MIME: Mutual Information Minimisation Exploration
CoRR(2020)
摘要
We show that reinforcement learning agents that learn by surprise (surprisal) get stuck at abrupt environmental transition boundaries because these transitions are difficult to learn. We propose a counter-intuitive solution that we call Mutual Information Minimising Exploration (MIME) where an agent learns a latent representation of the environment without trying to predict the future states. We show that our agent performs significantly better over sharp transition boundaries while matching the performance of surprisal driven agents elsewhere. In particular, we show state-of-the-art performance on difficult learning games such as Gravitar, Montezuma's Revenge and Doom.
更多查看译文
关键词
mutual information minimisation exploration
AI 理解论文
溯源树
样例
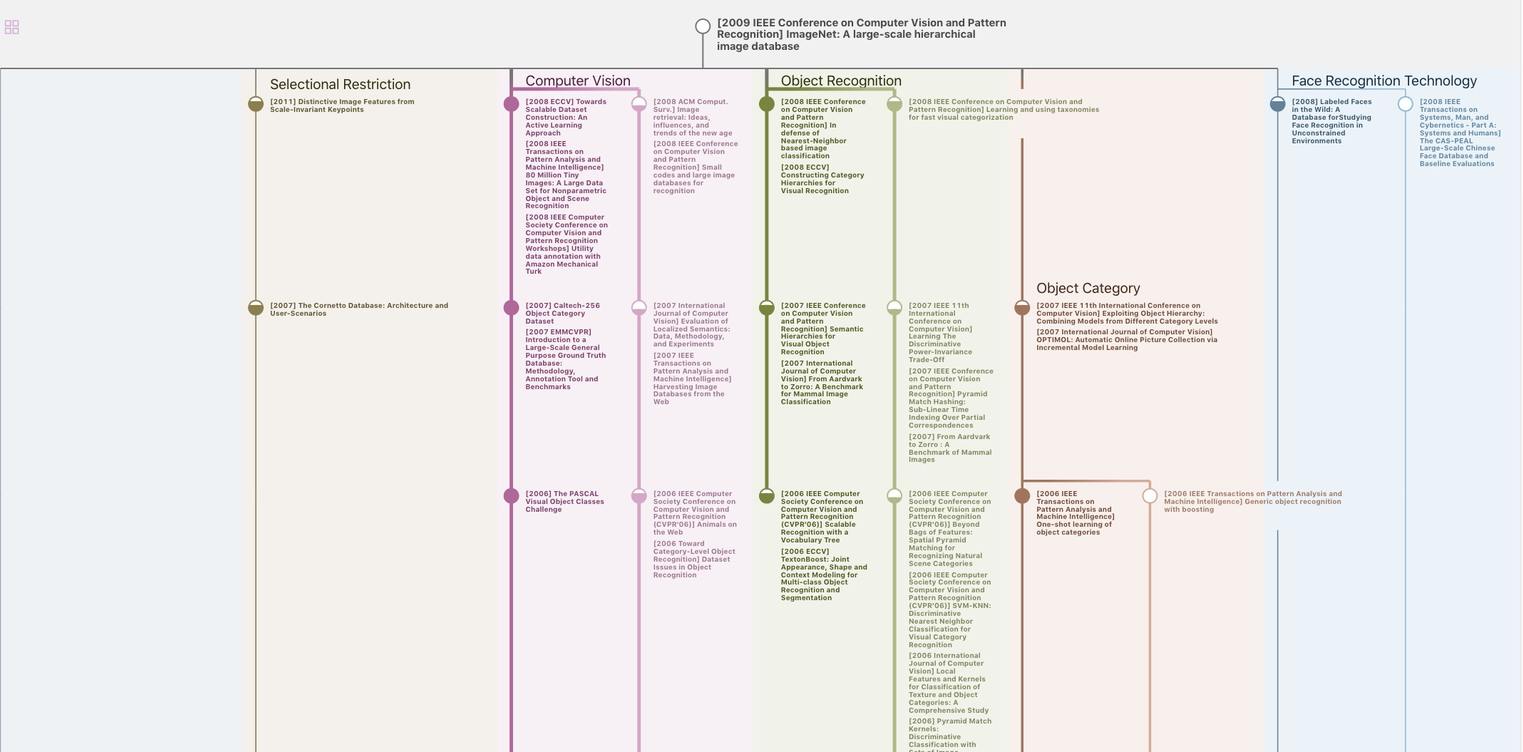
生成溯源树,研究论文发展脉络
Chat Paper
正在生成论文摘要