Privacy Amplification of Iterative Algorithms via Contraction Coefficients
ISIT(2020)
摘要
We investigate the framework of privacy amplification by iteration, recently proposed by Feldman et al., from an information-theoretic lens. We demonstrate that differential privacy guarantees of iterative mappings can be determined by a direct application of contraction coefficients derived from strong data processing inequalities for f-divergences. In particular, by generalizing the Dobrushin’s contraction coefficient for total variation distance to an f-divergence known as E γ -divergence, we derive tighter bounds on the differential privacy parameters of the projected noisy stochastic gradient descent algorithm with hidden intermediate updates.
更多查看译文
关键词
privacy amplification,information-theoretic lens,differential privacy guarantees,iterative mappings,data processing inequalities,f-divergence,Dobrushin contraction coefficient,total variation distance,differential privacy parameters,stochastic gradient descent algorithm,iterative algorithms,Eγ-divergence
AI 理解论文
溯源树
样例
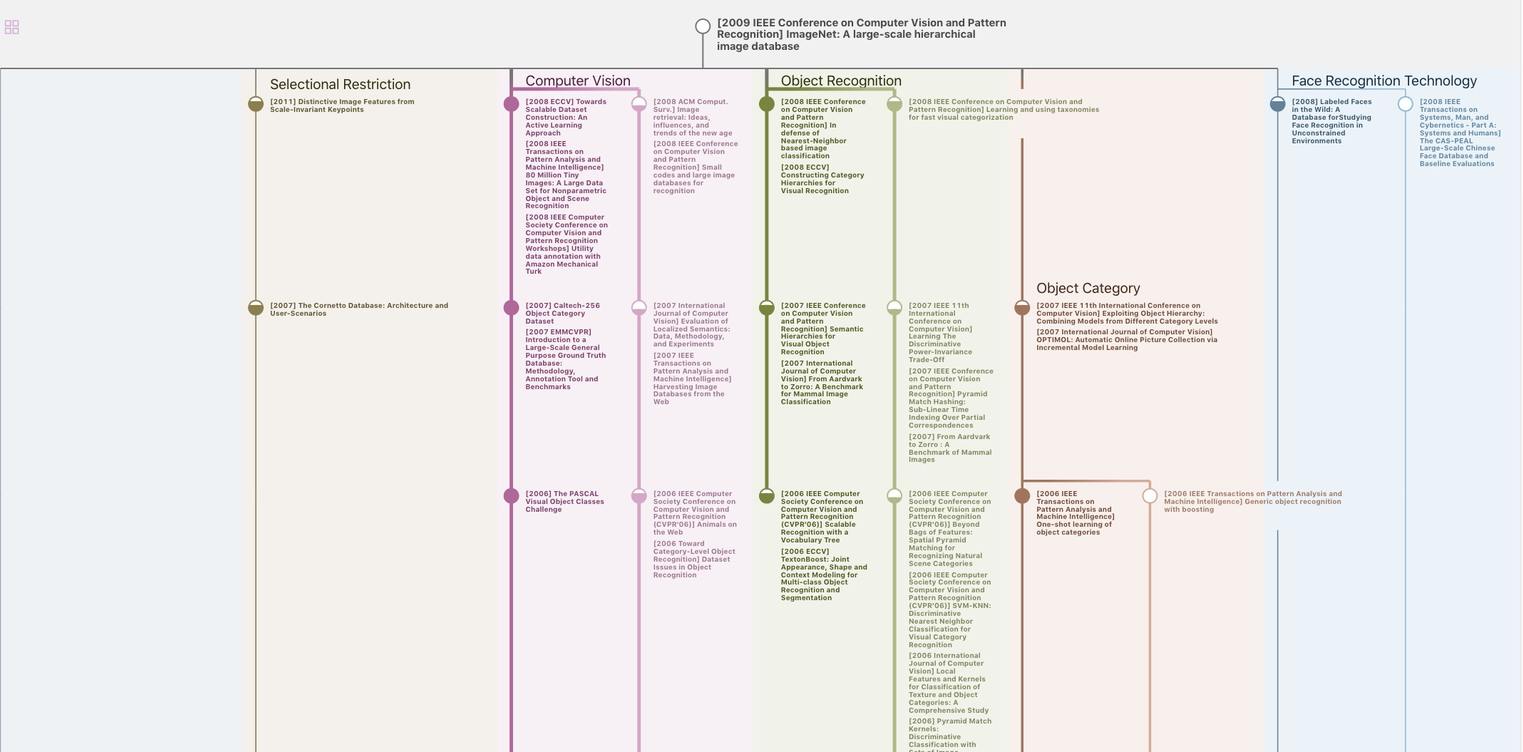
生成溯源树,研究论文发展脉络
Chat Paper
正在生成论文摘要