Leveraging Uncertainties for Deep Multi-modal Object Detection in Autonomous Driving
2020 IEEE INTELLIGENT VEHICLES SYMPOSIUM (IV)(2020)
摘要
This work presents a probabilistic deep neural network that combines LiDAR point clouds and RGB camera images for robust, accurate 3D object detection. We explicitly model uncertainties in the classification and regression tasks, and leverage uncertainties to train the fusion network via a sampling mechanism. We validate our method on three datasets with challenging real-world driving scenarios. Experimental results show that the predicted uncertainties reflect complex environmental uncertainty like difficulties of a human expert to label objects. The results also show that our method consistently improves the Average Precision by up to 7% compared to the baseline method. When sensors are temporally misaligned, the sampling method improves the Average Precision by up to 20%, showing its high robustness against noisy sensor inputs.
更多查看译文
关键词
autonomous driving,uncertainties,detection,multi-modal
AI 理解论文
溯源树
样例
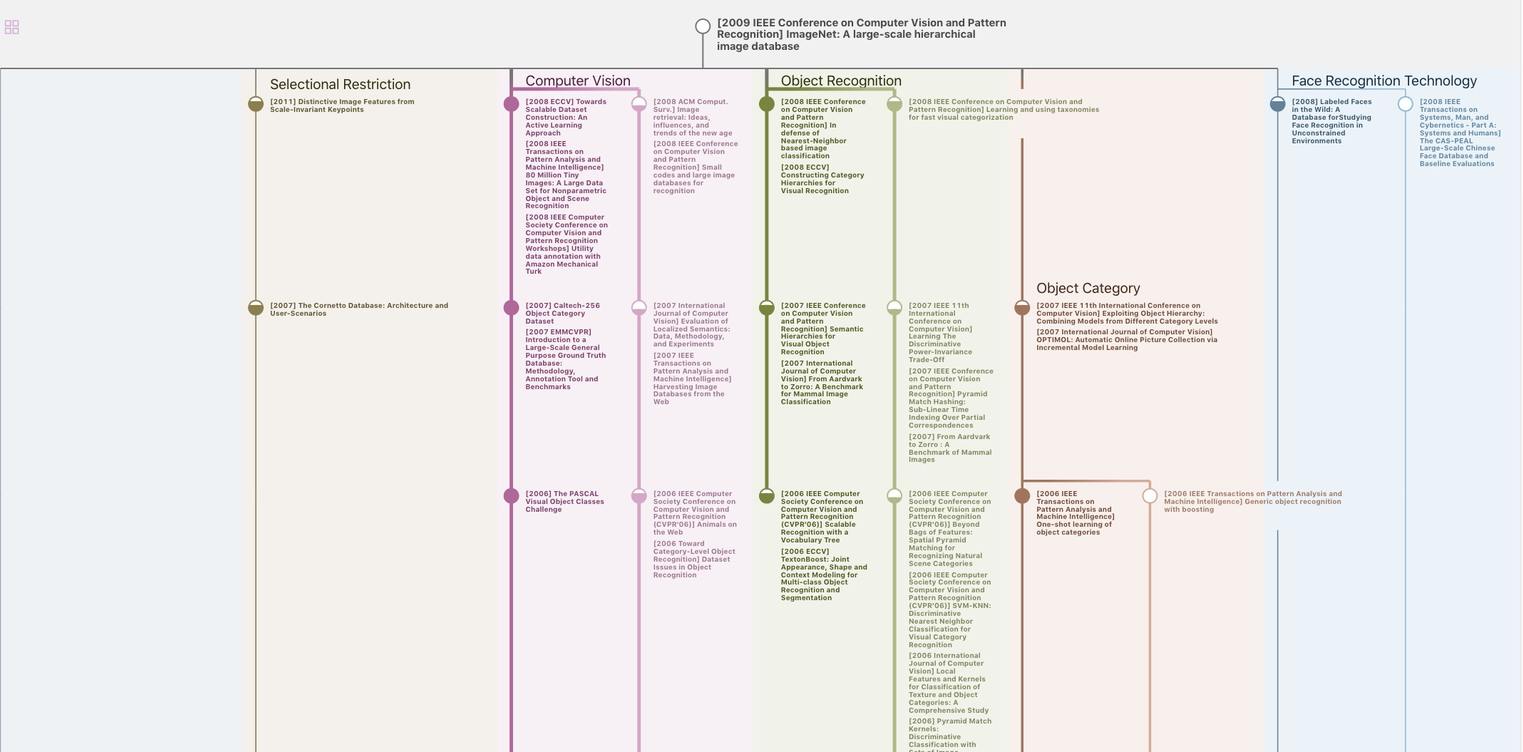
生成溯源树,研究论文发展脉络
Chat Paper
正在生成论文摘要