NAPLES;Mining the lead-lag Relationship from Non-synchronous and High-frequency Data
arxiv(2020)
摘要
In time-series analysis, the term "lead-lag effect" is used to describe a delayed effect on a given time series caused by another time series. lead-lag effects are ubiquitous in practice and are specifically critical in formulating investment strategies in high-frequency trading. At present, there are three major challenges in analyzing the lead-lag effects. First, in practical applications, not all time series are observed synchronously. Second, the size of the relevant dataset and rate of change of the environment is increasingly faster, and it is becoming more difficult to complete the computation within a particular time limit. Third, some lead-lag effects are time-varying and only last for a short period, and their delay lengths are often affected by external factors. In this paper, we propose NAPLES (Negative And Positive lead-lag EStimator), a new statistical measure that resolves all these problems. Through experiments on artificial and real datasets, we demonstrate that NAPLES has a strong correlation with the actual lead-lag effects, including those triggered by significant macroeconomic announcements.
更多查看译文
关键词
data,lead-lag,non-synchronous,high-frequency
AI 理解论文
溯源树
样例
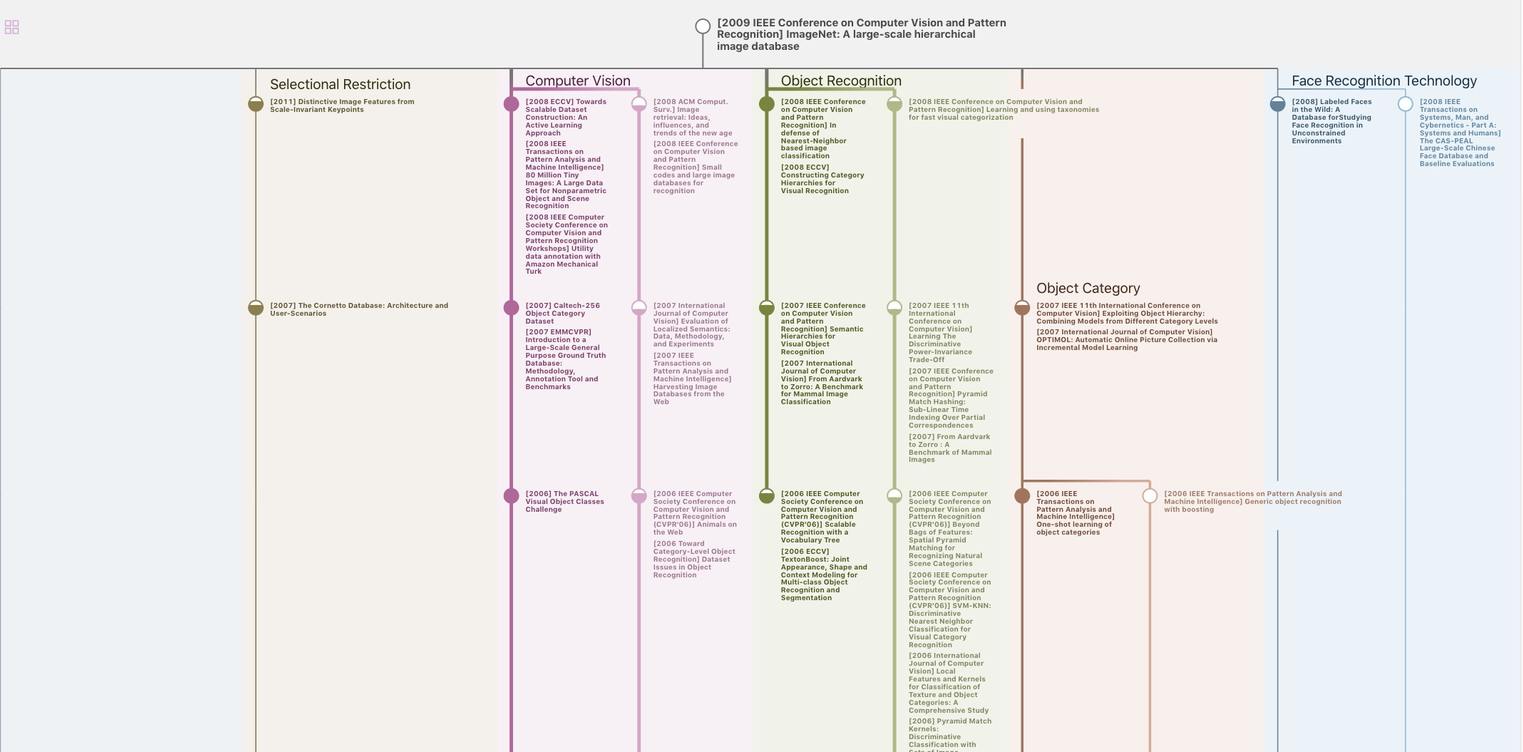
生成溯源树,研究论文发展脉络
Chat Paper
正在生成论文摘要