Adapted Random Survival Forest For Histograms To Analyze Nox Sensor Failure In Heavy Trucks
MACHINE LEARNING, OPTIMIZATION, AND DATA SCIENCE(2019)
摘要
In heavy duty trucks operation, important components need to be examined regularly so that any unexpected breakdowns can be prevented. Data-driven failure prediction models can be built using operational data from a large fleet of trucks. Machine learning methods such as Random Survival Forest (RSF) can be used to generate a survival model that can predict the survival probabilities of a particular component over time. Operational data from the trucks usually have many feature variables represented as histograms. Although bins of a histogram can be considered as an independent numeric variable, dependencies among the bins might exist that could be useful and neglected when bins are treated individually. Therefore, in this article, we propose extension to the standard RSF algorithm that can handle histogram variables and use it to train survival models for a NOx sensor. The trained model is compared in terms of overall error rate with the standard RSF model where bins of a histogram are treated individually as numeric features. The experiment results shows that the adapted approach outperforms the standard approach and the feature variables considered important are ranked.
更多查看译文
关键词
Histogram survival forest, Histogram features, NOx sensor failure
AI 理解论文
溯源树
样例
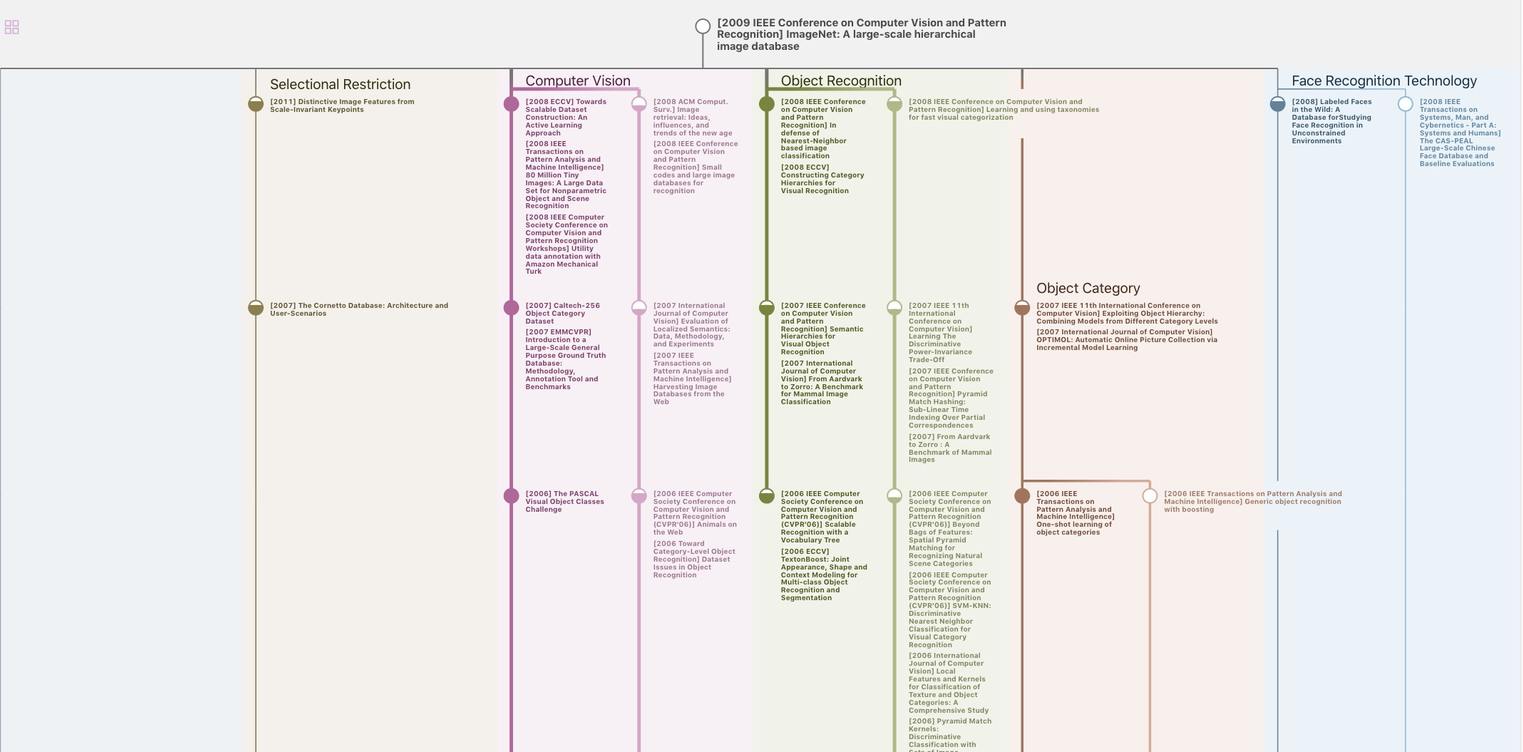
生成溯源树,研究论文发展脉络
Chat Paper
正在生成论文摘要