Discovering Sparse and Ununiform Lag Structure Using VAR Models with Latent Group LASSO
2019 International Conference on Data Mining Workshops (ICDMW)(2019)
Abstract
Structurally regularized learning methods have been attracting attention in the field of statistics, machine learning, and signal processing as useful analysis tools for learning in high-dimensional settings. Using these methods, specific structure can be represented in parameters. In multivariate time series data, it is important to capture time-delay structure where necessary lag length to explain each variable is different. In this paper, we deal with a problem to discover the optimal number of order for each variable in VAR models. When we apply conventional structurally regularized learning methods such as Group Lasso, some problems, which include loss of necessary coefficients, occur. To overcome these problems, we propose a method based on the Latent Group LASSO regularization. To apply it to VAR models, we introduce overlapped coefficient groups in time direction and use a heavy-tailed distribution, such as Student's t-distribution, as a prior to enhance its sparsity. We evaluated the effectiveness of the proposed method using artificial data and actual data set (Beijing PM2.5 data set). As the result, we obtained the sparse models that capture ununiform time-delay structure represented by fewer variables with the same degree of forecast accuracy comparing with ordinary VAR models.
MoreTranslated text
Key words
structure, time series, time delay, sparsity, latent
AI Read Science
Must-Reading Tree
Example
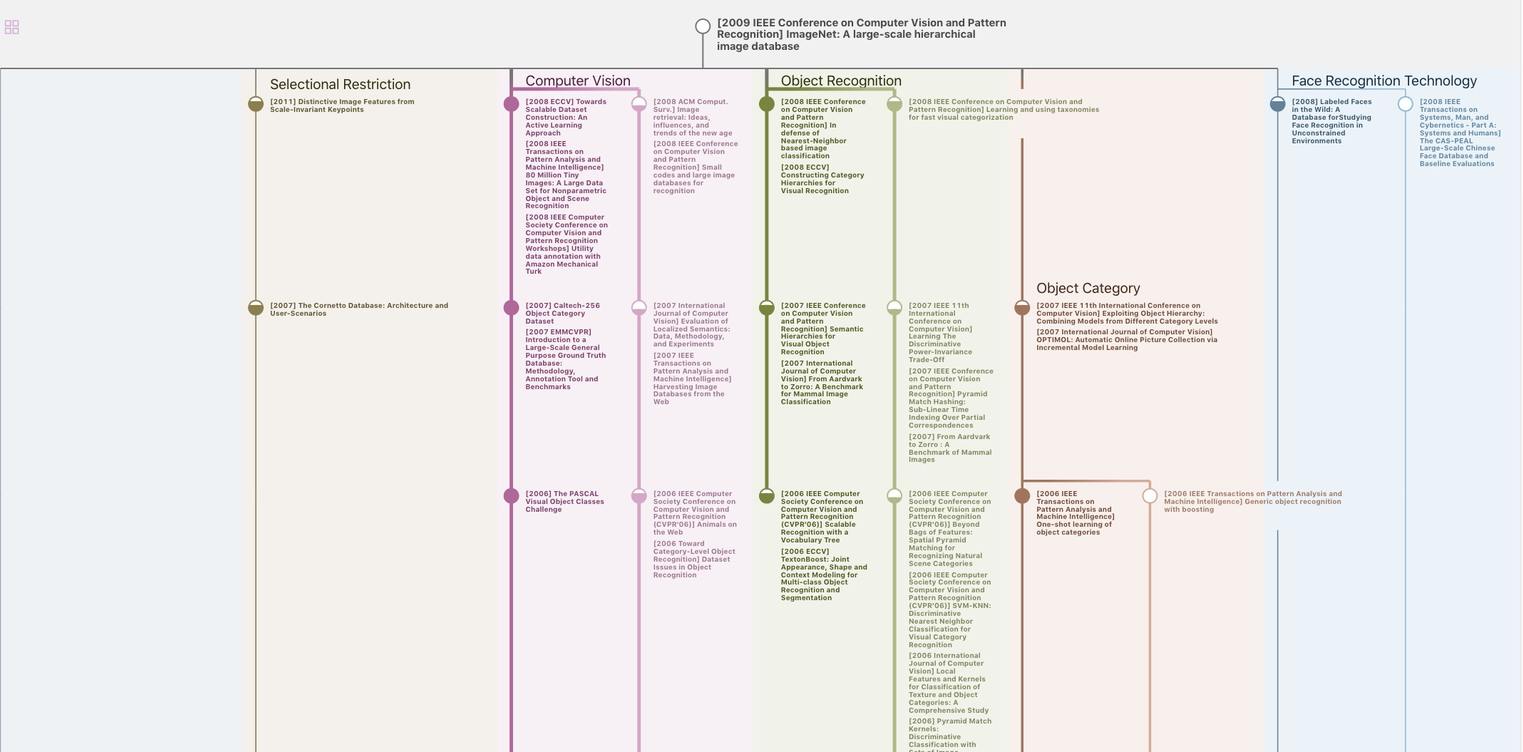
Generate MRT to find the research sequence of this paper
Chat Paper
Summary is being generated by the instructions you defined