Semi-Supervised Cross-Modal Hashing With Graph Convolutional Networks
DATABASES THEORY AND APPLICATIONS, ADC 2020(2020)
摘要
Cross-modal hashing for large-scale approximate neighbor search has attracted great attention recently because of its significant computational and storage efficiency. However, it is still challenging to generate high-quality binary codes to preserve inter-modal and intra-modal semantics, especially in a semi-supervised manner. In this paper, we propose a semi-supervised cross-modal discrete code learning framework. This is the very first work of applying asymmetric graph convolutional networks (GCNs) for scalable cross-modal retrieval. Specifically, the architecture contains multiple GCN branches, each of which is for one data modality to extract modality-specific features and then to generate unified binary hash codes across different modalities, so that the underlying correlations and similarities across modalities are simultaneously preserved into the hash values. Moreover, the branches are built with asymmetric graph convolutional layers, which employ randomly sampled anchors to tackle the scalability and out-of-sample issue in graph learning, and reduce the complexity of cross-modal similarity calculation. Extensive experiments conducted on benchmark datasets demonstrate that our method can achieve superior retrieval performance in comparison with the state-of-the-art methods.
更多查看译文
关键词
Cross-modal hashing, GCN, Semi-supervised learning
AI 理解论文
溯源树
样例
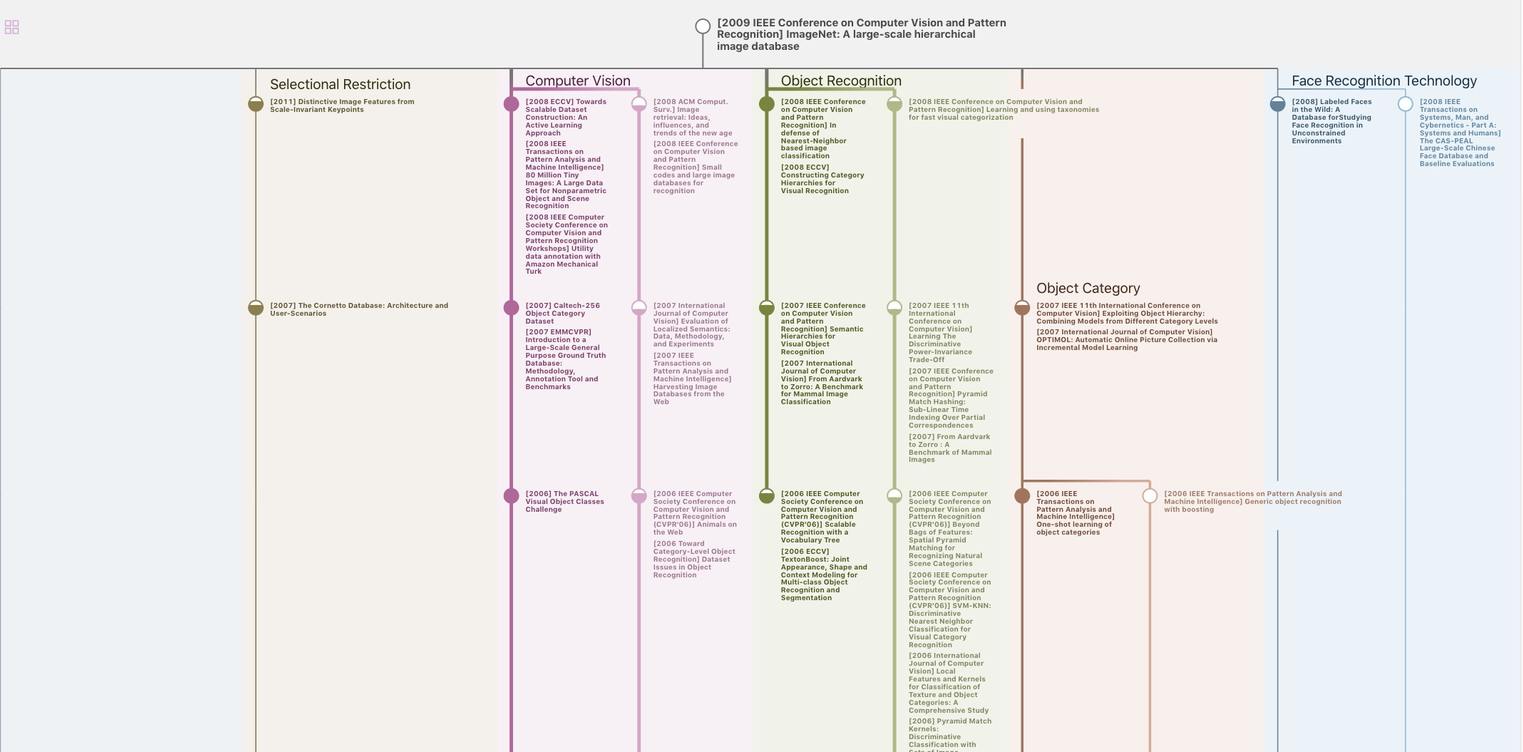
生成溯源树,研究论文发展脉络
Chat Paper
正在生成论文摘要