A step towards information extraction: Named entity recognition in Bangla using deep learning.
JOURNAL OF INTELLIGENT & FUZZY SYSTEMS(2019)
摘要
Information Extraction allows machines to decipher natural language through using two tasks: Named Entity Recognition and Relation Extraction. In order to build such a system for Bangla Language, in this work a Named Entity Recognition (NER) System is proposed, which requires a minimum information to deliver a decent performance having less dependency on handcrafted features. The proposed model is based on Deep Learning, which is accomplished through the use of a Densely Connected Network (DCN) in collaboration with a Bidirectional-LSTM (BiLSTM) and word embedding, i.e., DCN-BiLSTM. Such a system, specific to the Bangla language, has never been done before. Furthermore, a unique dataset was made since no Named Entity Recognition dataset exists for Bangla language till date. In the dataset, over 71 thousand Bangla sentences have been collected, annotated, and classified into four different groups using IOB tagging scheme. Those groups are person, location, organization, and object. Due to Bangla's morphological structure, character level feature extraction is also applied so that we can access more features to determine relational structure between different words. This is initially done with the use of a Convolutional Neural Network but is later outperformed by our second approach which is through the use of a Densely Connected Network (DCN). As for the training portion, it has been done for two variations of word embedding which are word2vec and glove, the outcome being the largest vocabulary size known to both models. A detailed discussion in regard to the methodology of the NER system is explained in a comprehensive manner followed by an examination of the various evaluation scores achieved. The proposed model in this work resulted in having a Fl score of 63.37, which is evaluated at Named Entity Level.
更多查看译文
关键词
Named entity recognition,information extraction,word embedding,sequence labelling,Bi-LSTM,densely connected network,Bangla,annotation,dataset,NLP,neural network,character level feature extraction,CNN
AI 理解论文
溯源树
样例
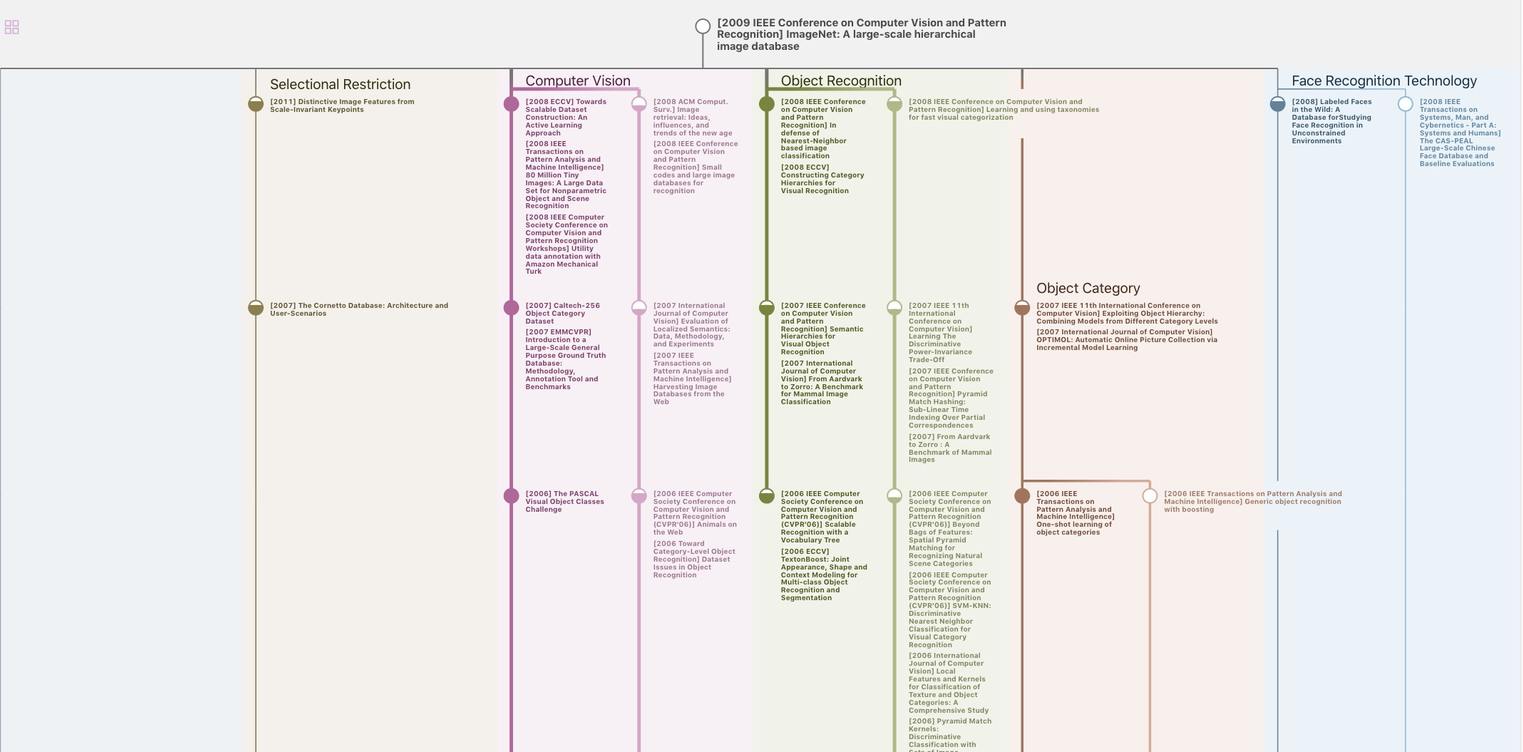
生成溯源树,研究论文发展脉络
Chat Paper
正在生成论文摘要