Sequential Modeling of Hierarchical User Intention and Preference for Next-item Recommendation.
WSDM '20: The Thirteenth ACM International Conference on Web Search and Data Mining Houston TX USA February, 2020(2020)
摘要
The next-item recommendation has attracted great research interests with both static and dynamic users' preferences considered. Existing approaches typically utilize user-item binary relations, and assume a flat preference distribution over items for each user. However, this assumption neglects the hierarchical discrimination between user intentions and user preferences, causing the methods have limited capacity to depict intention-specific preference. In fact, a consumer's purchasing behavior involves a natural sequential process, i.e., he/she first has an intention to buy one type of items, followed by choosing a specific item according to his/her preference under this intention. To this end, we propose a novel key-array memory network (KA-MemNN), which takes both user intentions and preferences into account for next-item recommendation. Specifically, the user behavioral intention tendency is determined through key addressing. Further, each array outputs an intention-specific preference representation of a user. Then, the degree of user's behavioral intention tendency and intention-specific preference representation are combined to form a hierarchical representation of a user. This representation is further utilized to replace the static profile of users in traditional matrix factorization for the purposes of reasoning. The experimental results on real-world data demonstrate the advantages of our approach over state-of-the-art methods.
更多查看译文
关键词
Representation learning, memory networks, intention modeling
AI 理解论文
溯源树
样例
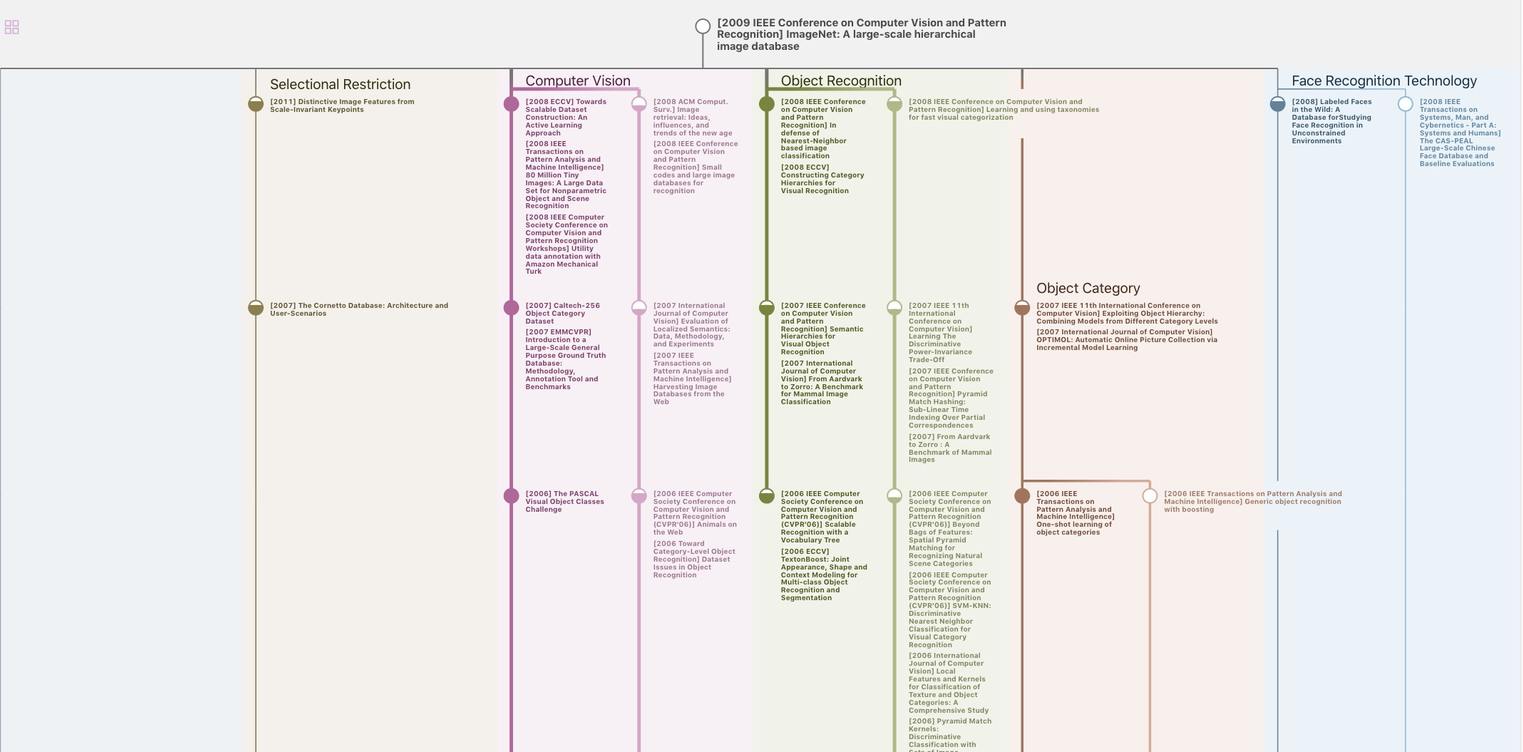
生成溯源树,研究论文发展脉络
Chat Paper
正在生成论文摘要