Machine Learning in Materials Modeling -- Fundamentals and the Opportunities in 2D Materials
arxiv(2020)
摘要
The application of machine learning in materials presents a unique challenge of dealing with scarce and varied materials data - both experimental and theoretical. Nevertheless, several state-of-the-art machine learning models for materials have been successfully developed to predict material properties for various applications such as materials for photovoltaic cells, thermoelectric materials, dielectrics, materials for batteries, fuel cells, etc. The setup of comprehensive materials databases, and openly accessible algorithm frameworks have also spurred the usage of machine learning for solving some of the most pressing problems in materials science. Some such recent implementations are discussed in this book chapter. A multitude of two-dimensional (2D) materials exist with the potential to replace the conventional materials for energy storage and nanodevices. The challenges faced in designing batteries and how machine learning tools can help in screening and narrowing down on the best composition, as well as the synthesis of air-stable 2D materials, are also discussed.
更多查看译文
关键词
materials modeling—fundamentals,machine learning
AI 理解论文
溯源树
样例
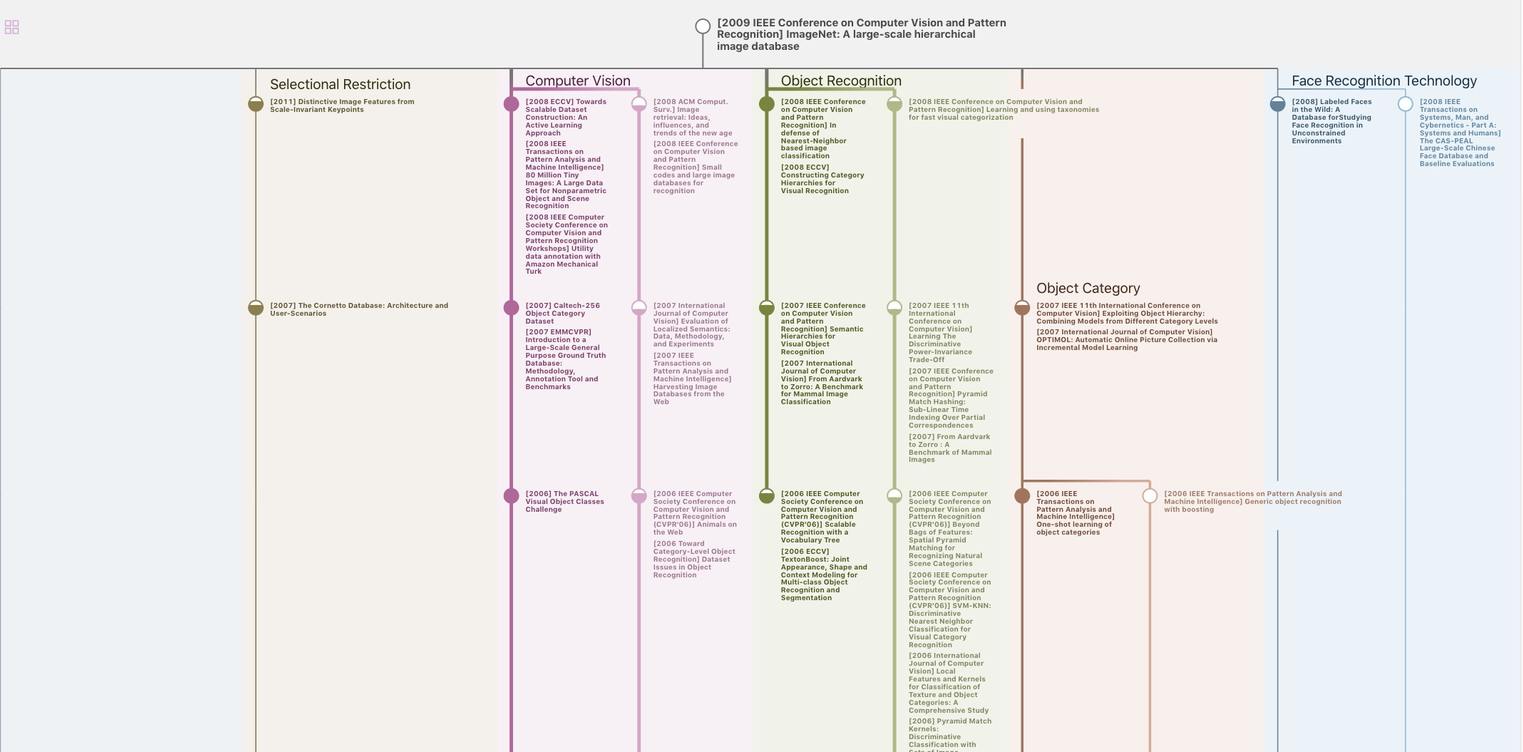
生成溯源树,研究论文发展脉络
Chat Paper
正在生成论文摘要