Finite-Sample Analysis of Contractive Stochastic Approximation Using Smooth Convex Envelopes
NIPS 2020(2020)
摘要
Stochastic Approximation (SA) is a popular approach for solving fixed point equations where the information is corrupted by noise. In this paper, we consider an SA involving a contraction mapping with respect to an arbitrary norm, and show its finite-sample bound for using either constant or diminishing step sizes. The idea is to construct a smooth Lyapunov function using the generalized Moreau envelope, and show that the iterates of SA are contracting in expectation with respect to that Lyapunov function. The result is applicable to various Reinforcement Learning (RL) algorithms. In particular, we use it to establish the first-known convergence rate of the V-trace algorithm for the off-policy TD-Learning [15], and improve the existing bound for the tabular $Q$-Learning algorithm. Further, for these two applications, our construction of the Lyapunov functions results in only a logarithmic dependence of the convergence bound on the state-space dimension.
更多查看译文
AI 理解论文
溯源树
样例
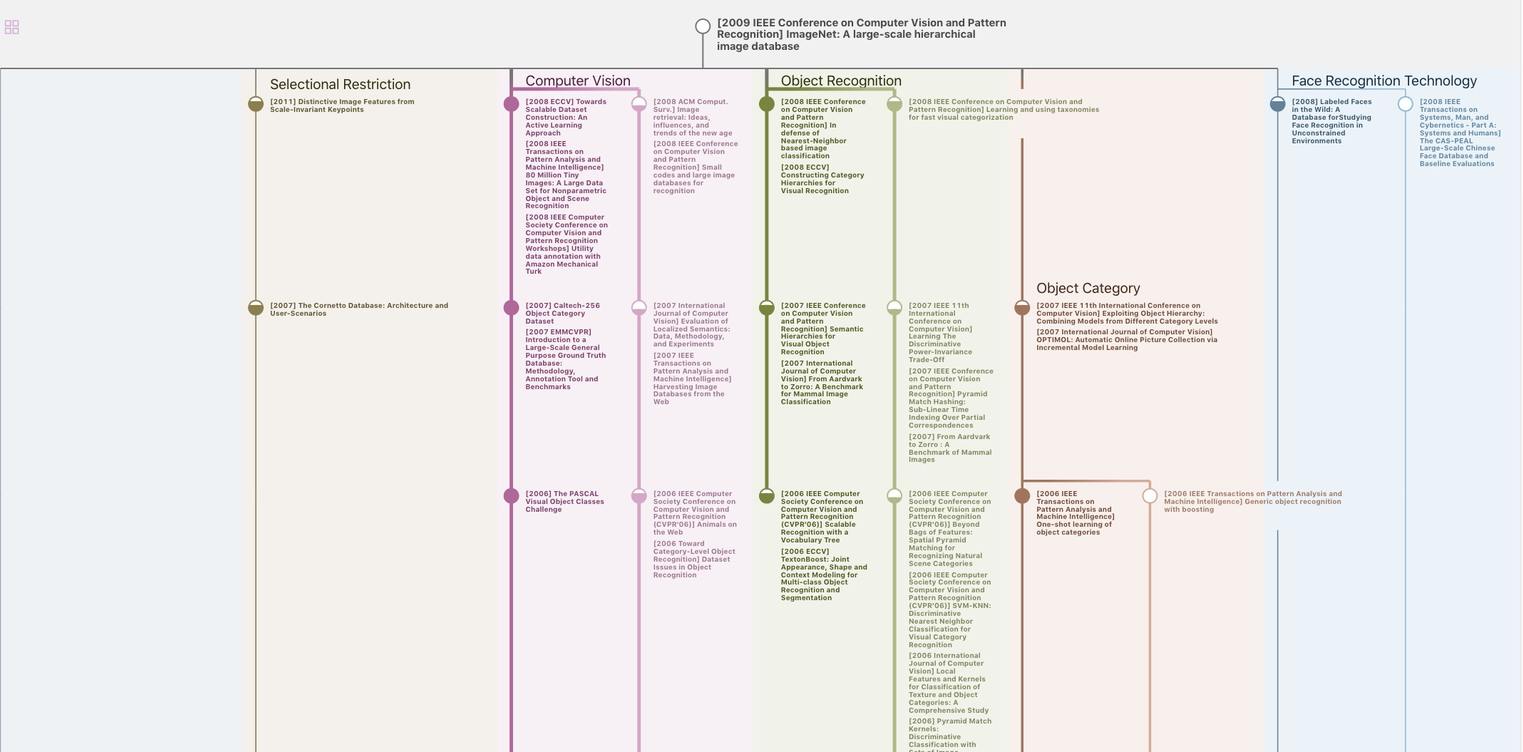
生成溯源树,研究论文发展脉络
Chat Paper
正在生成论文摘要