Exploiting Uncertainty in Popularity Prediction of Information Diffusion Cascades Using Self-exciting Point Processes
arxiv(2020)
摘要
Hawkes processes have been successfully applied to understand online information diffusion and popularity of online items. Most prior work concentrate on individually modeling successful diffusion cascades, while discarding smaller cascades which, however, account for a majority proportion of the available data. In this work, we propose a set of tools to leverage information in the small cascades: a joint fitting procedure that accounts for cascade size bias in the sample, a Borel mixture model and a clustering algorithm to uncover latent groups within these cascades, and the posterior final size distribution of Hawkes processes. On a dataset of Twitter cascades, we show that, compared to the state-of-art models, the proposed method improves the generalization performance on unseen data, delivers better prediction for final popularity and provides means to characterize online content from the way Twitter users discuss about it.
更多查看译文
AI 理解论文
溯源树
样例
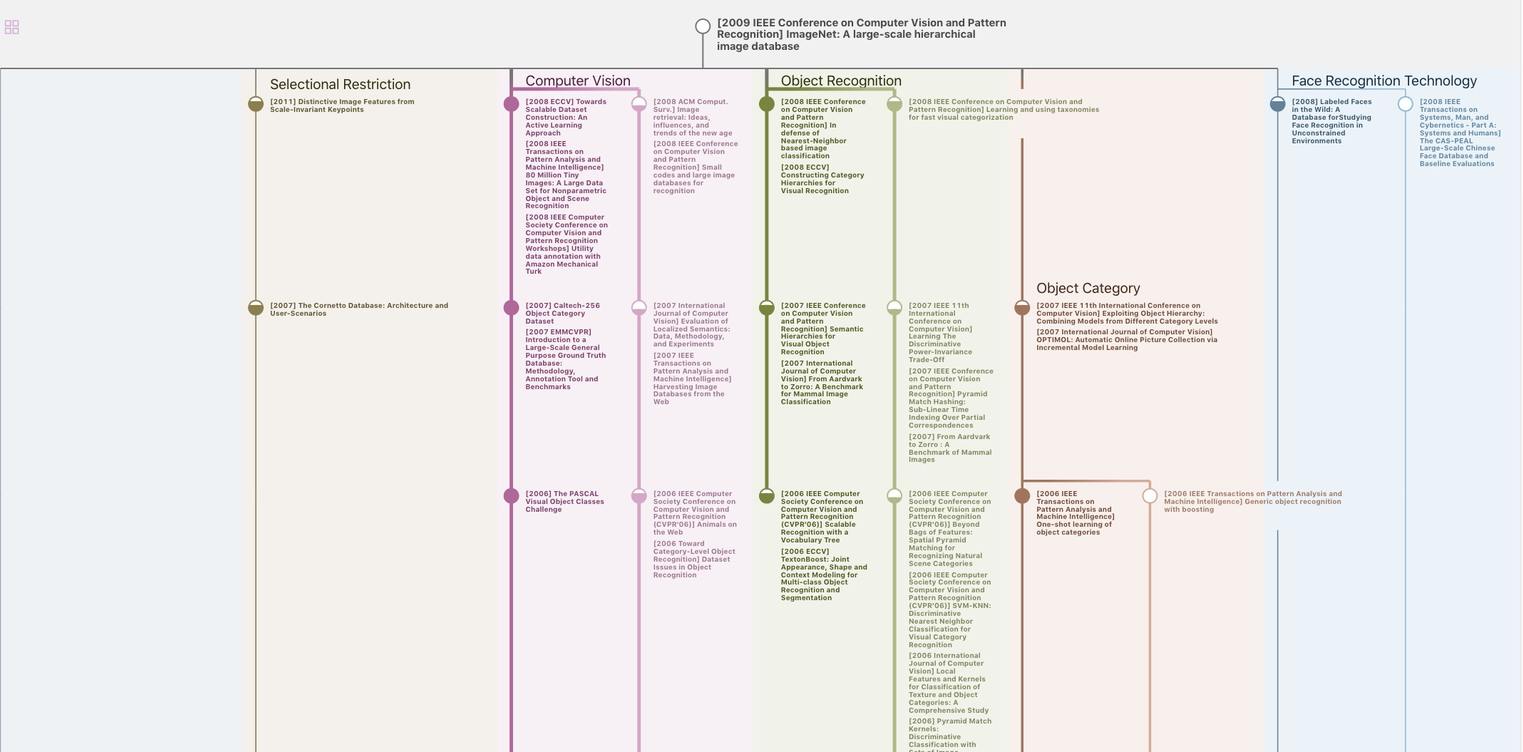
生成溯源树,研究论文发展脉络
Chat Paper
正在生成论文摘要