Incentivising Exploration and Recommendations for Contextual Bandits with Payments
EUMAS/AT(2020)
摘要
We propose a contextual bandit based model to capture the learning and social welfare goals of a web platform in the presence of myopic users. By using payments to incentivize these agents to explore different items/recommendations, we show how the platform can learn the inherent attributes of items and achieve a sublinear regret while maximizing cumulative social welfare. We also calculate theoretical bounds on the cumulative costs of incentivization to the platform. Unlike previous works in this domain, we consider contexts to be completely adversarial, and the behavior of the adversary is unknown to the platform. Our approach can improve various engagement metrics of users on e-commerce stores, recommendation engines and matching platforms.
更多查看译文
关键词
contextual bandits
AI 理解论文
溯源树
样例
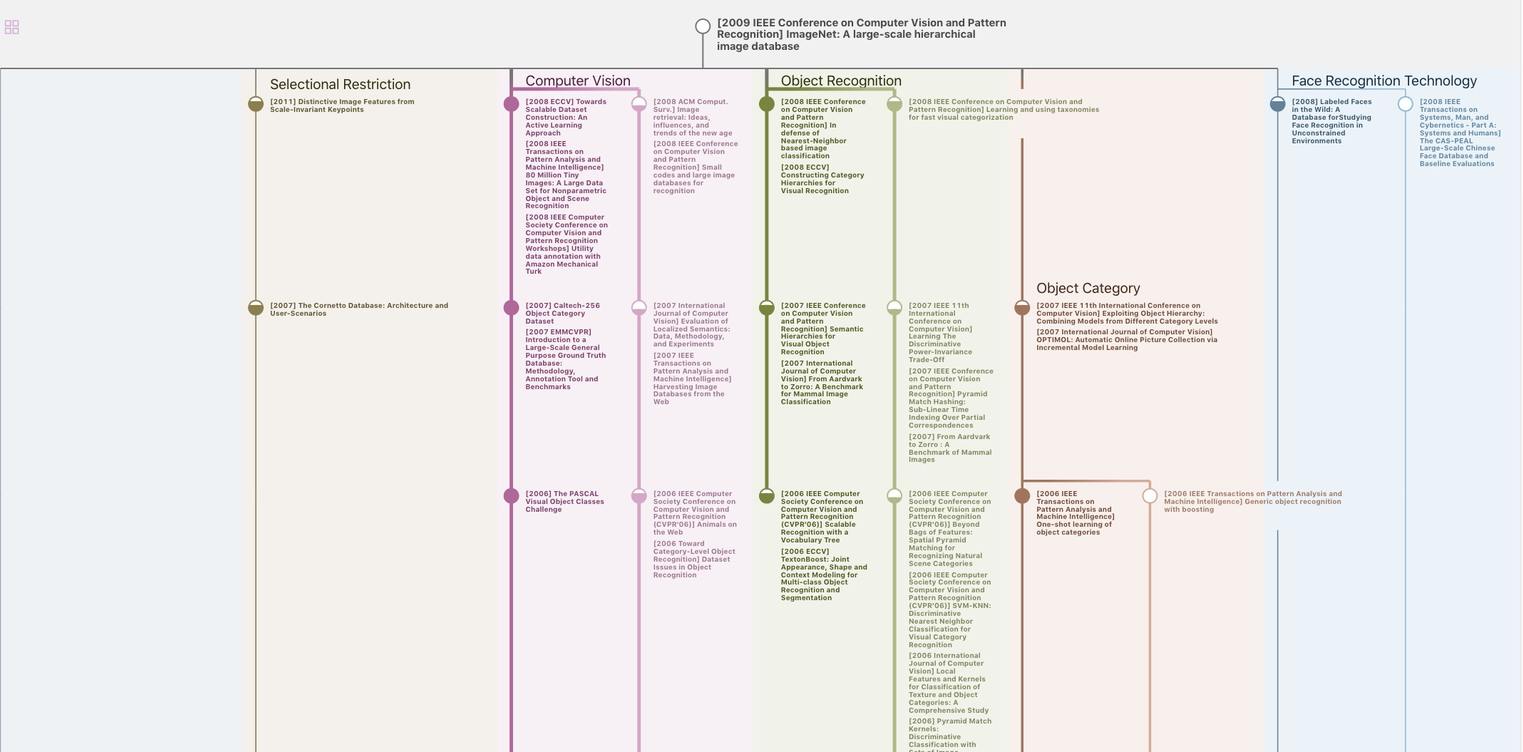
生成溯源树,研究论文发展脉络
Chat Paper
正在生成论文摘要