Deep Image Translation With an Affinity-Based Change Prior for Unsupervised Multimodal Change Detection
IEEE TRANSACTIONS ON GEOSCIENCE AND REMOTE SENSING(2022)
摘要
Image translation with convolutional neural networks has recently been used as an approach to multimodal change detection. Existing approaches train the networks by exploiting supervised information of the change areas, which, however, is not always available. A main challenge in the unsupervised problem setting is to avoid that change pixels affect the learning of the translation function. We propose two new network architectures trained with loss functions weighted by priors that reduce the impact of change pixels on the learning objective. The change prior is derived in an unsupervised fashion from relational pixel information captured by domain-specific affinity matrices. Specifically, we use the vertex degrees associated with an absolute affinity difference matrix and demonstrate their utility in combination with cycle consistency and adversarial training. The proposed neural networks are compared with the state-of-the-art algorithms. Experiments conducted on three real data sets show the effectiveness of our methodology.
更多查看译文
关键词
Feature extraction, Deep learning, Synthetic aperture radar, Spatial resolution, Satellites, Remote sensing, Optical imaging, Adversarial networks, affinity matrix, deep learning, heterogeneous data, image regression, multimodal image analysis, unsupervised change detection (CD)
AI 理解论文
溯源树
样例
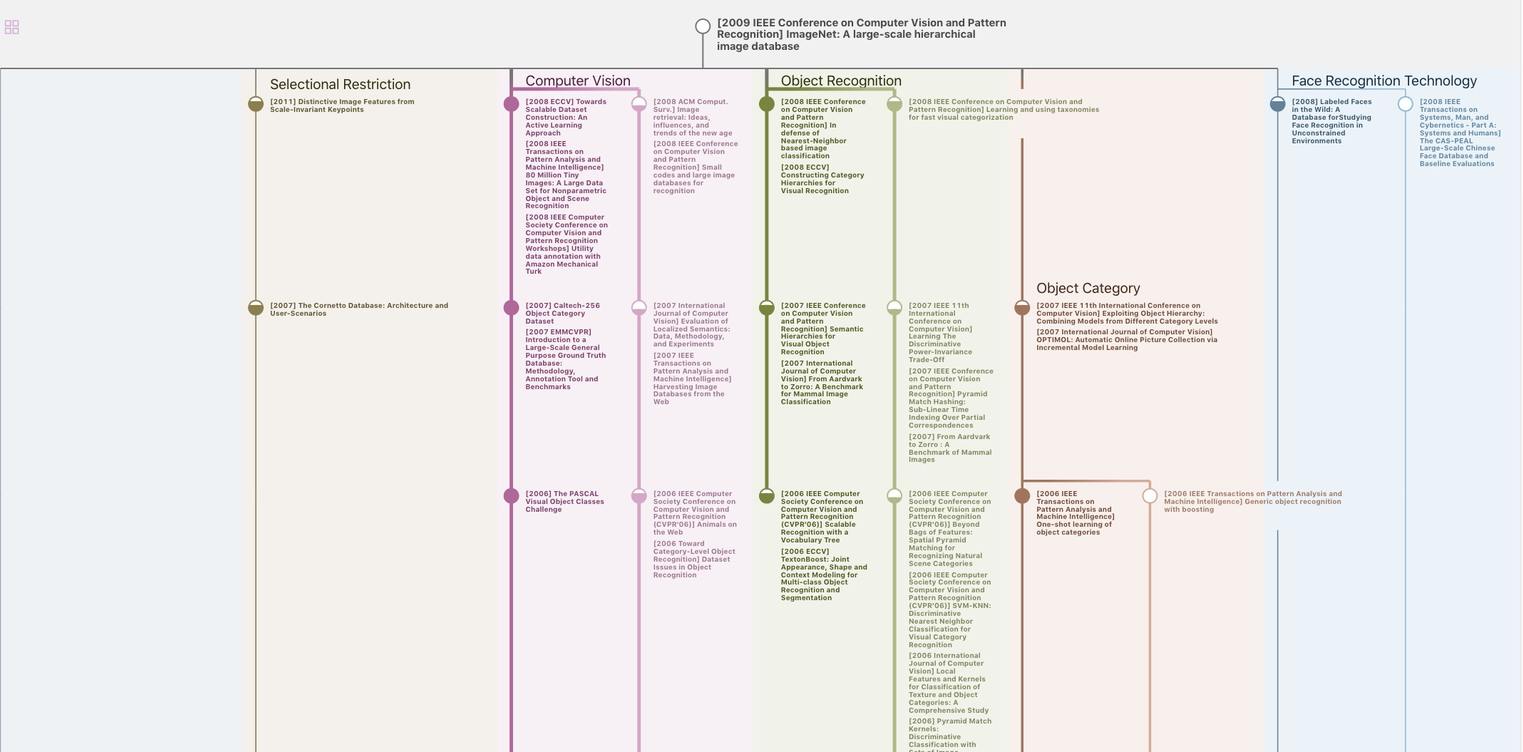
生成溯源树,研究论文发展脉络
Chat Paper
正在生成论文摘要