Analysis of Bayesian Inference Algorithms by the Dynamical Functional Approach
JOURNAL OF PHYSICS A-MATHEMATICAL AND THEORETICAL(2020)
Abstract
We analyze the dynamics of an algorithm for approximate inference with large Gaussian latent variable models in a student-teacher scenario. To model nontrivial dependencies between the latent variables, we assume random covariance matrices drawn from rotation invariant ensembles. For the case of perfect data-model matching, the knowledge of static order parameters derived from the replica method allows us to obtain efficient algorithmic updates in terms of matrix-vector multiplications with a fixed matrix. Using the dynamical functional approach, we obtain an exact effective stochastic process in the thermodynamic limit for a single node. From this, we obtain closed-form expressions for the rate of the convergence. Analytical results are in excellent agreement with simulations of single instances of large models.
MoreTranslated text
Key words
Bayesian inference,iterative algorithms,TAP equations,random matrices,dynamical functional theory
AI Read Science
Must-Reading Tree
Example
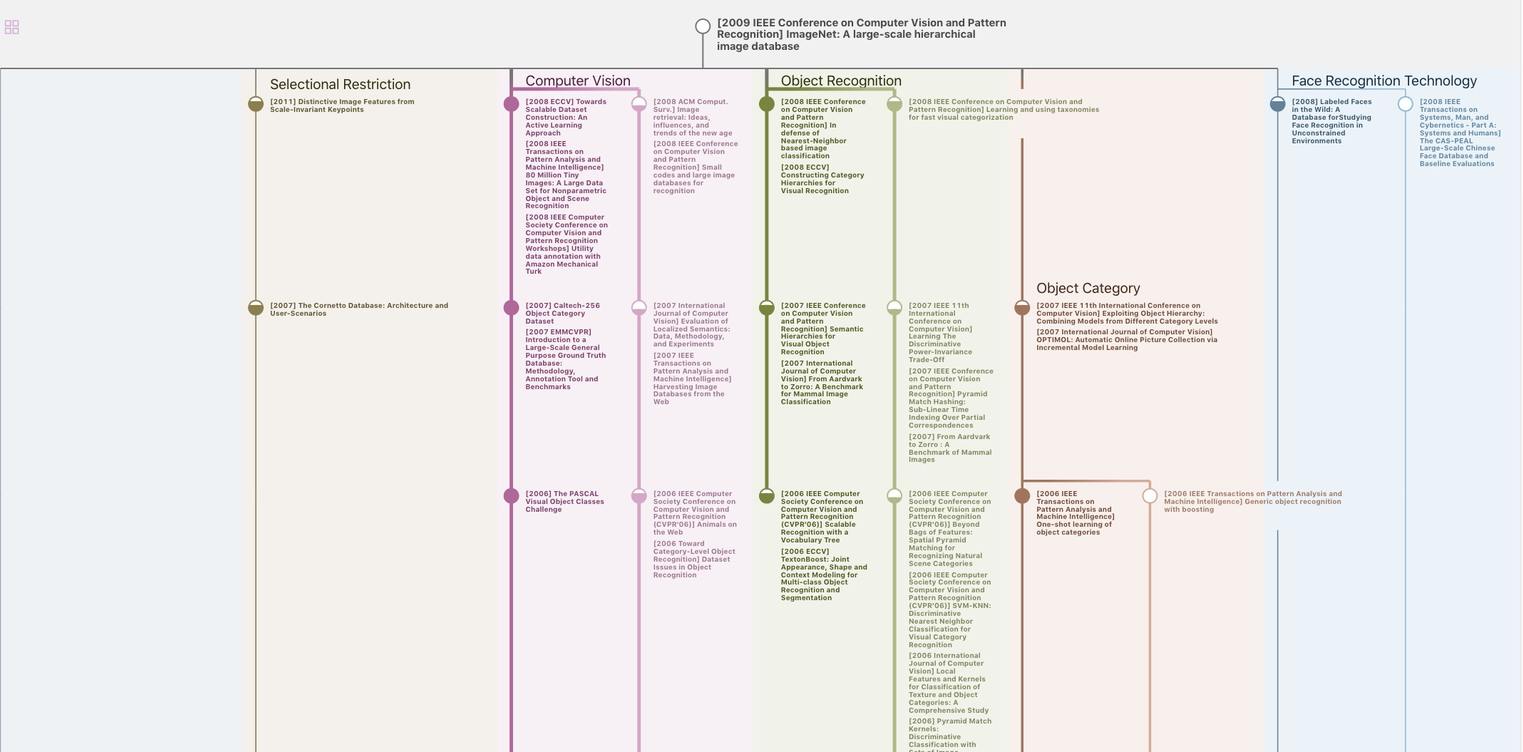
Generate MRT to find the research sequence of this paper
Chat Paper
Summary is being generated by the instructions you defined