Language Models Are An Effective Patient Representation Learning Technique For Electronic Health Record Data
arxiv(2020)
摘要
Widespread adoption of electronic health records (EHRs) has fueled development of clinical outcome models using machine learning. However, patient EHR data are complex, and how to optimally represent them is an open question. This complexity, along with often small training set sizes available to train these clinical outcome models, are two core challenges for training high quality models. In this paper, we demonstrate that learning generic representations from the data of all the patients in the EHR enables better performing prediction models for clinical outcomes, allowing for these challenges to be overcome. We adapt common representation learning techniques used in other domains and find that representations inspired by language models enable a 3.5% mean improvement in AUROC on five clinical outcomes compared to standard baselines, with the average improvement rising to 19% when only a small number of patients are available for training a prediction model for a given clinical outcome.
更多查看译文
关键词
Electronic health record,Representation learning,Transfer learning,Risk stratification,Machine learning
AI 理解论文
溯源树
样例
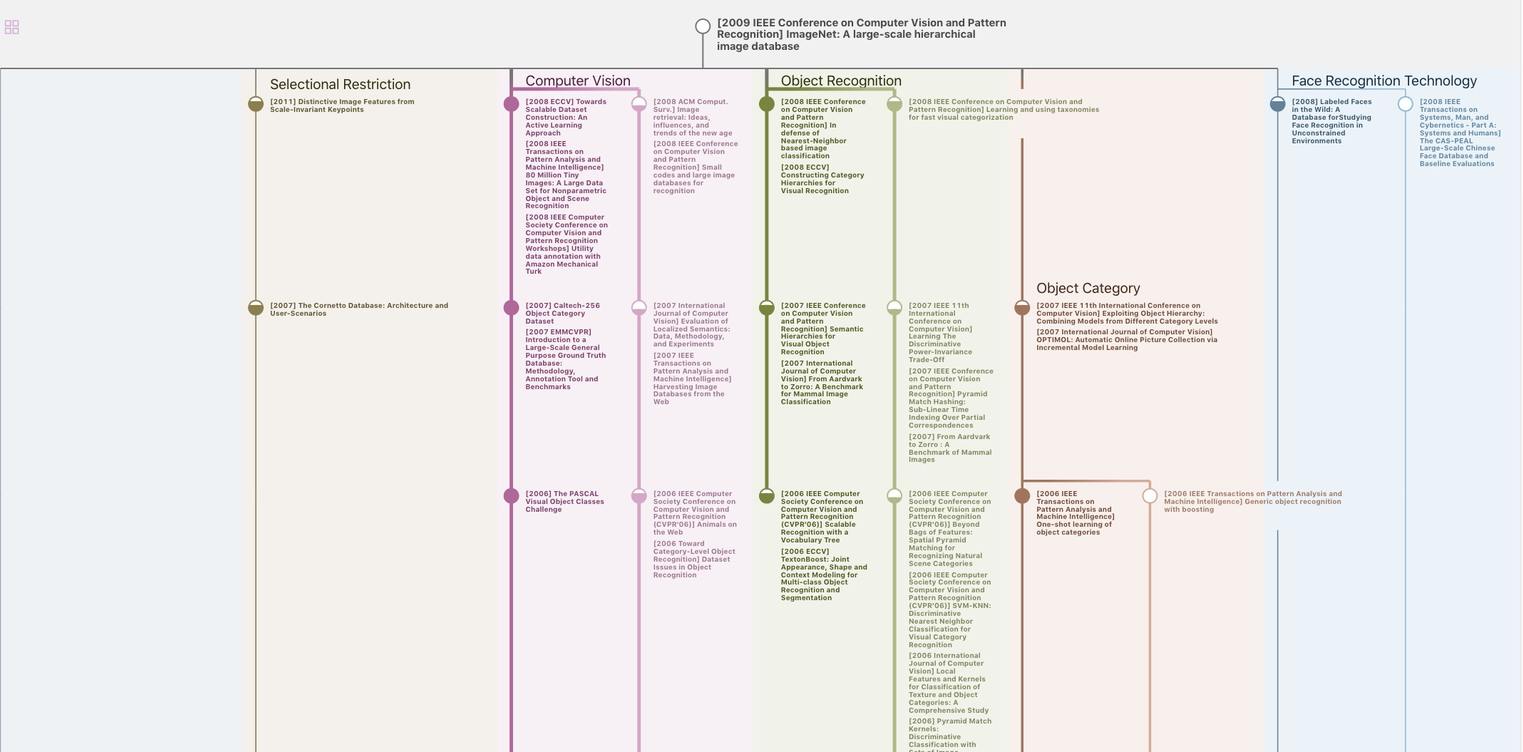
生成溯源树,研究论文发展脉络
Chat Paper
正在生成论文摘要