Deep Predictive Autonomous Driving Using Multi-Agent Joint Trajectory Prediction and Traffic Rules
IEEE/RJS International Conference on Intelligent RObots and Systems(2019)
摘要
Autonomous driving is a challenging problem because the autonomous vehicle must understand complex and dynamic environment. This understanding consists of predicting future behavior of nearby vehicles and recognizing predefined rules. It is observed that not all rules have equivalent values, and the priority of the rules may change depending on the situation or the driver's driving style. In this work, we jointly reason both a future trajectories of vehicles and degree of satisfaction of each rule in the deep learning framework. Joint reasoning allows modeling interactions between vehicles, and leads to better prediction results. A rule is represented as a signal temporal logic (STL) formula, and a robustness slackness, a margin to the satisfaction of the rule, is predicted for the both autonomous and other vehicle, in addition to future trajectories. Learned robustness slackness decides which rule should be prioritized for the given situation for the autonomous vehicle, and filter out non-valid predicted trajectories for surrounding vehicles. The predicted information from the deep learning framework is used in model predictive control (MPC), which allows the autonomous vehicle navigate efficiently and safely. We test the feasibility of our approach in publicly available NGSIM datasets. Proposed method shows a driving style similar to the human one and considers the safety related to the rules through the future prediction of the surrounding vehicles.
更多查看译文
关键词
future trajectories,nonvalid predicted trajectories,deep learning framework,model predictive control,autonomous vehicle navigation,driving style,multiagent joint trajectory prediction,traffic rules,dynamic environment,nearby vehicles,joint reasoning,signal temporal logic formula,robustness slackness learning,deep predictive autonomous driving,complex environment,predefined rules recognition,driver driving style,NGSIM datasets,safety
AI 理解论文
溯源树
样例
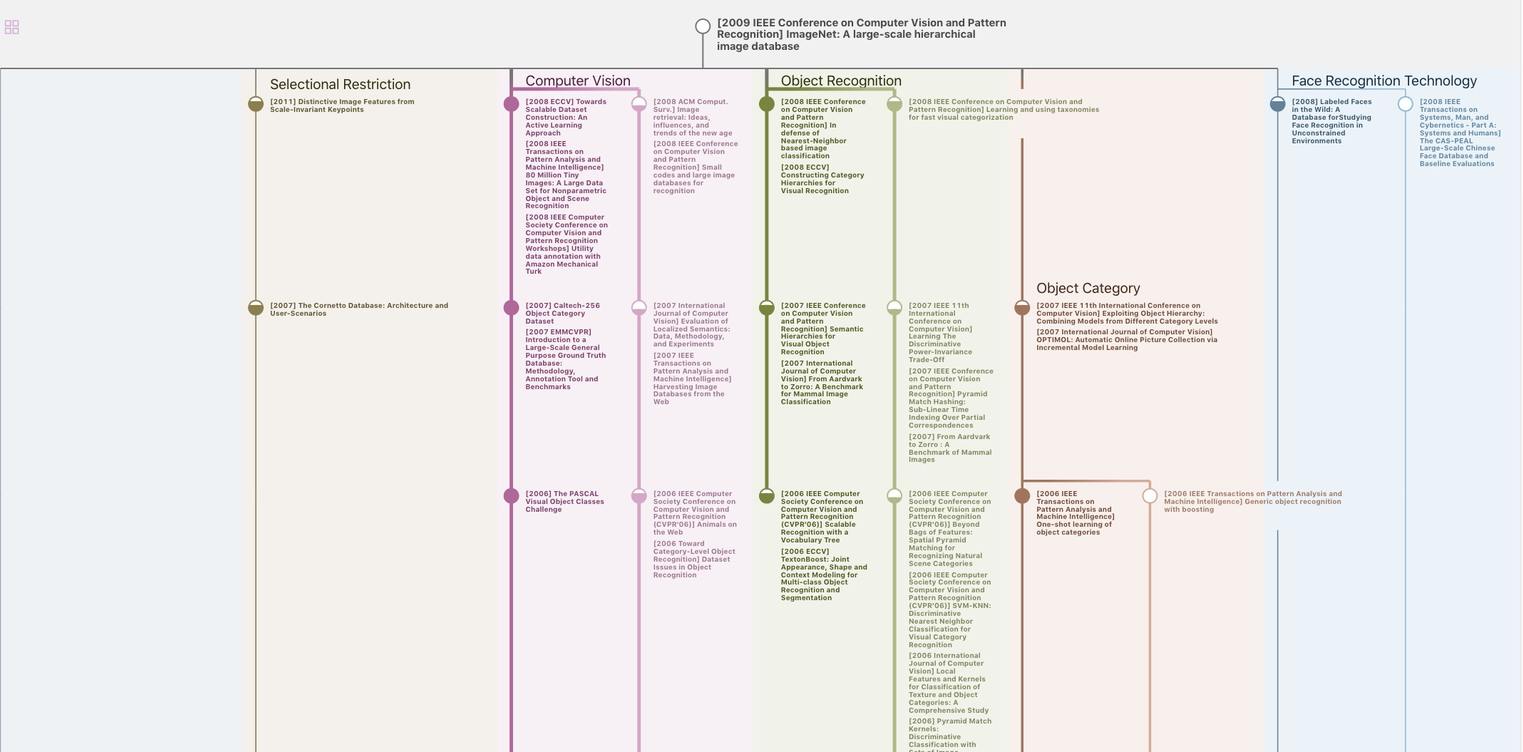
生成溯源树,研究论文发展脉络
Chat Paper
正在生成论文摘要