Classification and prediction of diabetes disease using machine learning paradigm
Health Information Science and Systems(2020)
摘要
Background and objectives Diabetes is a chronic disease characterized by high blood sugar. It may cause many complicated disease like stroke, kidney failure, heart attack, etc. About 422 million people were affected by diabetes disease in worldwide in 2014. The figure will be reached 642 million in 2040. The main objective of this study is to develop a machine learning (ML)-based system for predicting diabetic patients. Materials and methods Logistic regression (LR) is used to identify the risk factors for diabetes disease based on p value and odds ratio (OR). We have adopted four classifiers like naïve Bayes (NB), decision tree (DT), Adaboost (AB), and random forest (RF) to predict the diabetic patients. Three types of partition protocols (K2, K5, and K10) have also adopted and repeated these protocols into 20 trails. Performances of these classifiers are evaluated using accuracy (ACC) and area under the curve (AUC). Results We have used diabetes dataset, conducted in 2009–2012, derived from the National Health and Nutrition Examination Survey. The dataset consists of 6561 respondents with 657 diabetic and 5904 controls. LR model demonstrates that 7 factors out of 14 as age, education, BMI, systolic BP, diastolic BP, direct cholesterol, and total cholesterol are the risk factors for diabetes. The overall ACC of ML-based system is 90.62% . The combination of LR-based feature selection and RF-based classifier gives 94.25% ACC and 0.95 AUC for K10 protocol. Conclusion The combination of LR and RF-based classifier performs better. This combination will be very helpful for predicting diabetic patients.
更多查看译文
关键词
Diabetes, Classification, Machine learning, Naïve Bayes, Decision tree, Random forest, Adaboost
AI 理解论文
溯源树
样例
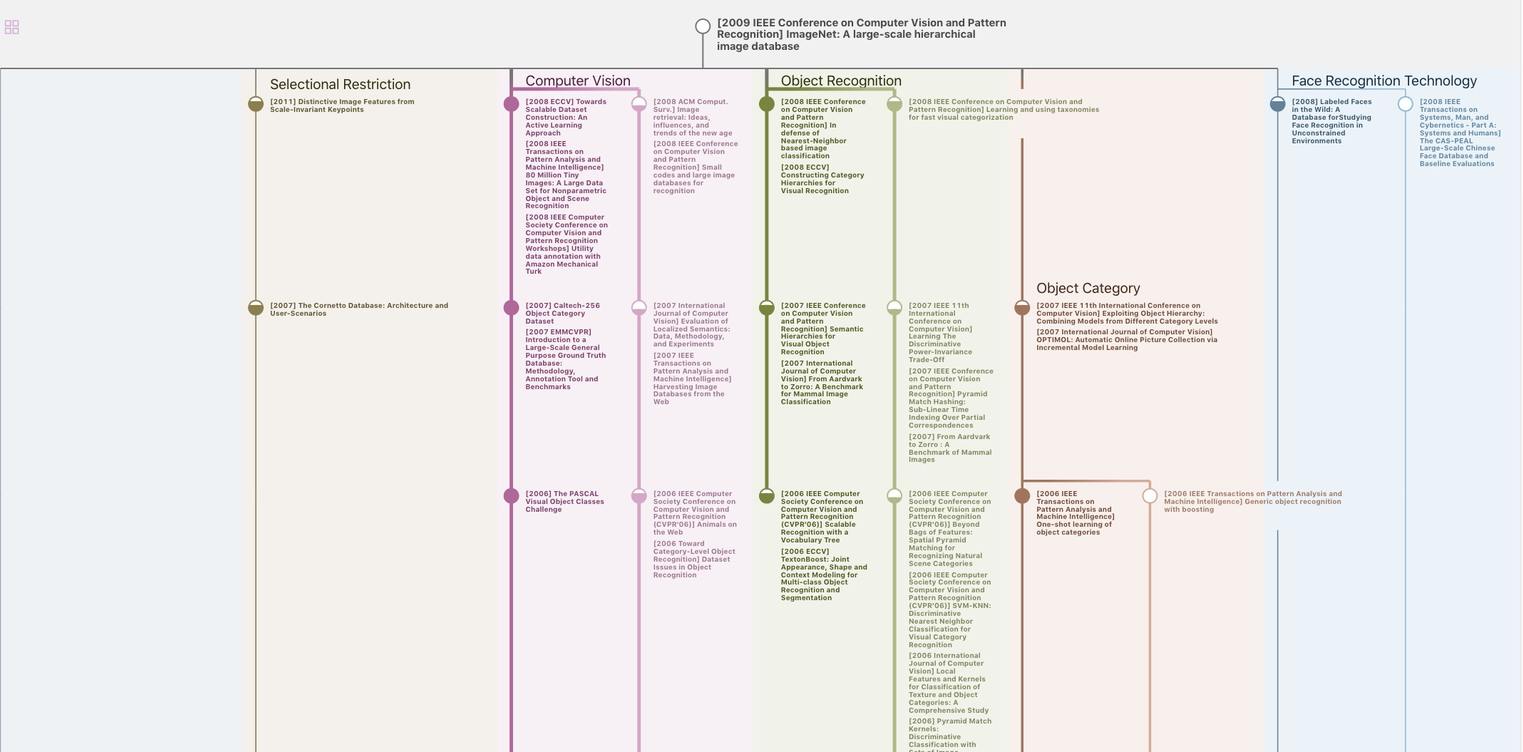
生成溯源树,研究论文发展脉络
Chat Paper
正在生成论文摘要