Statistical Exploration of Relationships Between Routine and Agnostic Features Towards Interpretable Risk Characterization
arxiv(2020)
摘要
As is typical in other fields of application of high throughput systems, radiology is faced with the challenge of interpreting increasingly sophisticated predictive models such as those derived from radiomics analyses. Interpretation may be guided by the learning output from machine learning models, which may however vary greatly with each technique. Whatever this output model, it will raise some essential questions. How do we interpret the prognostic model for clinical implementation? How can we identify potential information structures within sets of radiomic features, in order to create clinically interpretable models? And how can we recombine or exploit potential relationships between features towards improved interpretability? A number of statistical techniques are explored to assess (possibly nonlinear) relationships between radiological features from different angles.
更多查看译文
AI 理解论文
溯源树
样例
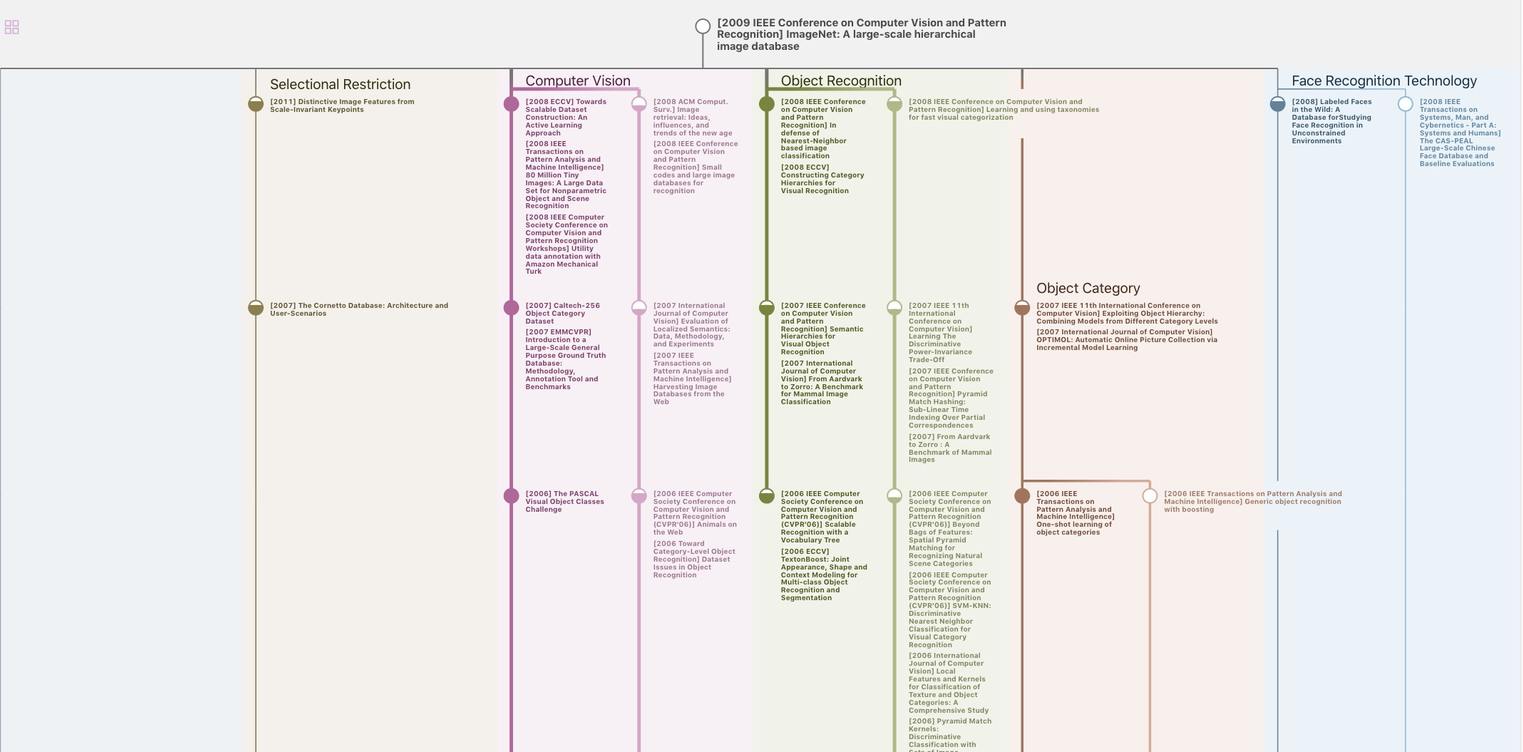
生成溯源树,研究论文发展脉络
Chat Paper
正在生成论文摘要