A Better Bound Gives a Hundred Rounds: Enhanced Privacy Guarantees via $f$-Divergences
arxiv(2020)
摘要
We derive the optimal differential privacy (DP) parameters of a mechanism that satisfies a given level of R\'enyi differential privacy (RDP). Our result is based on the joint range of two $f$-divergences that underlie the approximate and the R\'enyi variations of differential privacy. We apply our result to the moments accountant framework for characterizing privacy guarantees of stochastic gradient descent. When compared to the state-of-the-art, our bounds may lead to about 100 more stochastic gradient descent iterations for training deep learning models for the same privacy budget.
更多查看译文
关键词
f-divergences,Renyi variations,stochastic gradient descent,privacy budget,enhanced privacy guarantees,optimal differential privacy parameters,Renyí differential privacy,joint range,moments accountant framework,deep learning model training
AI 理解论文
溯源树
样例
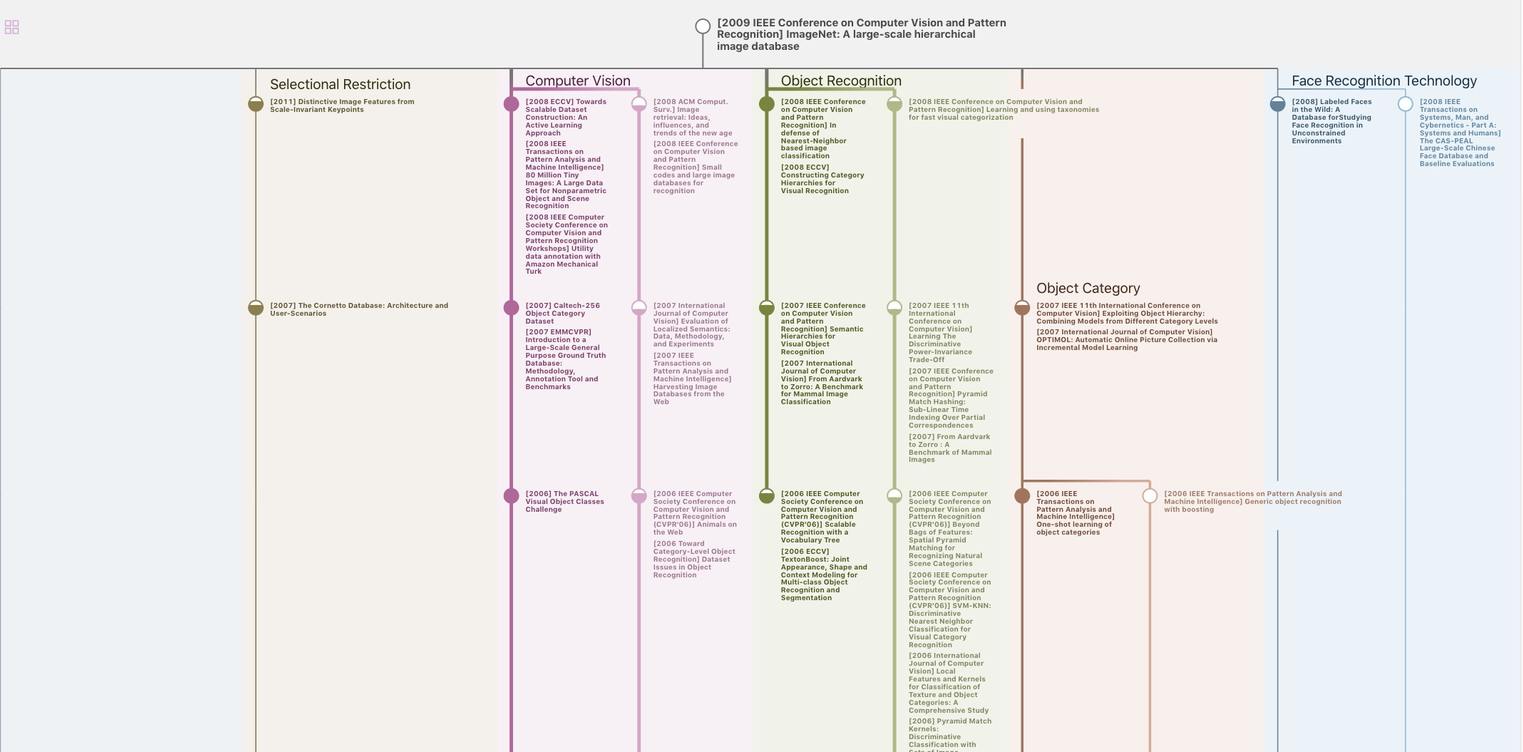
生成溯源树,研究论文发展脉络
Chat Paper
正在生成论文摘要