Semi-supervised Flexible Joint Distribution Adaptation.
ICNCC(2019)
摘要
For the traditional feature transfer method, there are problems that the projection transformation is too rigid and data manifold structure cannot be captured inadequately. In this paper, we propose a feature extraction method with the ability of knowledge transfer named Semi-supervised Flexible Joint Distribution Adaptation (SFJDA). Firstly, we introduce a flexible transformation constraint instead of the traditional linear projection into Joint Distribution Adaptation (JDA) to relax this constraint and extract shared feature between source and target domains. Secondly, Manifold Alignment (MA) is introduced to mine geometric information of the source and target domains. Finally, Linear Discriminant Analysis (LDA) and its kernel form are integrated into the objective function to keep class separability during label refinement procedure. Experimental results on 36 groups of image datasets in the classification task validate the feasibility and effectiveness of the proposed algorithm.
更多查看译文
AI 理解论文
溯源树
样例
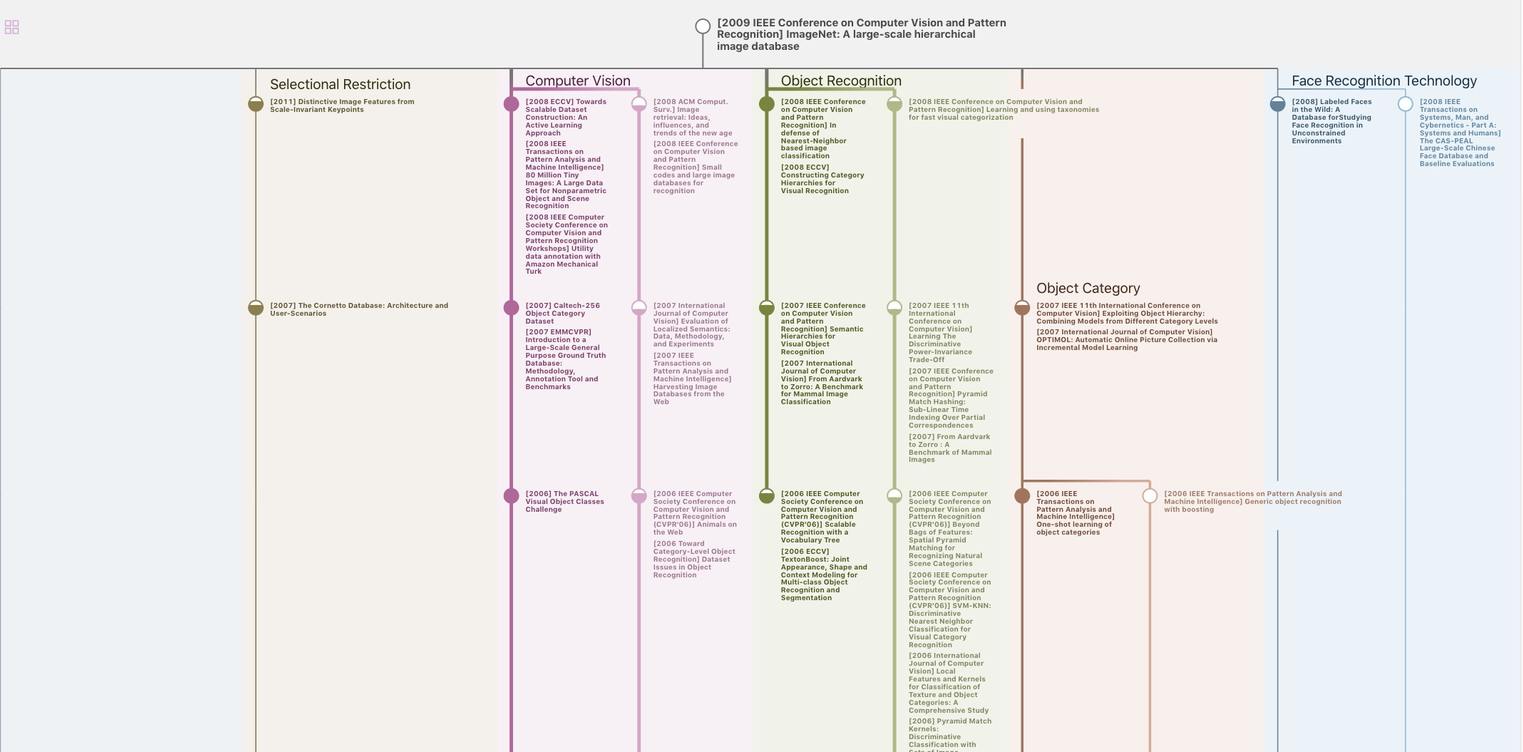
生成溯源树,研究论文发展脉络
Chat Paper
正在生成论文摘要