Profile least squares estimators in the monotone single index model
arxiv(2020)
摘要
We consider least squares estimators of the finite dimensional regression parameter $\alpha$ in the single index regression model $Y=\psi(\alpha^TX)+\epsilon$, where $X$ is a $d$-dimensional random vector, $E(Y|X)=\psi(\alpha^TX)$, and where $\psi$ is monotone. It has been suggested to estimate $\alpha$ by a profile least squares estimator, minimizing $\sum_{i=1}^n(Y_i-\psi(\alpha^TX_i))^2$ over monotone $\psi$ and $\alpha$ on the boundary $S_{d-1}$of the unit ball. Although this suggestion has been around for a long time, it is still unknown whether the estimate is $\sqrt{n}$ convergent. We show that a profile least squares estimator, using the same pointwise least squares estimator for fixed $\alpha$, but using a different global sum of squares, is $\sqrt{n}$-convergent and asymptotically normal. The difference between the corresponding loss functions is studied and also a comparison with other methods is given. An augmented Lagrange method, embedded in the Hooke-Jeeves pattern search algorithm, is implemented in R to compute the profile least squares estimators.
更多查看译文
关键词
estimators,index,profile,model
AI 理解论文
溯源树
样例
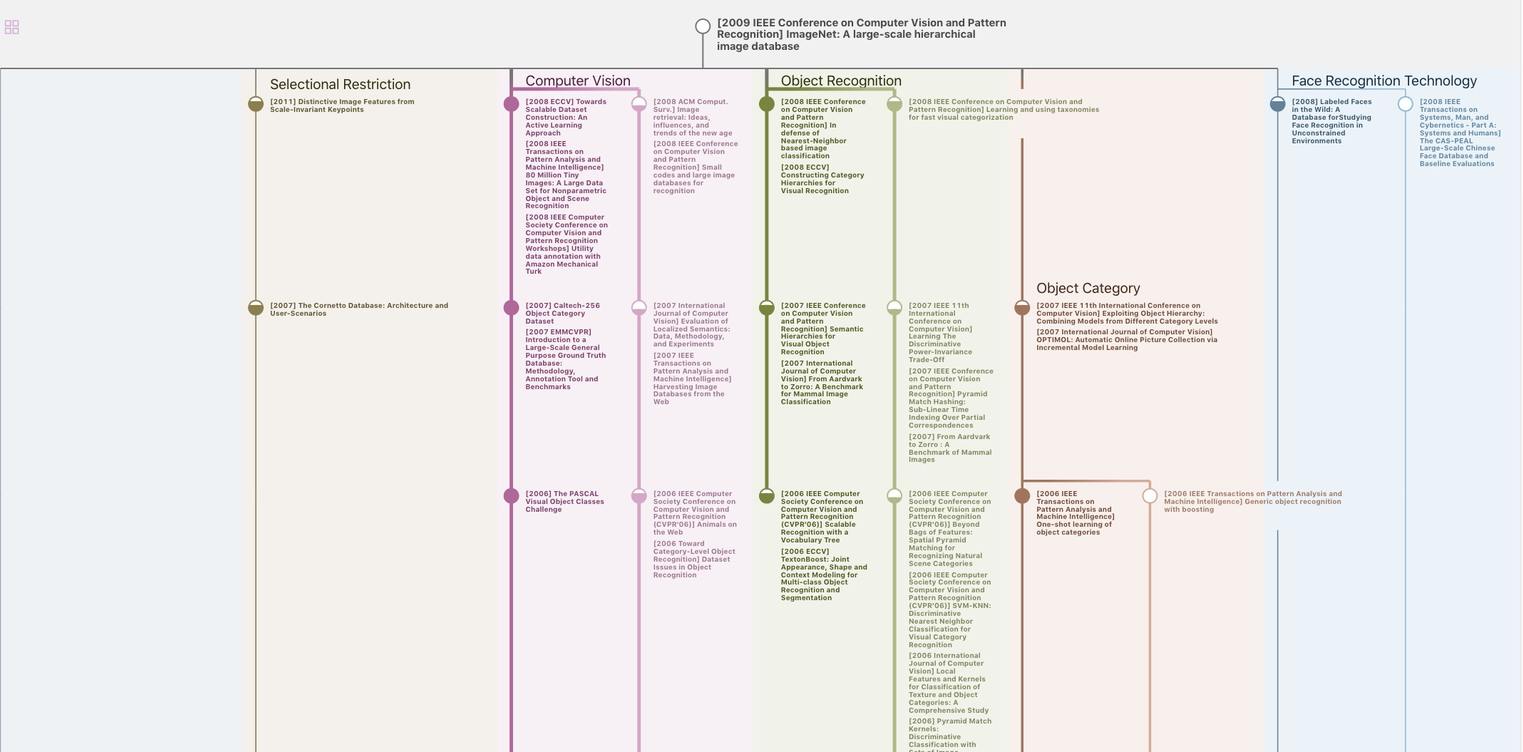
生成溯源树,研究论文发展脉络
Chat Paper
正在生成论文摘要