Compact Recurrent Neural Networks for Acoustic Event Detection on Low-Energy Low-Complexity Platforms
IEEE Journal of Selected Topics in Signal Processing(2020)
摘要
Outdoor acoustic event detection is an exciting research field but challenged by the need for complex algorithms and deep learning techniques, typically requiring many computational, memory, and energy resources. These challenges discourage IoT implementations, where an efficient use of resources is required. However, current embedded technologies and microcontrollers have increased their capabilities without penalizing energy efficiency. This paper addresses the application of sound event detection at the very edge, by optimizing deep learning techniques on resource-constrained embedded platforms for the IoT. The contribution is two-fold: firstly, a two-stage student-teacher approach is presented to make state-of-the-art neural networks for sound event detection fit on current microcontrollers; secondly, we test our approach on an ARM Cortex M4, particularly focusing on issues related to 8-bits quantization. Our embedded implementation can achieve 68% accuracy in recognition on Urbansound8k, not far from state-of-the-art performance, with an inference time of 125 ms for each second of the audio stream, and power consumption of 5.5 mW in just 34.3 kB of RAM.
更多查看译文
关键词
Sound event detection,knowledge distillation,internet of things,deep learning,quantized neural network,microcontroller
AI 理解论文
溯源树
样例
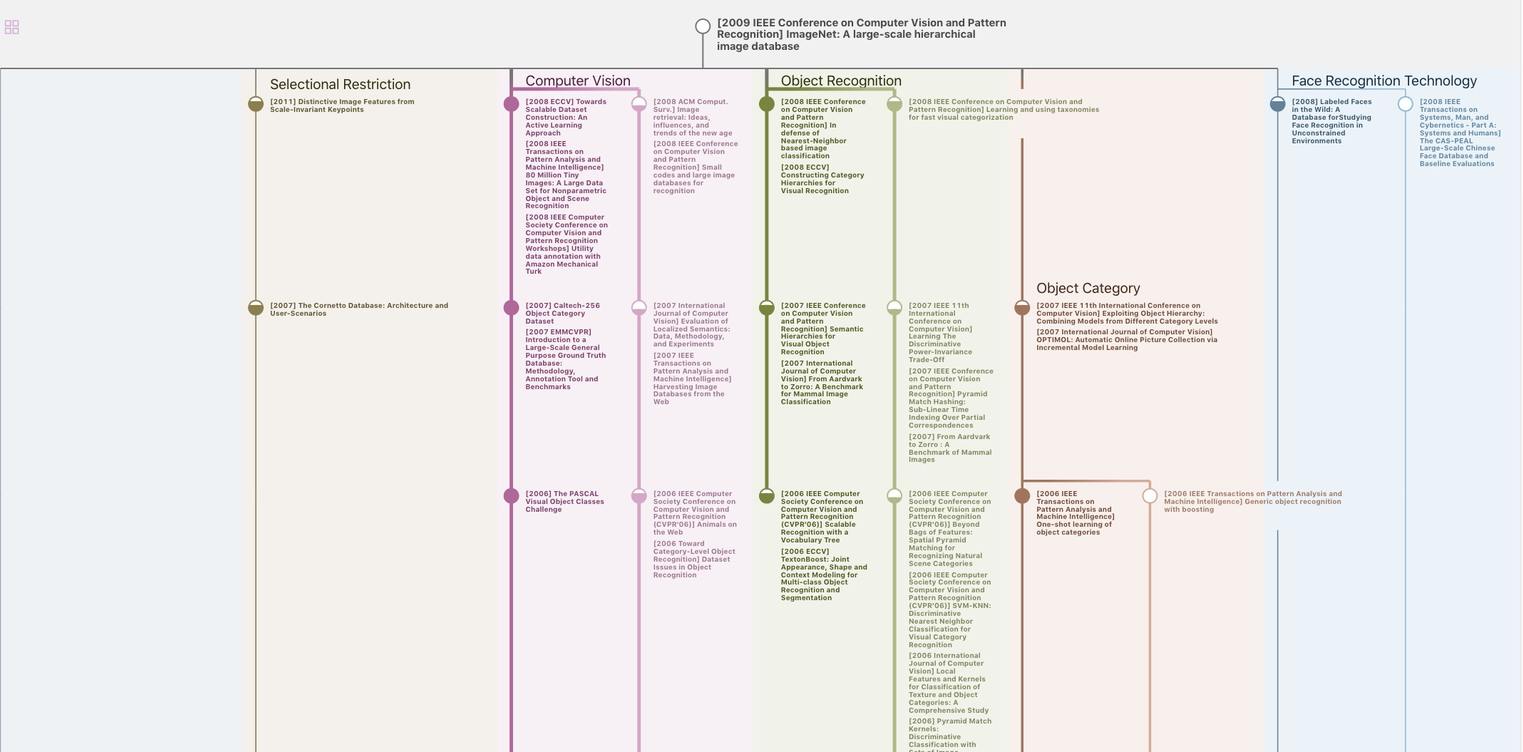
生成溯源树,研究论文发展脉络
Chat Paper
正在生成论文摘要