Comparison of Deep Autoencoder Architectures for Real-time Acoustic Based Anomaly Detection in Assets
2019 10th IEEE International Conference on Intelligent Data Acquisition and Advanced Computing Systems: Technology and Applications (IDAACS)(2019)
摘要
This paper compares several different Autoencoder architectures for unsupervised anomaly detection in acoustic signals. The goal of unsupervised anomaly detection in sound is to detect anomalies without having prior knowledge regarding potential anomalies. Use of autoencoders (AE) to learn a normal model is a state-of-the-art technique for unsupervised anomaly detection. However, the main focus is almost always to increase the difference between the reconstruction error of normal and anomalous data, without taking into account network architecture and speed. This is not a problem when enough computational power is available. However, if the aim is to bring this system to the edge, meaning implementing it on the sensor or close to the sensor, speed and amount of parameters of the network become more important. In this paper we will do a comparative study between different AE architectures. For this comparison both the detection accuracy and the computational complexity will be taken into account. Based on this information it can be decided which AE is most suited to be implemented on hardware for real-time applications.
更多查看译文
关键词
Anomaly detection in sound,deep learning,autoencoder,real-time
AI 理解论文
溯源树
样例
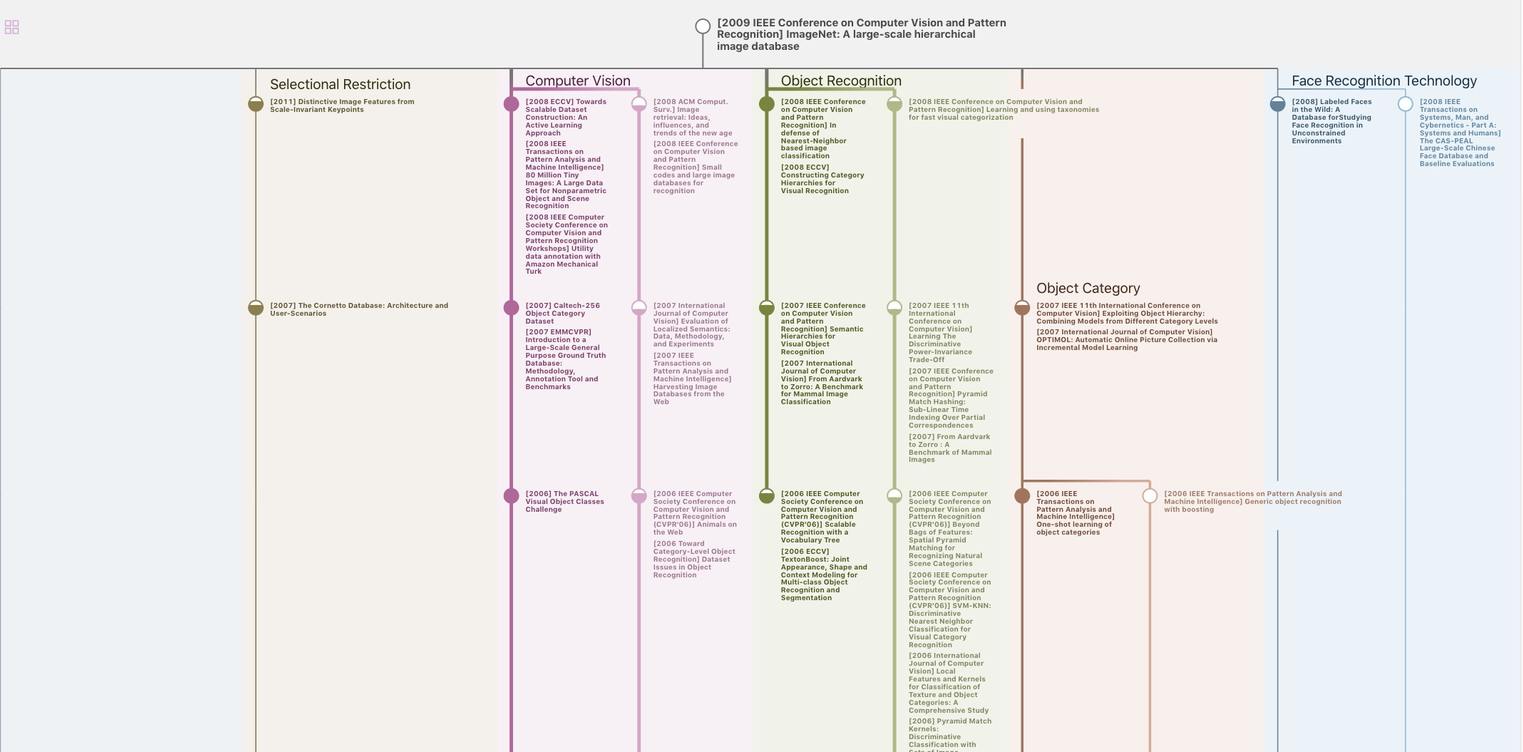
生成溯源树,研究论文发展脉络
Chat Paper
正在生成论文摘要