Robust Outdoor Self-localization In Changing Environments
2019 IEEE/RSJ International Conference on Intelligent Robots and Systems (IROS)(2019)
摘要
In outdoor scenarios changing conditions (e.g., seasonal, weather and lighting effects) have a substantial impact on the appearance of a scene, which often prevents successful visual localization. The application of an unsupervised Slow Feature Analysis (SFA) on the images captured by an autonomous robot enables self-localization from a single image. However, changes occurring during the training phase or over a more extended period can affect the learned representations. To address the problem, we propose to join long-term recordings from an outdoor environment based on their position correspondences. The established hierarchical model trained on raw images performs well, but as an extension, we extract Fourier components of the views and use that for learning of spatial representations, which reduces the computation time and makes it adequate to run on an ARM embedded system. We present the experimental results from a simulated environment and real-world outdoor recordings collected over a full year, which has effects like different day time, weather, seasons and dynamic objects. Results show an increasing invariance w.r.t. changing conditions over time, thus an outdoor robot can improve its localization performance during operation.
更多查看译文
关键词
robust outdoor self-localization,outdoor scenarios,visual localization,unsupervised Slow Feature Analysis,unsupervised SFA,autonomous robot,representation learning,outdoor environment,Fourier components,ARM embedded system,outdoor robot,localization performance,changing environments
AI 理解论文
溯源树
样例
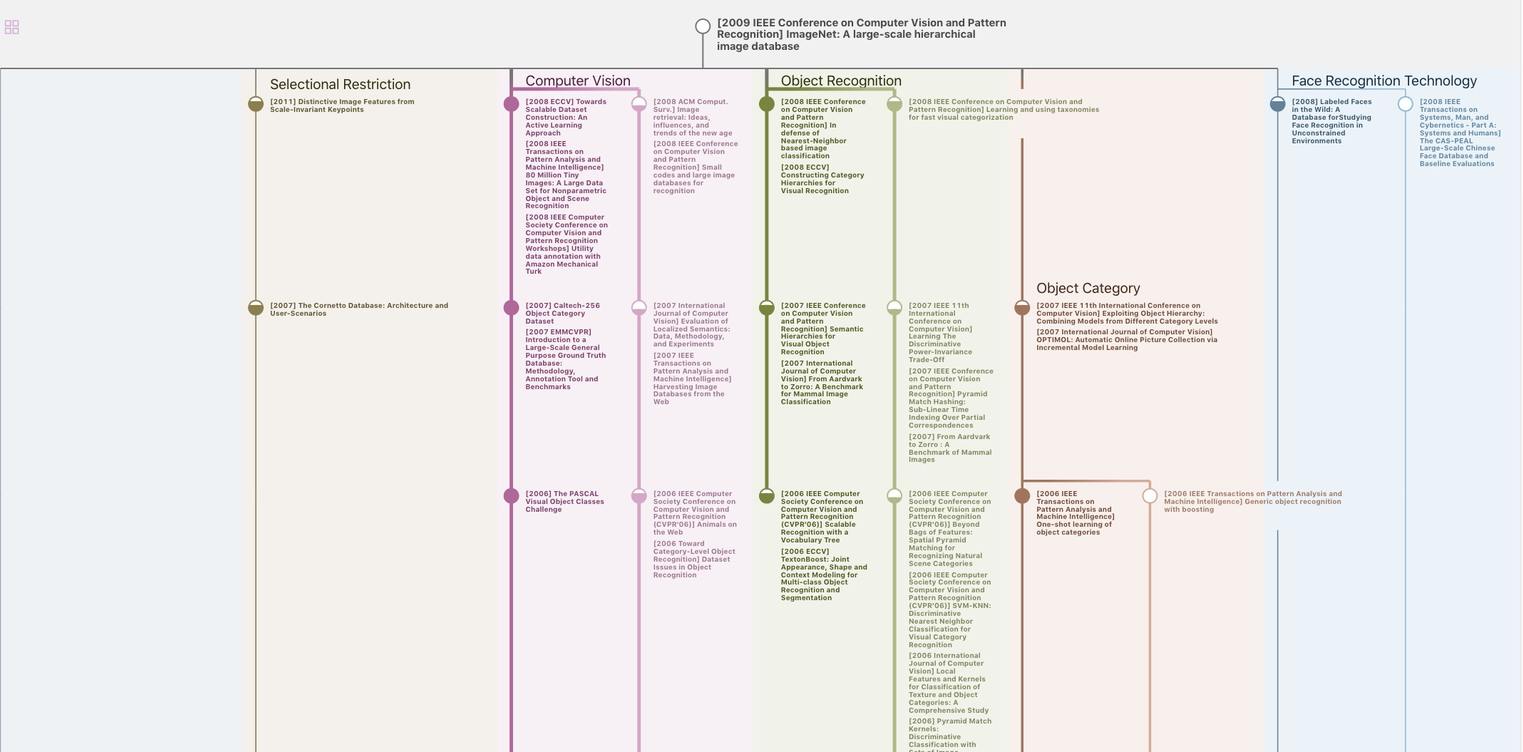
生成溯源树,研究论文发展脉络
Chat Paper
正在生成论文摘要