Reasoning About Generalization via Conditional Mutual Information
COLT(2020)
摘要
We provide an information-theoretic framework for studying the generalization properties of machine learning algorithms. Our framework ties together existing approaches, including uniform convergence bounds and recent methods for adaptive data analysis. Specifically, we use Conditional Mutual Information (CMI) to quantify how well the input (i.e., the training data) can be recognized given the output (i.e., the trained model) of the learning algorithm. We show that bounds on CMI can be obtained from VC dimension, compression schemes, differential privacy, and other methods. We then show that bounded CMI implies various forms of generalization.
更多查看译文
关键词
generalization,information,reasoning
AI 理解论文
溯源树
样例
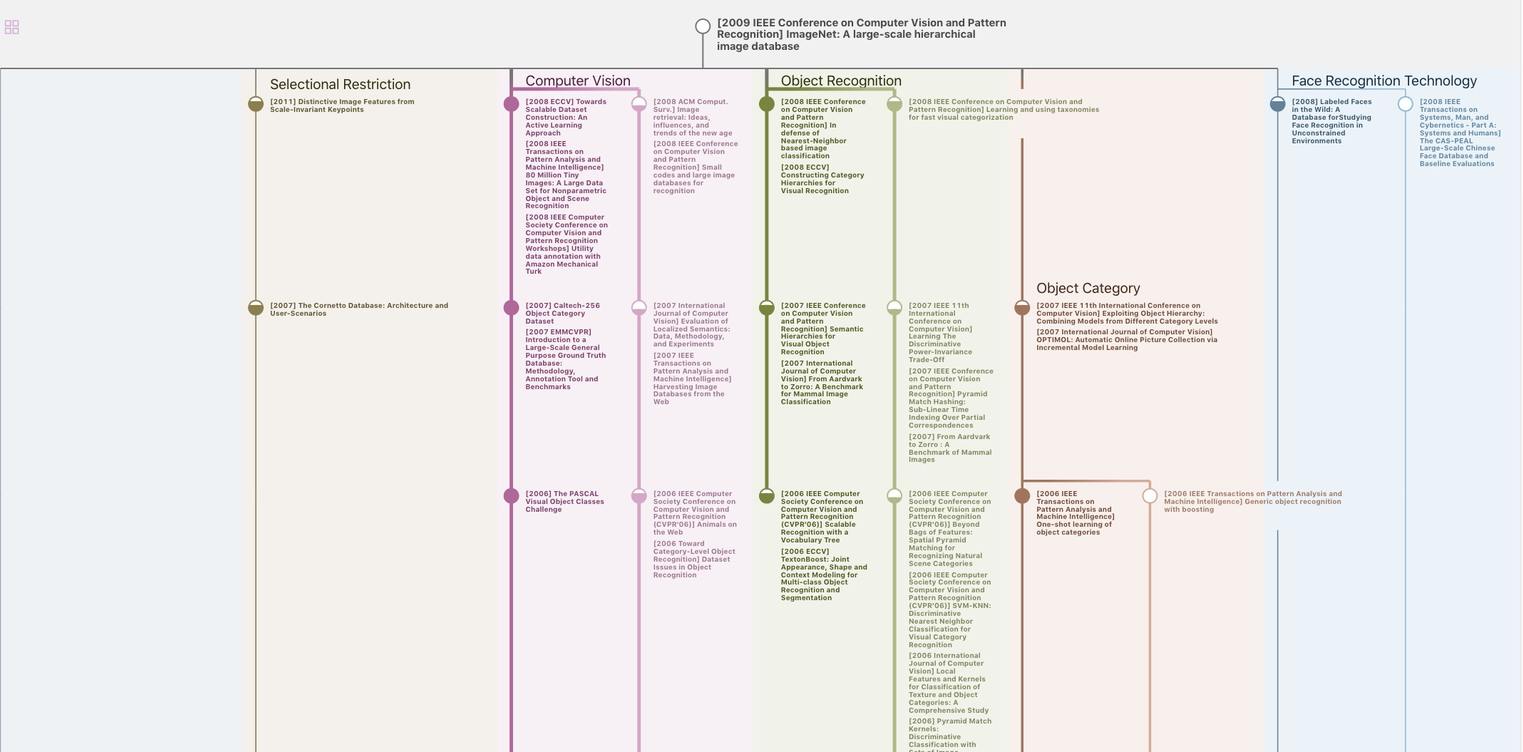
生成溯源树,研究论文发展脉络
Chat Paper
正在生成论文摘要