On Using Toeplitz and Circulant Matrices for Johnson–Lindenstrauss Transforms
Algorithmica(2019)
摘要
The Johnson–Lindenstrauss lemma is one of the cornerstone results in dimensionality reduction. A common formulation of it, is that there exists a random linear mapping f : ℝ^n →ℝ^m such that for any vector x ∈ℝ^n , f preserves its norm to within (1 ±ε ) with probability 1 - δ if m = (ε ^-2 (1/δ )) . Much effort has gone into developing fast embedding algorithms, with the Fast Johnson–Lindenstrauss transform of Ailon and Chazelle being one of the most well-known techniques. The current fastest algorithm that yields the optimal m = 𝒪(ε ^-2 (1/δ )) dimensions has an embedding time of 𝒪(n n + ε ^-2 ^3 (1/δ )) . An exciting approach towards improving this, due to Hinrichs and Vybíral, is to use a random m × n Toeplitz matrix for the embedding. Using Fast Fourier Transform, the embedding of a vector can then be computed in 𝒪(n m) time. The big question is of course whether m = 𝒪(ε ^-2 (1/δ )) dimensions suffice for this technique. If so, this would end a decades long quest to obtain faster and faster Johnson–Lindenstrauss transforms. The current best analysis of the embedding of Hinrichs and Vybíral shows that m = 𝒪(ε ^-2 ^2 (1/δ )) dimensions suffice. The main result of this paper, is a proof that this analysis unfortunately cannot be tightened any further, i.e., there exist vectors requiring m = (ε ^-2 ^2 (1/δ )) for the Toeplitz approach to work.
更多查看译文
关键词
Dimensionality reduction,Johnson–Lindenstrauss,Toeplitz matrices
AI 理解论文
溯源树
样例
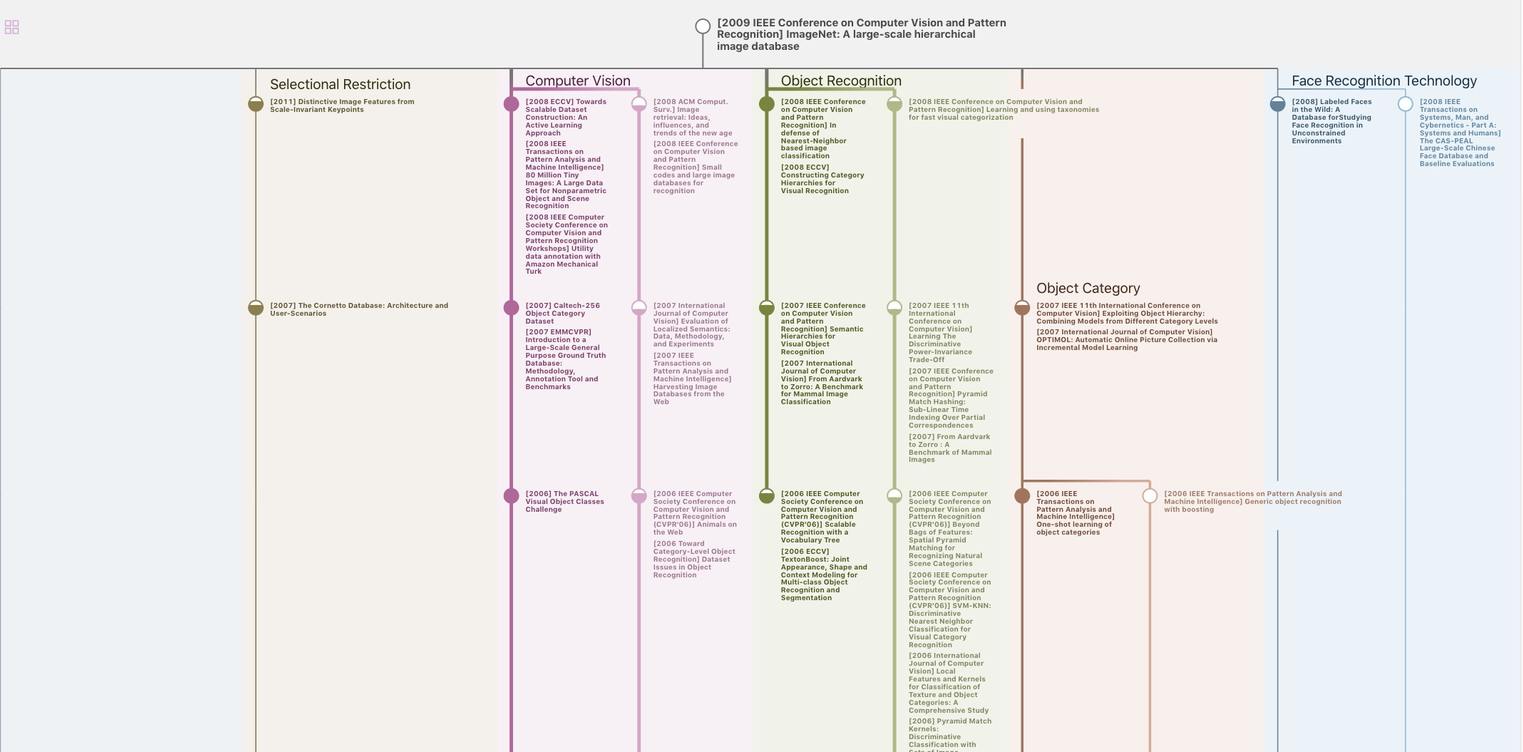
生成溯源树,研究论文发展脉络
Chat Paper
正在生成论文摘要