A novel method for malware detection on ML-based visualization technique.
Computers & Security(2020)
摘要
Malware detection is one of the challenging tasks in network security. With the flourishment of network techniques and mobile devices, the threat from malwares has been of an increasing significance, such as metamorphic malwares, zero-day attack, and code obfuscation, etc. Many machine learning (ML)-based malware detection methods are proposed to address this problem. However, considering the attacks from adversarial examples (AEs) and exponential increase in the malware variant thriving nowadays, malware detection is still an active field of research. To overcome the current limitation, we proposed a novel method using data visualization and adversarial training on ML-based detectors to efficiently detect the different types of malwares and their variants. Experimental results on the MS BIG malware database and the Ember database demonstrate that the proposed method is able to prevent the zero-day attack and achieve up to 97.73% accuracy, along with 96.25% in average for all the malwares tested.
更多查看译文
关键词
Malware detection,Adversarial training,Adversarial examples,Image texture,Data visualization
AI 理解论文
溯源树
样例
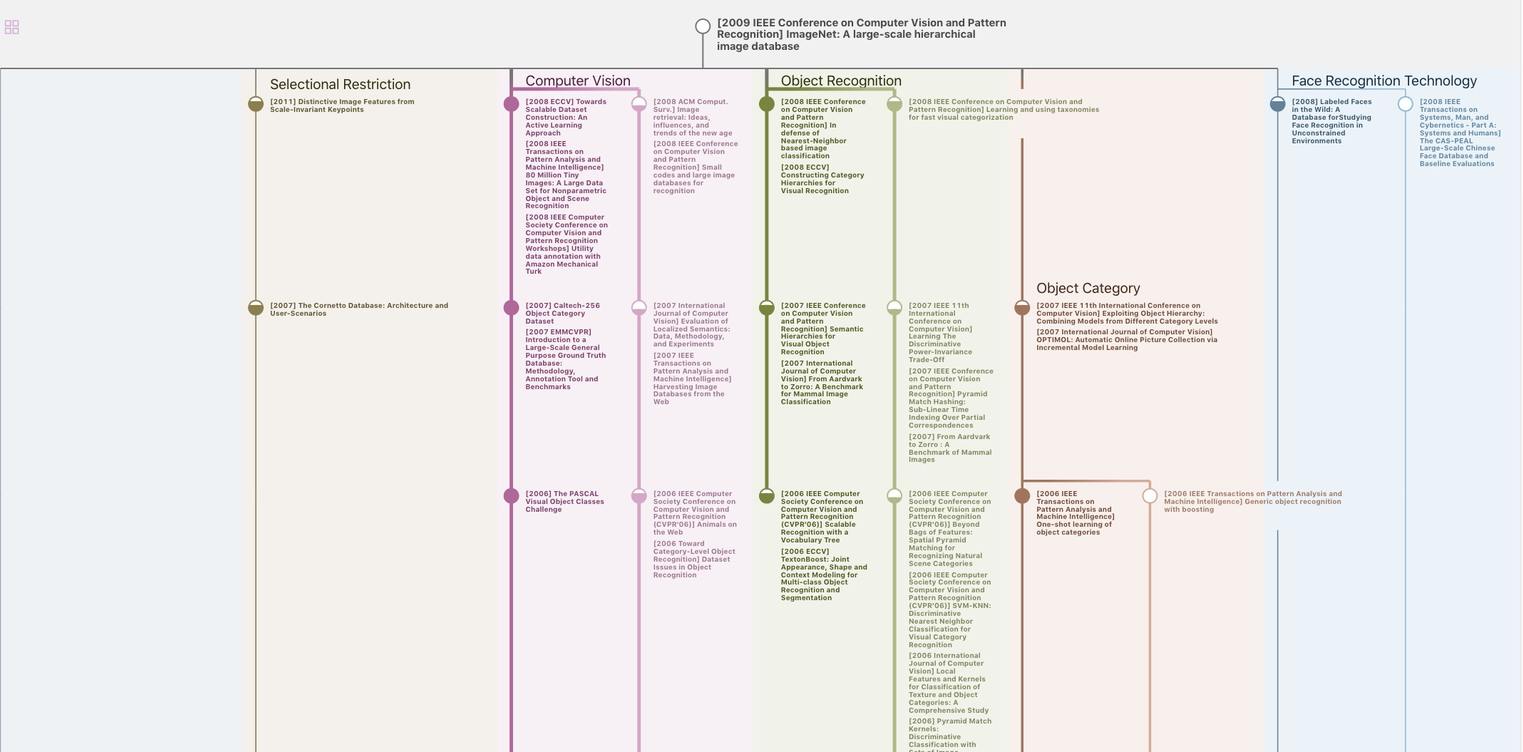
生成溯源树,研究论文发展脉络
Chat Paper
正在生成论文摘要