Modeling the diffusion-weighted imaging signal for breast lesions in the b = 200 to 3000 s/mm 2 range: quality of fit and classification accuracy for different representations.
MAGNETIC RESONANCE IN MEDICINE(2020)
摘要
Purpose To evaluate different non-Gaussian representations for the diffusion-weighted imaging (DWI) signal in the b-value range 200 to 3000 s/mm(2) in benign and malignant breast lesions. Methods Forty-three patients diagnosed with benign (n = 18) or malignant (n = 25) tumors of the breast underwent DWI (b-values 200, 600, 1200, 1800, 2400, and 3000 s/mm(2)). Six different representations were fit to the average signal from regions of interest (ROIs) at different b-value ranges. Quality of fit was assessed by the corrected Akaike information criterion (AICc), and the Friedman test was used for assessing representation ranks. The area under the curve (AUC) of receiver operating characteristic curves were used to evaluate the power of derived parameters to differentiate between malignant and benign lesions. The lesion ROI was divided in central and peripheral parts to assess potential effect of heterogeneity. Sensitivity to noise-floor correction was also evaluated. Results The Pade exponent was ranked as the best based on AICc, whereas 3 models (kurtosis, fractional, and biexponential) achieved the highest AUC = 0.99 for lesion differentiation. The monoexponential model at b(max) = 600 s/mm(2) already provides AUC = 0.96, with considerably shorter acquisition time and simpler analysis. Significant differences between central and peripheral parts of lesions were found in malignant lesions. The mono- and biexponential models were most stable against varying degrees of noise-floor correction. Conclusion Non-Gaussian representations are required for fitting of the DWI curve at high b-values in breast lesions. However, the added clinical value from the high b-value data for differentiation of benign and malignant lesions is not clear.
更多查看译文
关键词
biexponential model,breast MR,diffusion-weighted MRI,DKI,fractional order calculus,Pade exponent,statistical diffusion model,stretched exponential
AI 理解论文
溯源树
样例
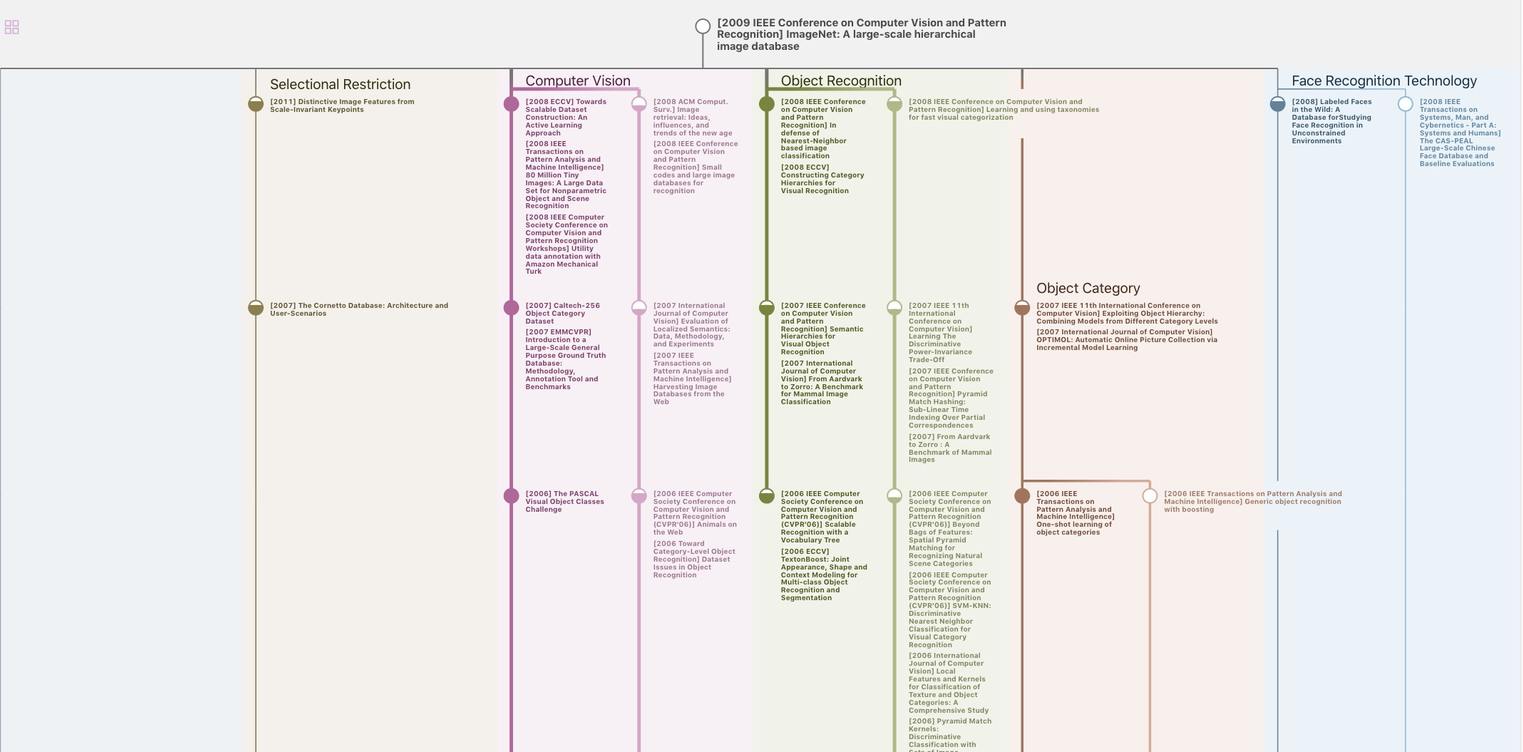
生成溯源树,研究论文发展脉络
Chat Paper
正在生成论文摘要