Ensemble Grammar Induction For Detecting Anomalies in Time Series.
arXiv (Cornell University)(2020)
摘要
Time series anomaly detection is an important task, with applications in a broad variety of domains. Many approaches have been proposed in recent years, but often they require that the length of the anomalies be known in advance and provided as an input parameter. This limits the practicality of the algorithms, as such information is often unknown in advance, or anomalies with different lengths might co-exist in the data. To address this limitation, previously, a linear time anomaly detection algorithm based on grammar induction has been proposed. While the algorithm can find variable-length patterns, it still requires preselecting values for at least two parameters at the discretization step. How to choose these parameter values properly is still an open problem. In this paper, we introduce a grammar-induction-based anomaly detection method utilizing ensemble learning. Instead of using a particular choice of parameter values for anomaly detection, the method generates the final result based on a set of results obtained using different parameter values. We demonstrate that the proposed ensemble approach can outperform existing grammar-induction-based approaches with different criteria for selection of parameter values. We also show that the proposed approach can achieve performance similar to that of the state-of-the-art distance-based anomaly detection algorithm.
更多查看译文
关键词
ensemble grammar induction,detecting anomalies,time series
AI 理解论文
溯源树
样例
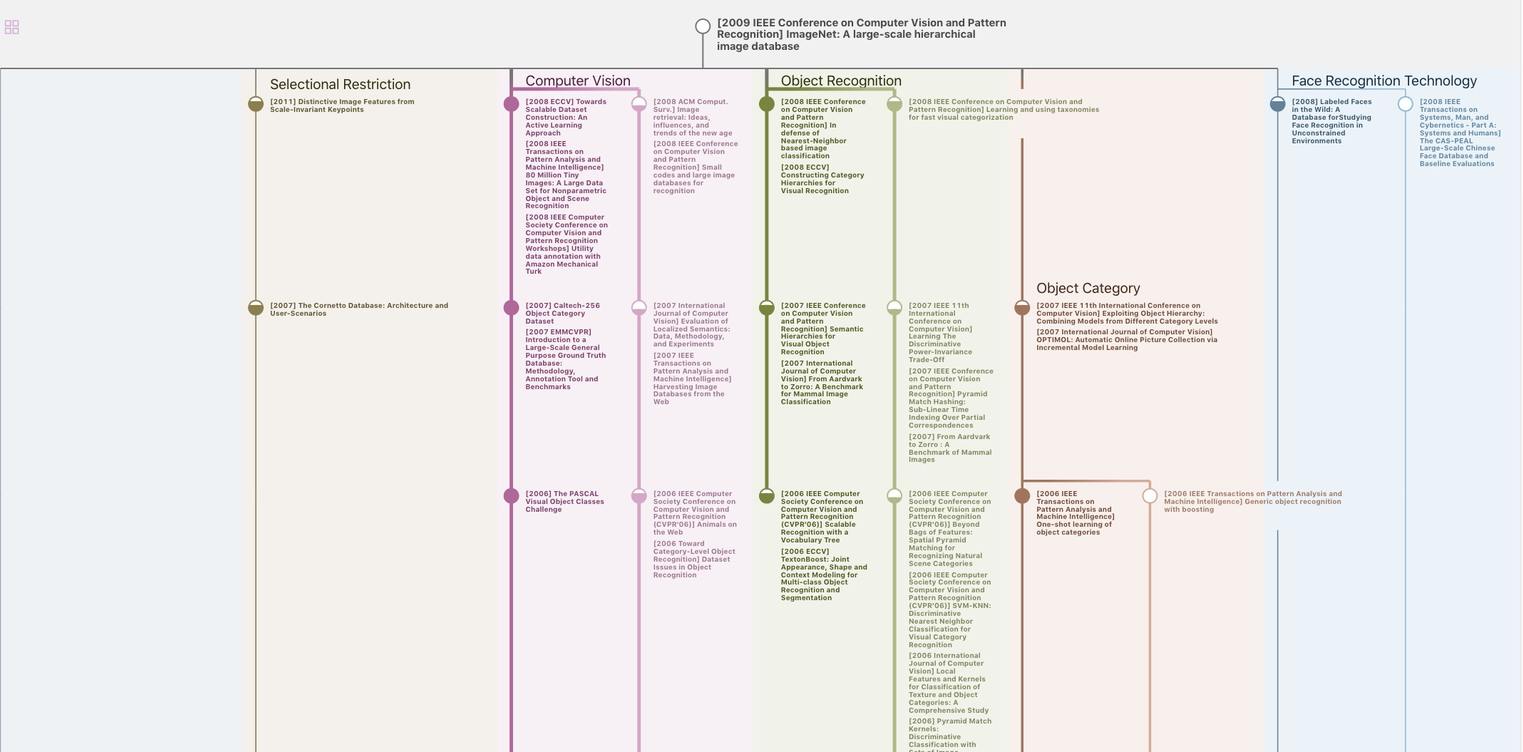
生成溯源树,研究论文发展脉络
Chat Paper
正在生成论文摘要