Improve the generalization of the cross-task emotion classifier using EEG based on feature selection and SVR
2019 IEEE 10th International Conference on Awareness Science and Technology (iCAST)(2019)
摘要
Emotion is a state that comprehensively represents human feeling, thought and behavior. In our daily life, emotion has played an increasingly important role, and emotion recognition has become a research focus. What' more, the application has a broader perspective at home and abroad. Most existing studies identified emotion under specific tasks, but emotion classifiers are required to recognize emotion under any conditions in practice. Therefore, cross-task emotion recognition is a necessary step to move from the laboratory to the practical use. In this work, we designed three different induced tasks, picture-induced, music-induced and video-induced tasks. 13 (8 females and 5 males) participants were recruited and evoked to be positive, neutral and negative states respectively. The results using support vector regression highlighted that the correlation coefficient was higher for inter-task classification in video-induced and music-induced tasks, while deteriorated significantly in cross-task classification. Combining recursive feature screening and support vector regression to optimize features, the optimal feature set had better performance than all features employed, obtaining above 0.8 for correlation coefficient. These results indicated that SVR could achieve a better performance of cross-task emotion recognition, partly because it avoided the problem of emotion intensity mismatch in different tasks.
更多查看译文
关键词
EEG,emotion recognition,cross-task,emotion classifiers,recursive feature screening,SVR
AI 理解论文
溯源树
样例
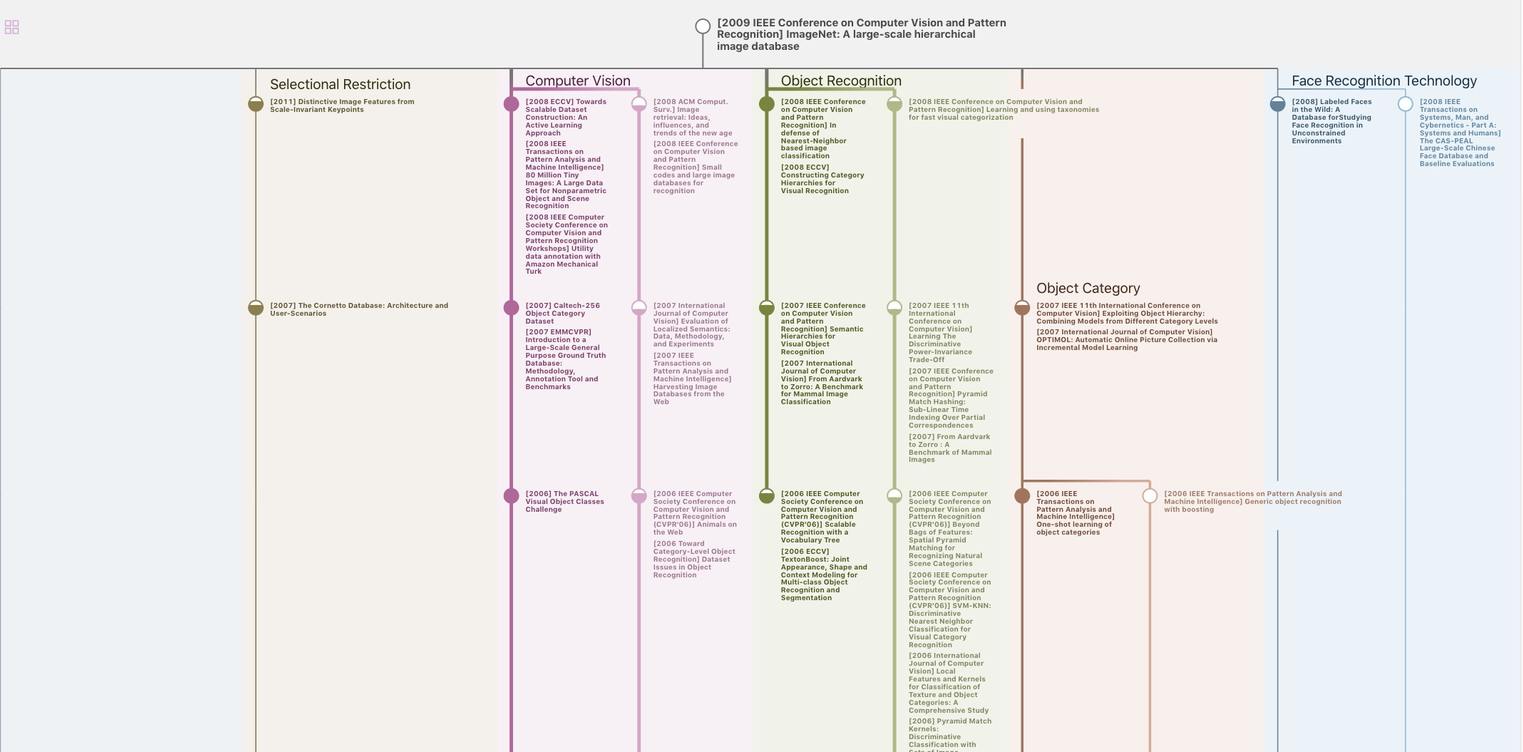
生成溯源树,研究论文发展脉络
Chat Paper
正在生成论文摘要