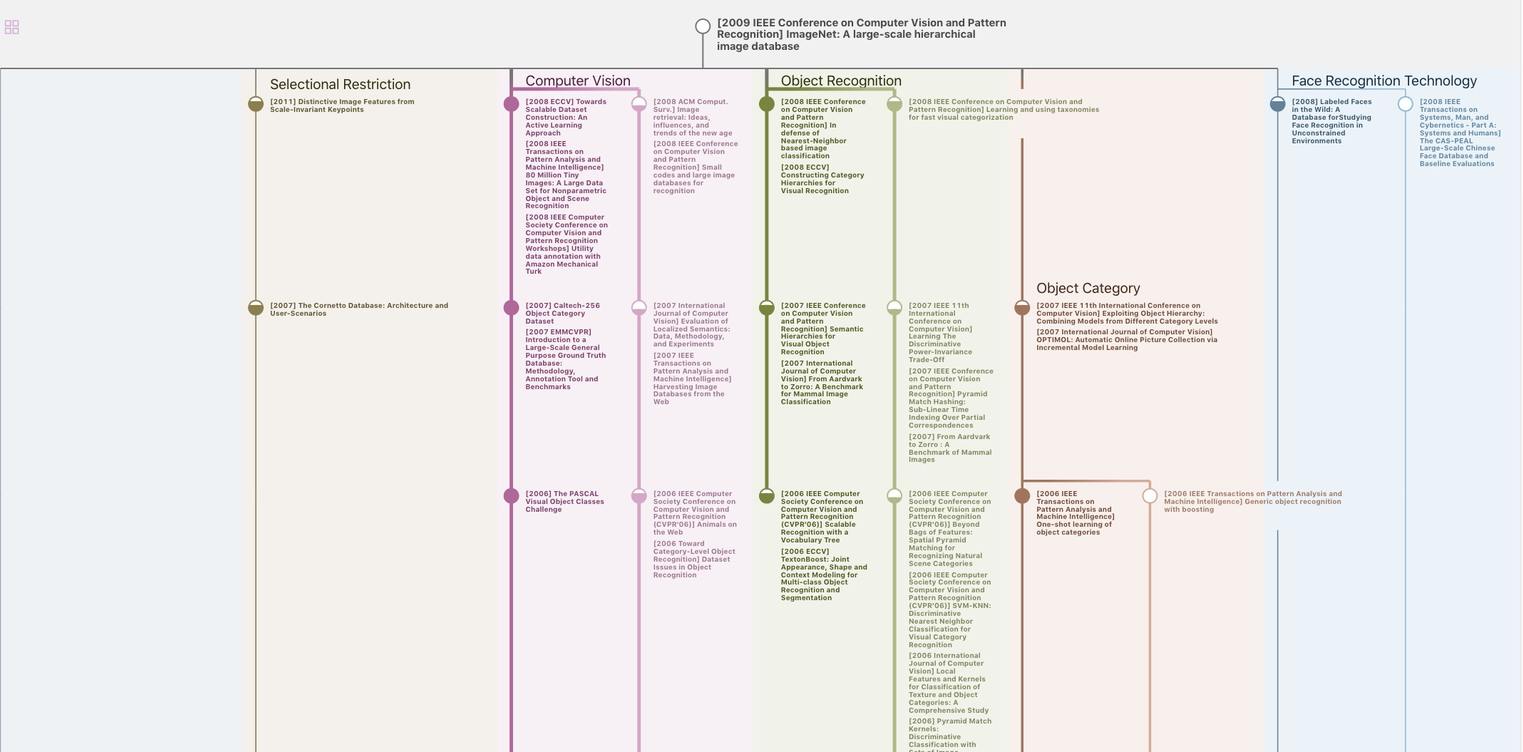
生成溯源树,研究论文发展脉络
Chat Paper
正在生成论文摘要