Generate High-Resolution Adversarial Samples by Identifying Effective Features
arxiv(2020)
摘要
As the prevalence of deep learning in computer vision, adversarial samples that weaken the neural networks emerge in large numbers, revealing their deep-rooted defects. Most adversarial attacks calculate an imperceptible perturbation in image space to fool the DNNs. In this strategy, the perturbation looks like noise and thus could be mitigated. Attacks in feature space produce semantic perturbation, but they could only deal with low resolution samples. The reason lies in the great number of coupled features to express a high-resolution image. In this paper, we propose Attack by Identifying Effective Features (AIEF), which learns different weights for features to attack. Effective features, those with great weights, influence the victim model much but distort the image little, and thus are more effective for attack. By attacking mostly on them, AIEF produces high resolution adversarial samples with acceptable distortions. We demonstrate the effectiveness of AIEF by attacking on different tasks with different generative models.
更多查看译文
关键词
effective features,high-resolution
AI 理解论文
溯源树
样例
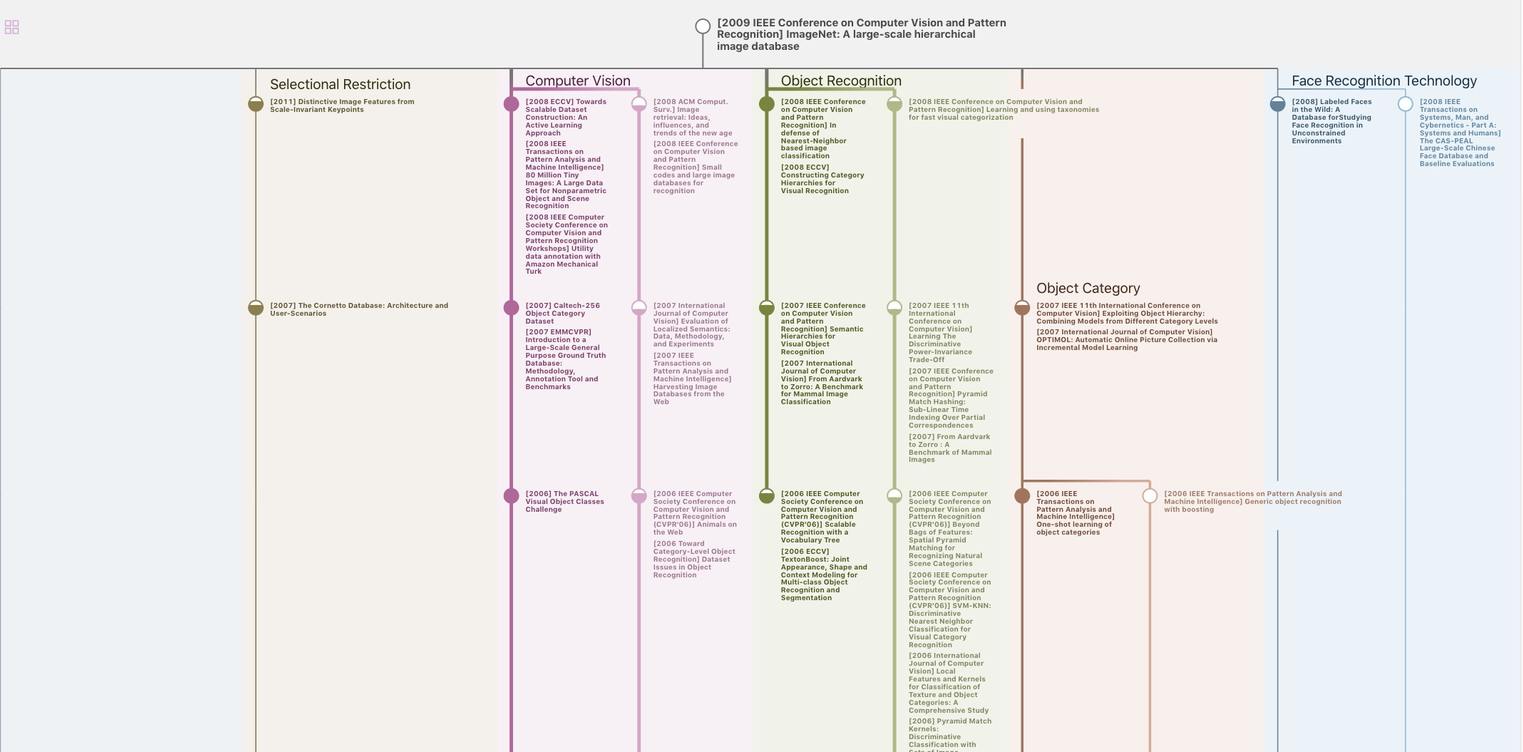
生成溯源树,研究论文发展脉络
Chat Paper
正在生成论文摘要