The POLAR Framework: Polar Opposites Enable Interpretability of Pre-Trained Word Embeddings
WWW '20: The Web Conference 2020 Taipei Taiwan April, 2020(2020)
摘要
We introduce ‘POLAR’ — a framework that adds interpretability to pre-trained word embeddings via the adoption of semantic differentials. Semantic differentials are a psychometric construct for measuring the semantics of a word by analysing its position on a scale between two polar opposites (e.g., cold – hot, soft – hard). The core idea of our approach is to transform existing, pre-trained word embeddings via semantic differentials to a new “polar” space with interpretable dimensions defined by such polar opposites. Our framework also allows for selecting the most discriminative dimensions from a set of polar dimensions provided by an oracle, i.e., an external source. We demonstrate the effectiveness of our framework by deploying it to various downstream tasks, in which our interpretable word embeddings achieve a performance that is comparable to the original word embeddings. We also show that the interpretable dimensions selected by our framework align with human judgement. Together, these results demonstrate that interpretability can be added to word embeddings without compromising performance. Our work is relevant for researchers and engineers interested in interpreting pre-trained word embeddings.
更多查看译文
关键词
word embeddings, neural networks, interpretable, semantic differential
AI 理解论文
溯源树
样例
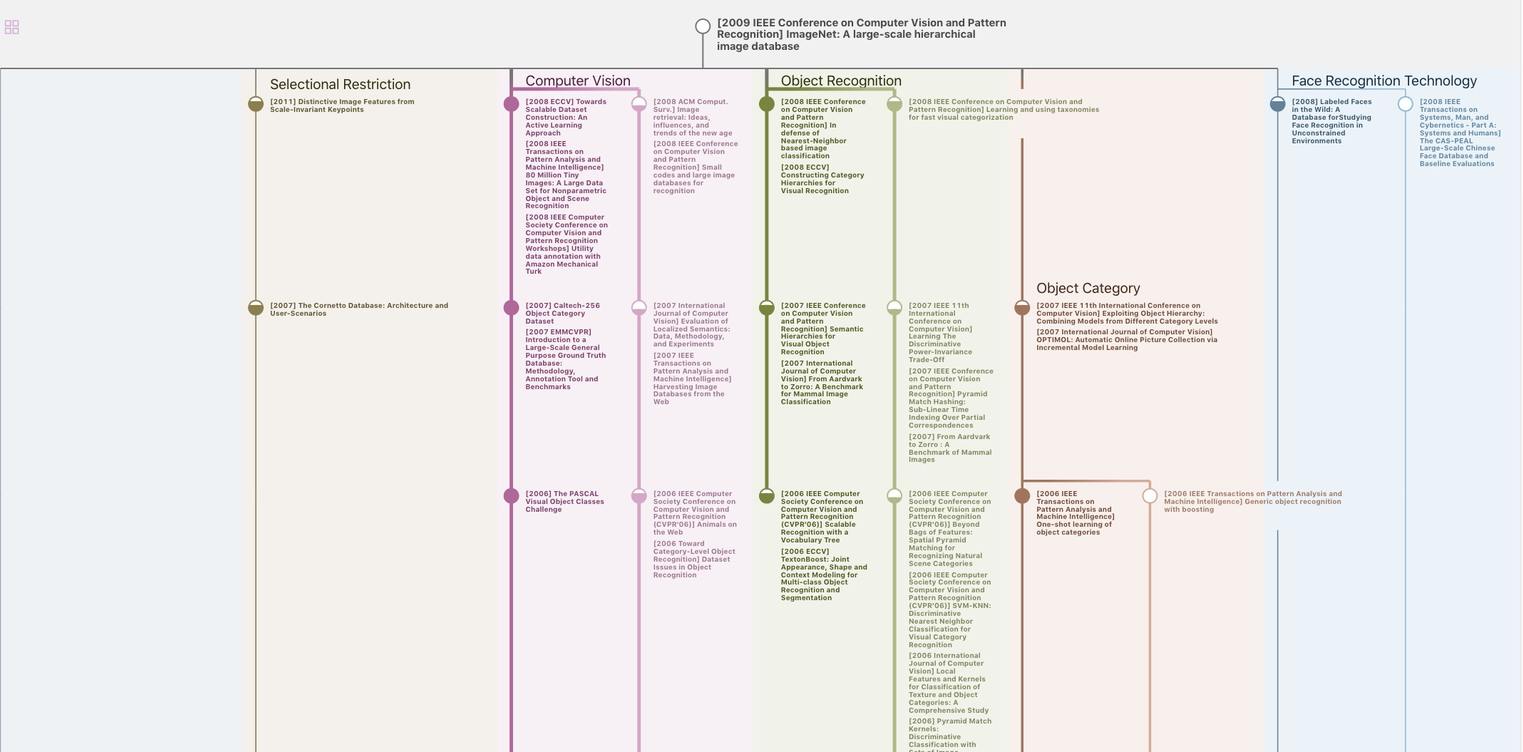
生成溯源树,研究论文发展脉络
Chat Paper
正在生成论文摘要