Deep Dual-Channel Neural Network for Image-Based Smoke Detection
IEEE Transactions on Multimedia(2020)
摘要
Smoke detection plays an important role in industrial safety warning systems and fire prevention. Due to the complicated changes in the shape, texture, and color of smoke, identifying the smoke from a given image still remains a substantial challenge, and this has accordingly aroused a considerable amount of research attention recently. To address the problem, we devise a new deep dual-channel neural network (DCNN) for smoke detection. In contrast to popular deep convolutional networks (e.g., Alex-Net, VGG-Net, Res-Net, and Dense-Net and the DNCNN that is specifically devoted to detecting smoke), our proposed end-to-end network is mainly composed of dual channels of deep subnetworks. In the first subnetwork, we sequentially connect multiple convolutional layers and max-pooling layers. Then, we selectively append the batch normalization layer to each convolutional layer for overfitting reduction and training acceleration. The first subnetwork is shown to be good at extracting the detailed information of smoke, such as texture. In the second subnetwork, in addition to the convolutional, batch normalization, and max-pooling layers, we further introduce two important components. One is the skip connection for avoiding the vanishing gradient and improving the feature propagation. The other is the global average pooling for reducing the number of parameters and mitigating the overfitting issue. The second subnetwork can capture the base information of smoke, such as contours. We finally deploy a concatenation operation to combine the aforementioned two deep subnetworks to complement each other. Based on the augmented data obtained by rotating the training images, our proposed DCNN can promptly and stably converge to the perfect performance. Experimental results conducted on the publicly available smoke detection database verify that the proposed DCNN has attained a very high detection rate that exceeds 99.5% on average, superior to state-of-the-art relevant competitors. Furthermore, our DCNN only employs approximately one-third of the parameters needed by the comparatively tested deep neural networks. The source code of DCNN will be released at
https://kegu.netlify.com/
.
更多查看译文
关键词
Feature extraction,Neural networks,Training,Deep learning,Convolutional codes,Convolution,Safety
AI 理解论文
溯源树
样例
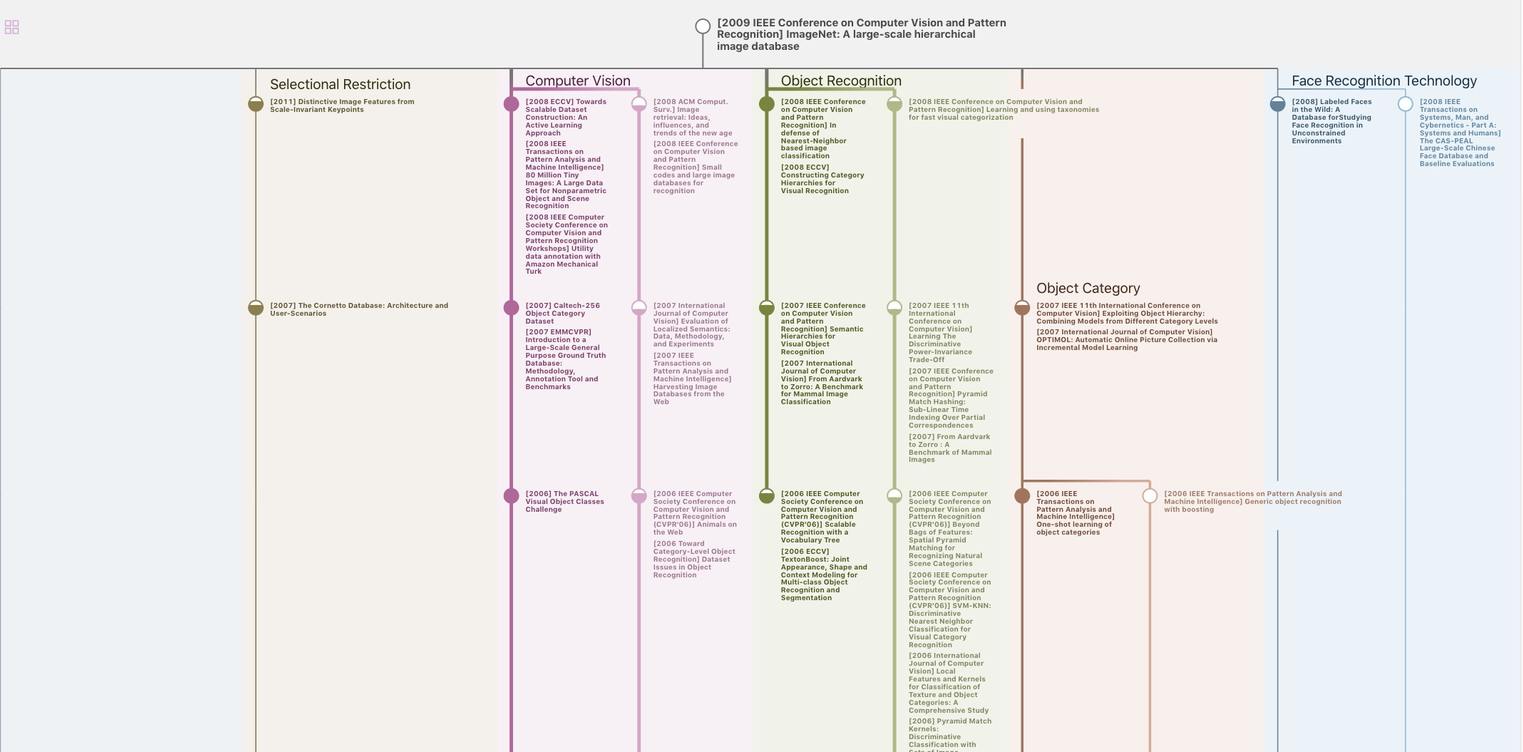
生成溯源树,研究论文发展脉络
Chat Paper
正在生成论文摘要