Differentially Private Release of Synthetic Graphs.
SODA '20: ACM-SIAM Symposium on Discrete Algorithms Salt Lake City Utah January, 2020(2020)
摘要
We propose a (ϵ, δ)-differentially private mechanism that, given an input graph G with n vertices and m edges, in polynomial time generates a synthetic graph G' approximating all cuts of the input graph up to an additive error of [MATH HERE]. This is the first construction of differentially private cut approximator that allows additive error o(m) for all m > nlogCn. The best known previous results gave additive O(n3/2) error and hence only retained information about the cut structure on very dense graphs. Thus, we are making a notable progress on a promiment problem in differential privacy. We also present lower bounds showing that our utility/privacy tradeoff is essentially the best possible if one seeks to get purely additive cut approximations.
更多查看译文
AI 理解论文
溯源树
样例
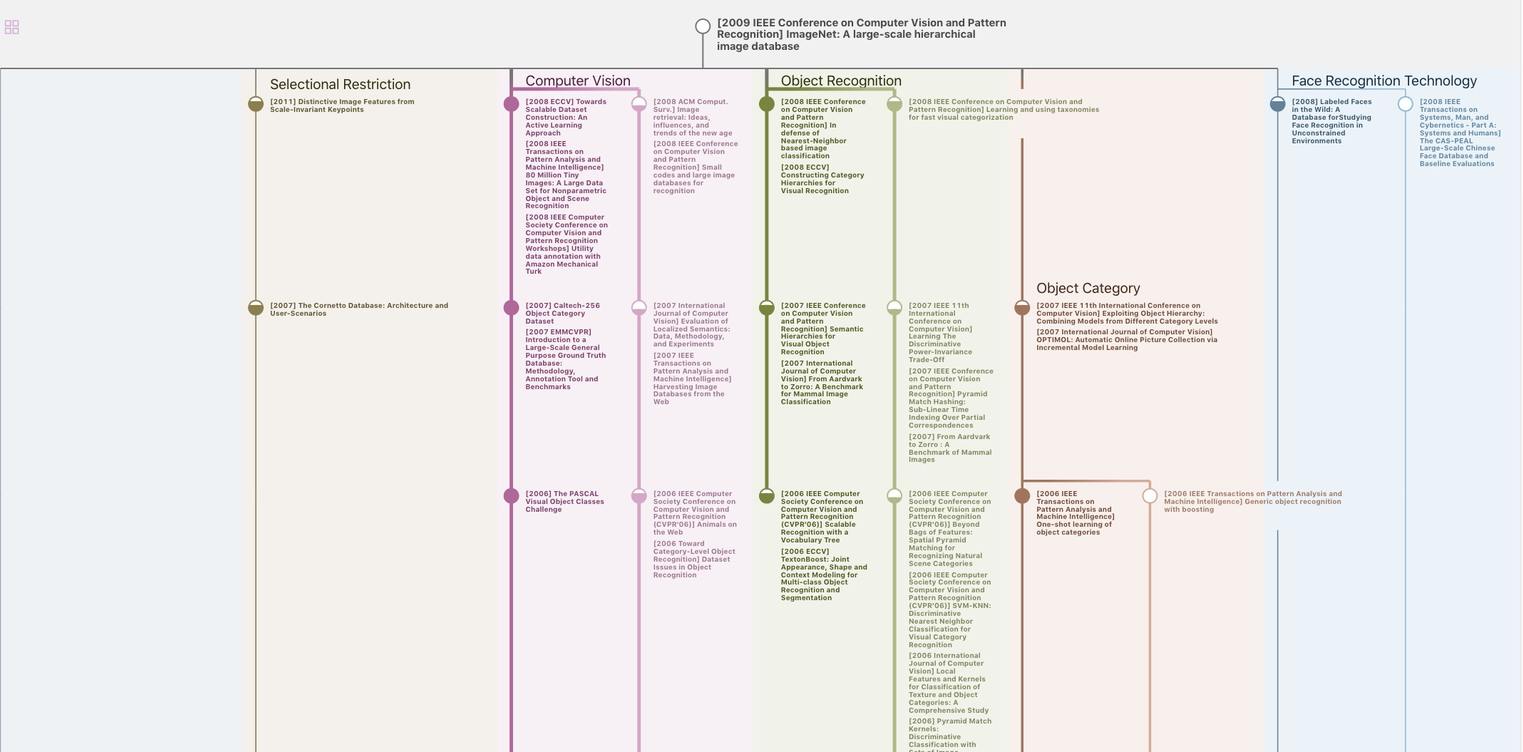
生成溯源树,研究论文发展脉络
Chat Paper
正在生成论文摘要