Imputation Methods Outperform Missing-Indicator for Data Missing Completely at Random
2019 International Conference on Data Mining Workshops (ICDMW)(2019)
摘要
Missing data is a ubiquitous cross-domain problem persistent in the context of big data analytics. Approaches to deal with missing data can be partitioned into methods that impute substitute values and methods that introduce missing-indicator variables. In this work, we demonstrate that the missing-indicator method underperforms compared to any of the other imputation methods. Most studies either focus on minimizing the squared error for the imputed values or use the missing-indicator in machine learning tasks as an assumed best practice. We study the difference between the missing-indicator method and various imputation methods on classifier learning performance when data are missing completely at random (MCAR). We compute the classifier performance over 22 complete classification datasets of varying sample size and dimensionality from an open data repository, simulating synthetic missingness at different percentages. We compare classifier performances yielded by applying mean, median, linear regression, and tree-based regression imputation methods with the corresponding performances yielded by applying the missing-indicator approach. The impact is measured with respect to three different classifiers, namely a tree-based ensemble classifier, radial basis function support vector machine classifier and k-nearest neighbours classifier. With these experiments, we conclude that given a classification problem with missing numerical data under MCAR, the missing-indicator method provides a performance decrease and should be, therefore, dismissed as a missing data-handling approach in the MCAR scenario.
更多查看译文
关键词
missing-indicator method,missing data,data preprocessing,imputation,classification
AI 理解论文
溯源树
样例
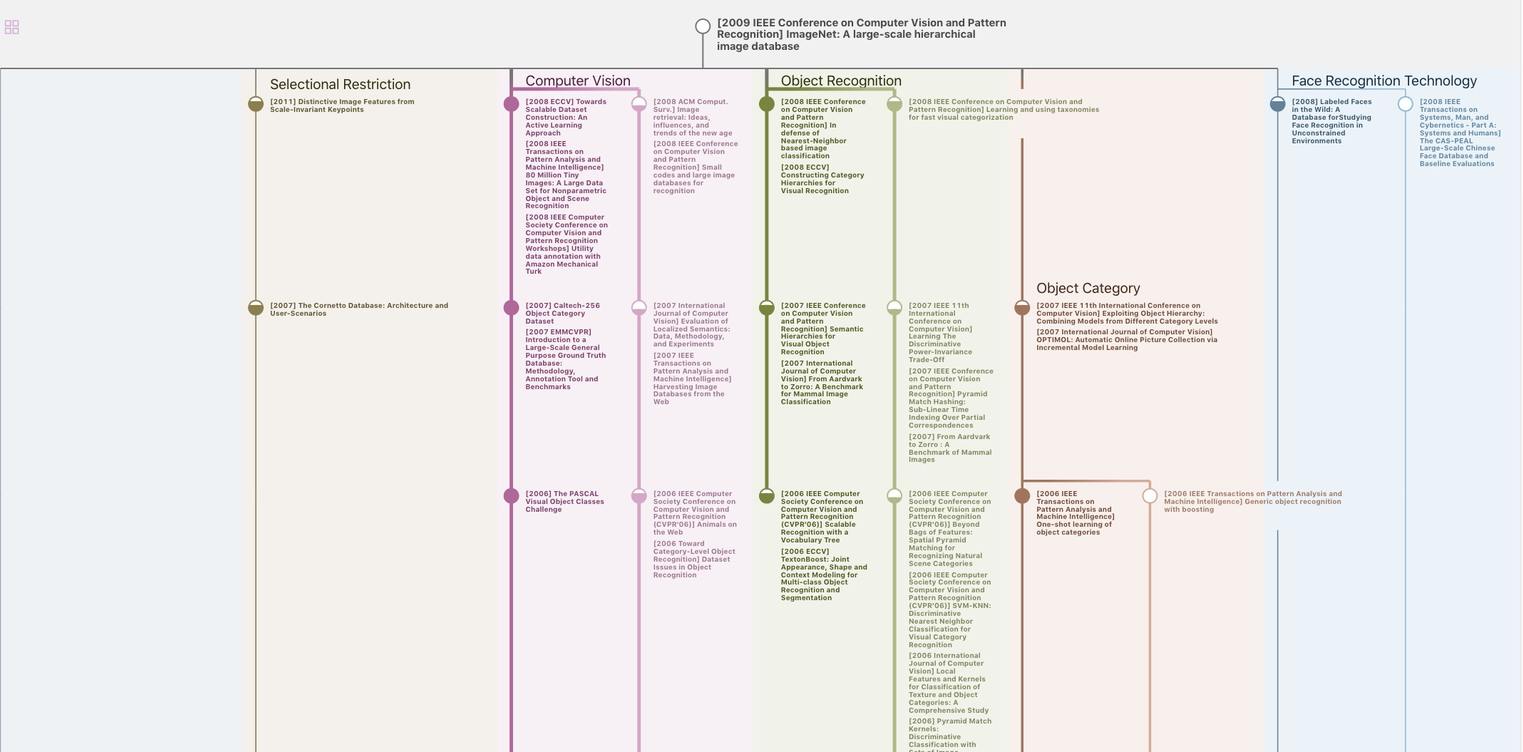
生成溯源树,研究论文发展脉络
Chat Paper
正在生成论文摘要