Association of Machine Learning-Derived Phenogroupings of Echocardiographic Variables with Heart Failure in Stable Coronary Artery Disease: The Heart and Soul Study.
Journal of the American Society of Echocardiography : official publication of the American Society of Echocardiography(2020)
摘要
BACKGROUND:Many individual echocardiographic variables have been associated with heart failure (HF) in patients with stable coronary artery disease (CAD), but their combined utility for prediction has not been well studied.
METHODS:Unsupervised model-based cluster analysis was performed by researchers blinded to the study outcome in 1,000 patients with stable CAD on 15 transthoracic echocardiographic variables. We evaluated associations of cluster membership with HF hospitalization using Cox proportional hazards regression analysis.
RESULTS:The echo-derived clusters partitioned subjects into four phenogroupings: phenogroup 1 (n = 85) had the highest levels, phenogroups 2 (n = 314) and 3 (n = 205) displayed intermediate levels, and phenogroup 4 (n = 396) had the lowest levels of cardiopulmonary structural and functional abnormalities. Over 7.1 ± 3.2 years of follow-up, there were 198 HF hospitalizations. After multivariable adjustment for traditional cardiovascular risk factors, phenogroup 1 was associated with a nearly fivefold increased risk (hazard ratio [HR] = 4.8; 95% CI, 2.4-9.5), phenogroup 2 was associated with a nearly threefold increased risk (HR = 2.7; 95% CI, 1.4-5.0), and phenogroup 3 was associated with a nearly twofold increased risk (HR = 1.9; 95% CI, 1.0-3.8) of HF hospitalization, relative to phenogroup 4.
CONCLUSIONS:Transthoracic echocardiographic variables can be used to classify stable CAD patients into separate phenogroupings that differentiate cardiopulmonary structural and functional abnormalities and can predict HF hospitalization, independent of traditional cardiovascular risk factors.
更多查看译文
AI 理解论文
溯源树
样例
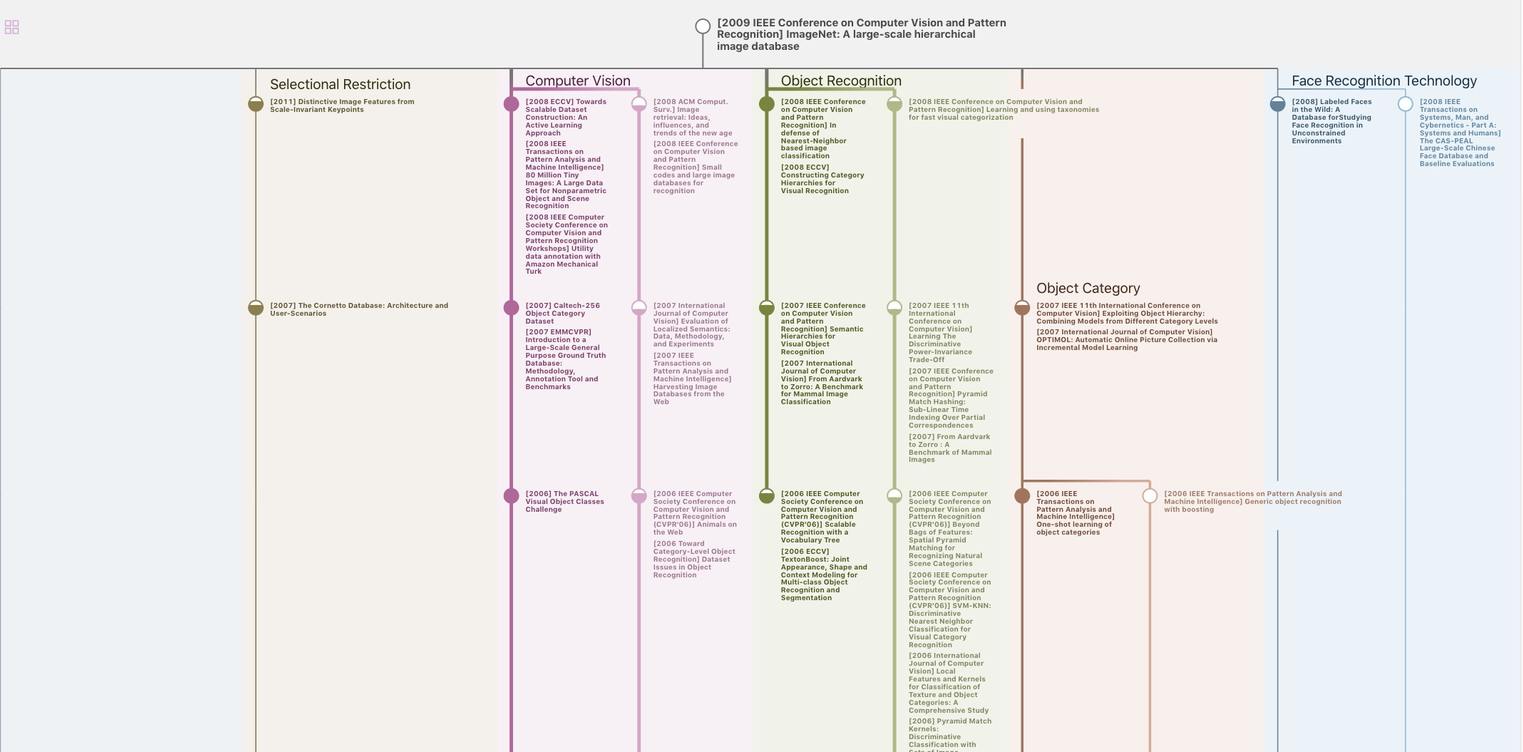
生成溯源树,研究论文发展脉络
Chat Paper
正在生成论文摘要