R2DE - a NLP approach to estimating IRT parameters of newly generated questions.
LAK20: THE TENTH INTERNATIONAL CONFERENCE ON LEARNING ANALYTICS & KNOWLEDGE(2020)
摘要
The main objective of exams consists in performing an assessment of students' expertise on a specific subject. Such expertise, also referred to as skill or knowledge level, can then be leveraged in different ways (e.g., to assign a grade to the students, to understand whether a student might need some support, etc.). Similarly, the questions appearing in the exams have to be assessed in some way before being used to evaluate students. Standard approaches to questions' assessment are either subjective (e.g., assessment by human experts) or introduce a long delay in the process of question generation (e.g., pretesting with real students). In this work we introduce R2DE (which is a Regressor for Difficulty and Discrimination Estimation), a model capable of assessing newly generated multiple-choice questions by looking at the text of the question and the text of the possible choices. In particular, it can estimate the difficulty and the discrimination of each question, as they are defined in Item Response Theory. We also present the results of extensive experiments we carried out on a real world large scale dataset coming from an e-learning platform, showing that our model can be used to perform an initial assessment of newly created questions and ease some of the problems that arise in question generation.
更多查看译文
关键词
learning analytics,natural language processing,knowledge tracing,item response theory,latent traits estimation,educational data mining
AI 理解论文
溯源树
样例
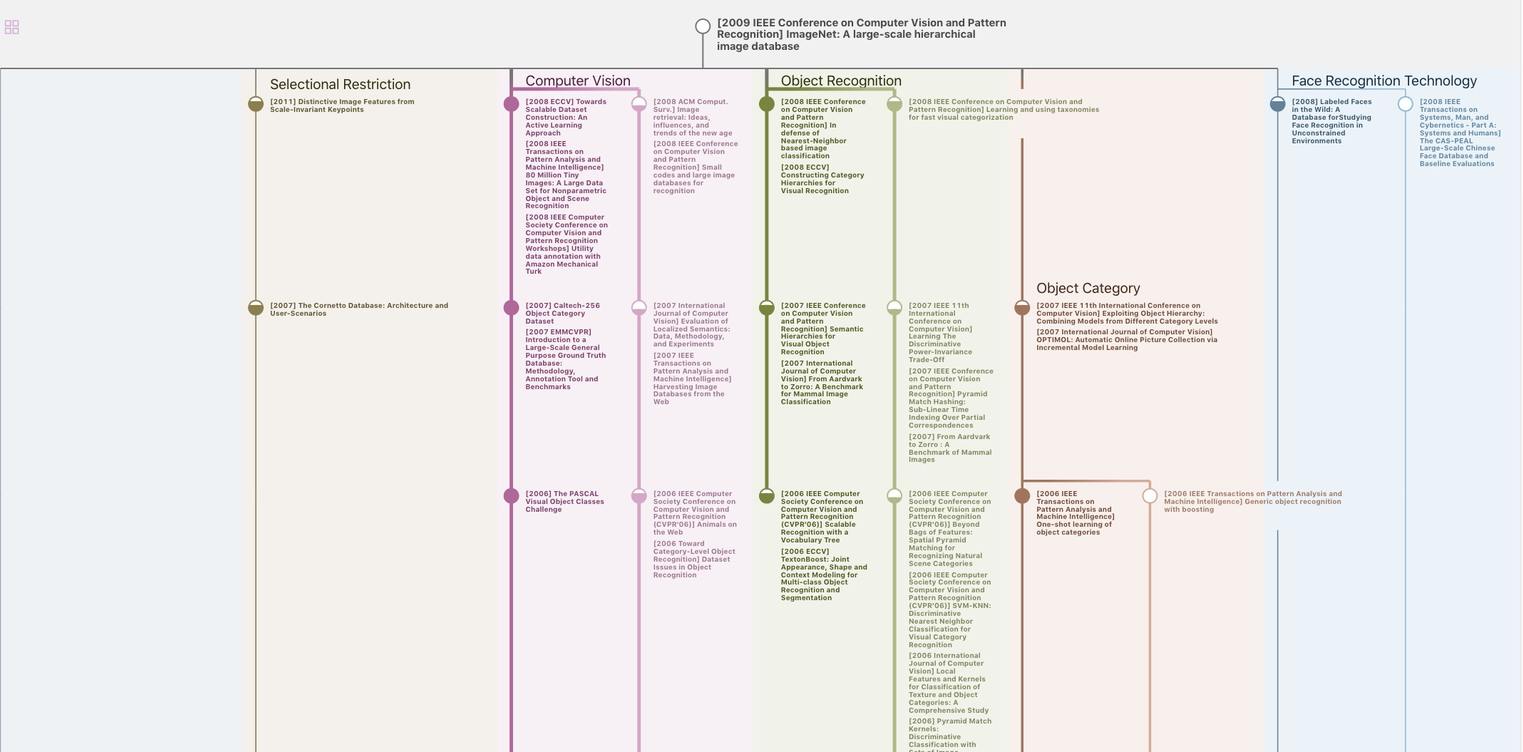
生成溯源树,研究论文发展脉络
Chat Paper
正在生成论文摘要