Localization And Mapping Using Instance-Specific Mesh Models
2019 IEEE/RSJ INTERNATIONAL CONFERENCE ON INTELLIGENT ROBOTS AND SYSTEMS (IROS)(2019)
摘要
This paper focuses on building semantic maps, containing object poses and shapes, using a monocular camera. This is an important problem because robots need rich understanding of geometry and context if they are to shape the future of transportation, construction, and agriculture. Our contribution is an instance-specific mesh model of object shape that can be optimized online based on semantic information extracted from camera images. Multi-view constraints on the object shape are obtained by detecting objects and extracting category-specific keypoints and segmentation masks. We show that the errors between projections of the mesh model and the observed keypoints and masks can be differentiated in order to obtain accurate instance-specific object shapes. We evaluate the performance of the proposed approach in simulation and on the KITTI dataset by building maps of car poses and shapes.
更多查看译文
关键词
KITTI dataset,category-specific keypoints,instance-specific object shapes,car poses,segmentation masks,camera images,semantic information,object shape,instance-specific mesh model,monocular camera,object poses
AI 理解论文
溯源树
样例
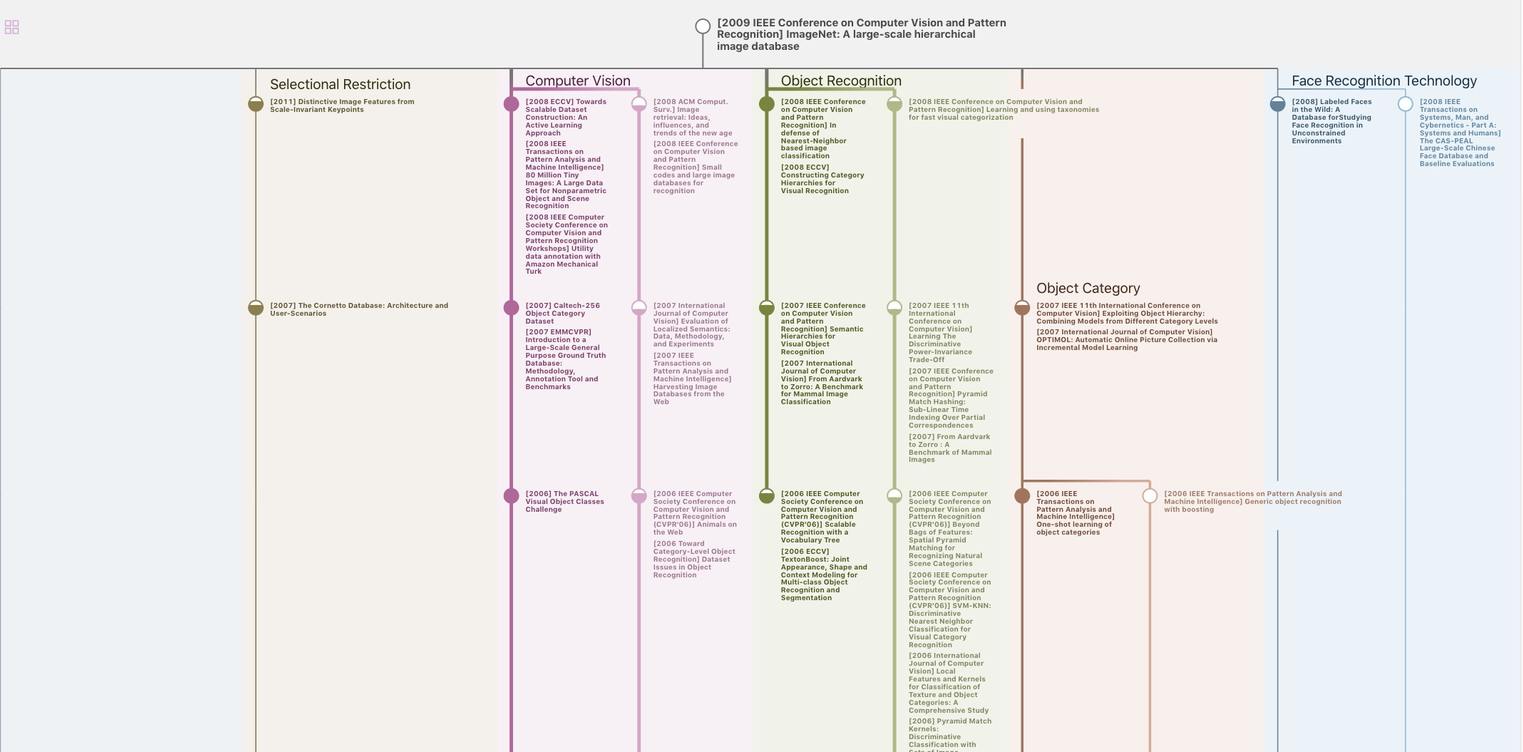
生成溯源树,研究论文发展脉络
Chat Paper
正在生成论文摘要