Learning Theory and Algorithms for Revenue Maximization in Sponsored Search
2019 International Conference on Data Mining Workshops (ICDMW)(2019)
摘要
Online advertising is the main source of revenue for Internet business. Almost in all the Ads platforms, advertisements are ranked by jointly considering the ads' estimated click-through rates and their bidding prices. But in practice, most of existing methods focus on estimating eCTR as accurately as possible and then incorporate the bidding price into the ranking phase, instead of modeling the expected ads revenue directly, mainly because of the absence of a good optimization target for expected revenue maximization. In this paper we propose two innovative metrics: real-valued AUC (rAUC) and soft AUC (sAUC) to evaluate the ads ranking model's performance and we prove that optimizing the proposed metrics is approximately equivalent to maximizing expected revenue. The proposed metrics bring in a great advantage that we can solve expected revenue maximization problem numerically rather than using heuristics following a famous Explicit Ranking Function (ERF). We also explore the Implicit Ranking Function (IRF) to maximize revenue through maximizing sAUC efficiently. Our experiments on two real-world platforms (Alibaba.com and Aliexpress.com) and also on Yahoo's open dataset show that our proposed methods perform better than the state-of-the-art methods.
更多查看译文
关键词
sponsored search,data mining,revenue maximization,e-commerce
AI 理解论文
溯源树
样例
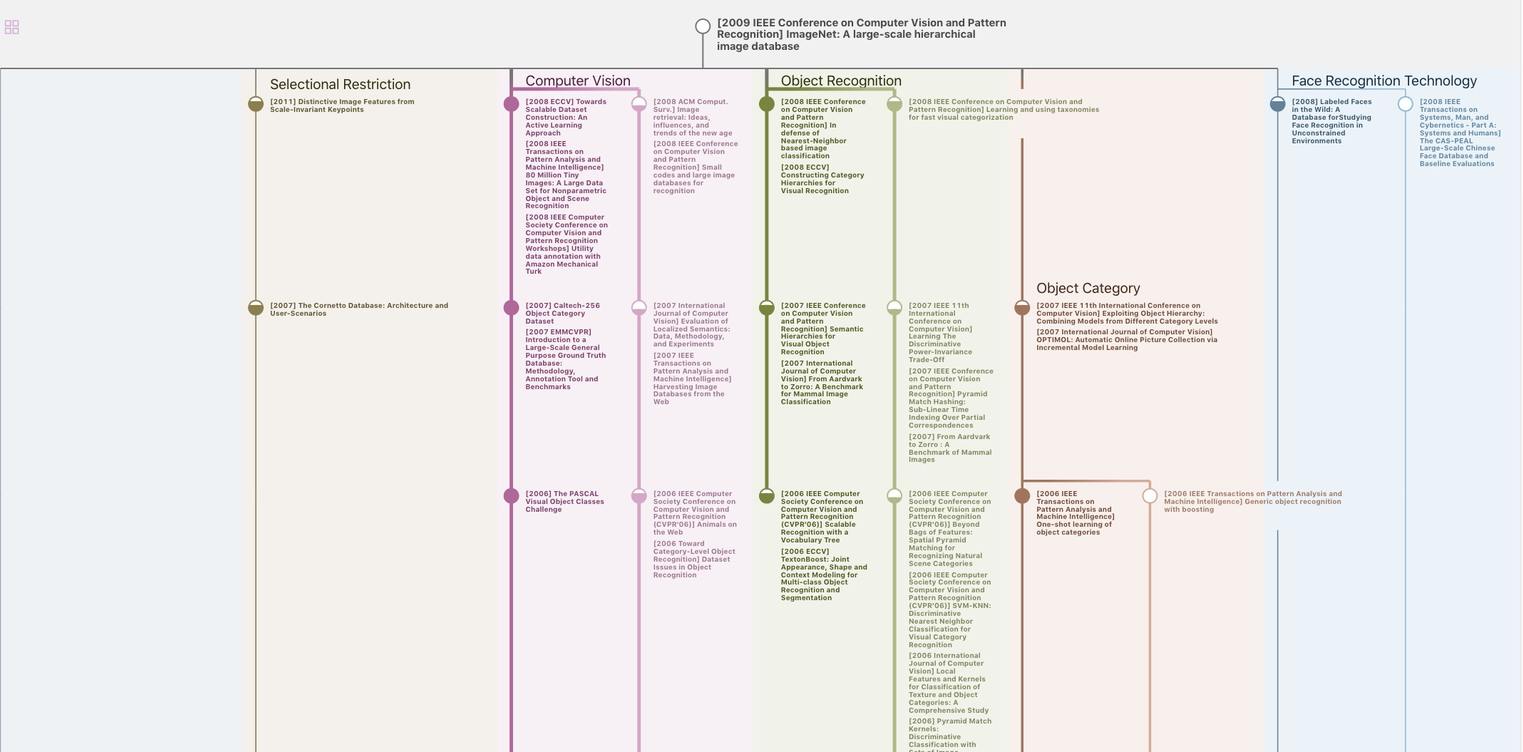
生成溯源树,研究论文发展脉络
Chat Paper
正在生成论文摘要