Comparative analysis of surface water quality prediction performance and identification of key water parameters using different machine learning models based on big data.
Water research(2019)
摘要
The water quality prediction performance of machine learning models may be not only dependent on the models, but also dependent on the parameters in data set chosen for training the learning models. Moreover, the key water parameters should also be identified by the learning models, in order to further reduce prediction costs and improve prediction efficiency. Here we endeavored for the first time to compare the water quality prediction performance of 10 learning models (7 traditional and 3 ensemble models) using big data (33,612 observations) from the major rivers and lakes in China from 2012 to 2018, based on the precision, recall, F1-score, weighted F1-score, and explore the potential key water parameters for future model prediction. Our results showed that the bigger data could improve the performance of learning models in prediction of water quality. Compared to other 7 models, decision tree (DT), random forest (RF) and deep cascade forest (DCF) trained by data sets of pH, DO, CODMn, and NH3-N had significantly better performance in prediction of all 6 Levels of water quality recommended by Chinese government. Moreover, two key water parameter sets (DO, CODMn, and NH3-N; CODMn, and NH3-N) were identified and validated by DT, RF and DCF to be high specificities for perdition water quality. Therefore, DT, RF and DCF with selected key water parameters could be prioritized for future water quality monitoring and providing timely water quality warning.
更多查看译文
AI 理解论文
溯源树
样例
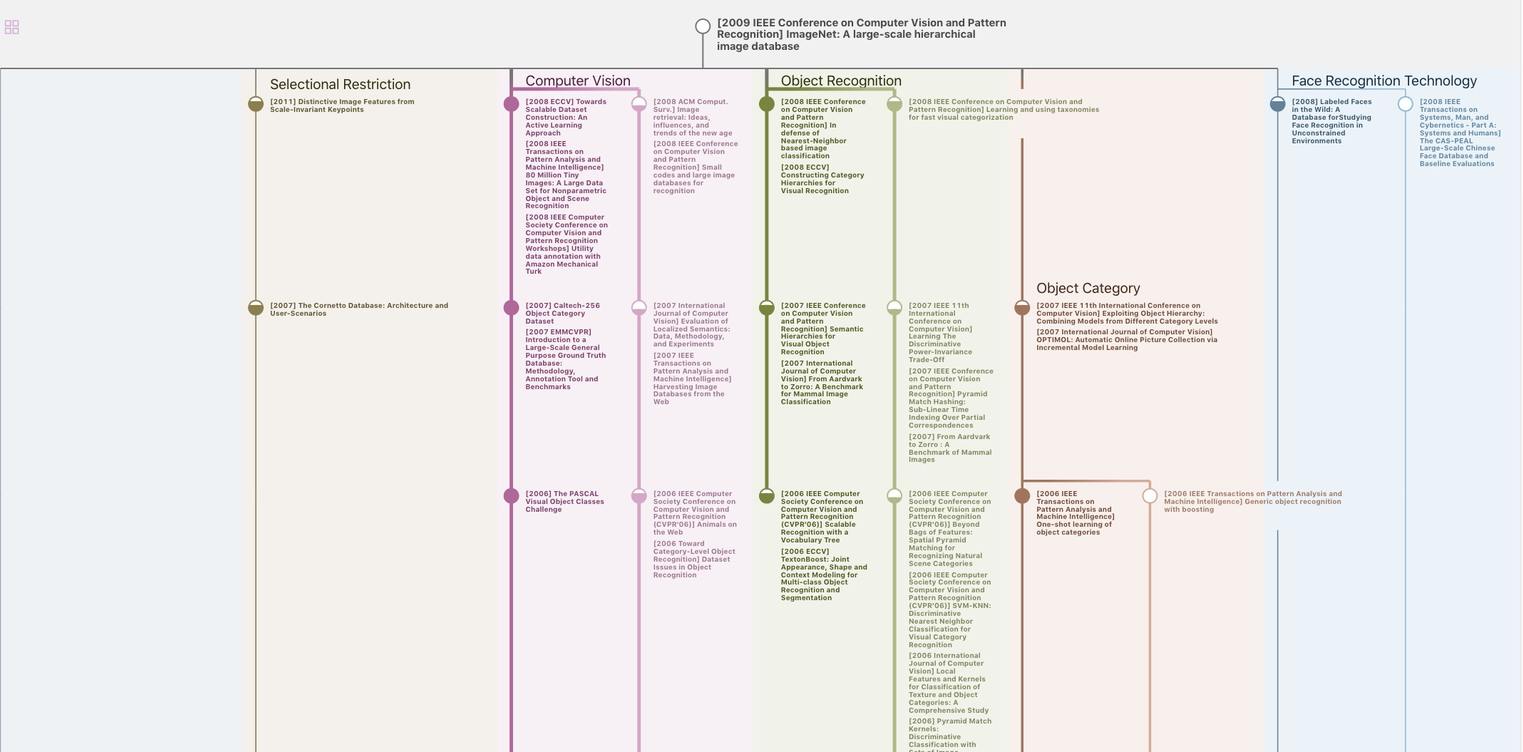
生成溯源树,研究论文发展脉络
Chat Paper
正在生成论文摘要