Rotation, Translation, and Cropping for Zero-Shot Generalization
2020 IEEE Conference on Games (CoG)(2020)
摘要
Deep Reinforcement Learning (DRL) has shown impressive performance on domains with visual inputs, in particular various games. However, the agent is usually trained on a fixed environment, e.g. a fixed number of levels. A growing mass of evidence suggests that these trained models fail to generalize to even slight variations of the environments they were trained on. This paper advances the hypothesis that the lack of generalization is partly due to the input representation, and explores how rotation, cropping and translation could increase generality. We show that a cropped, translated and rotated observation can get better generalization on unseen levels of two-dimensional arcade games from the GVGAI framework. The generality of the agents is evaluated on both human-designed and procedurally generated levels.
更多查看译文
关键词
generalization,reinforcement learning,representation,A2C,zero-shot generalization,gvgai
AI 理解论文
溯源树
样例
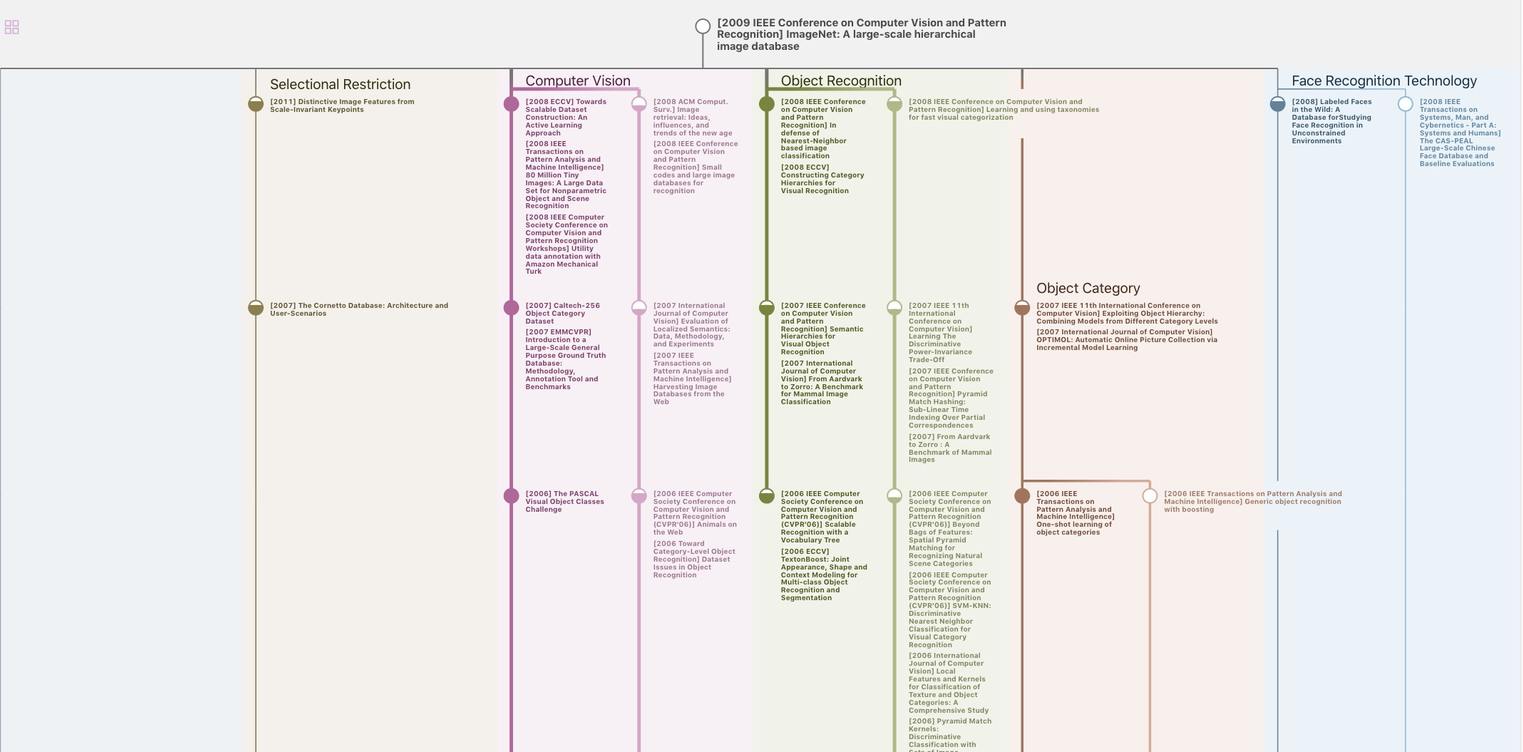
生成溯源树,研究论文发展脉络
Chat Paper
正在生成论文摘要