Using extremal events to characterize noisy time series
Journal of Mathematical Biology(2020)
摘要
Experimental time series provide an informative window into the underlying dynamical system, and the timing of the extrema of a time series (or its derivative) contains information about its structure. However, the time series often contain significant measurement errors. We describe a method for characterizing a time series for any assumed level of measurement error ε by a sequence of intervals, each of which is guaranteed to contain an extremum for any function that ε -approximates the time series. Based on the merge tree of a continuous function, we define a new object called the normalized branch decomposition, which allows us to compute intervals for any level ε . We show that there is a well-defined total order on these intervals for a single time series, and that it is naturally extended to a partial order across a collection of time series comprising a dataset. We use the order of the extracted intervals in two applications. First, the partial order describing a single dataset can be used to pattern match against switching model output (Cummins et al. in SIAM J Appl Dyn Syst 17(2):1589–1616, 2018), which allows the rejection of a network model. Second, the comparison between graph distances of the partial orders of different datasets can be used to quantify similarity between biological replicates.
更多查看译文
关键词
Time series, Merge trees, Order of extrema, Partial orders, 05C12, 06A06, 37M10
AI 理解论文
溯源树
样例
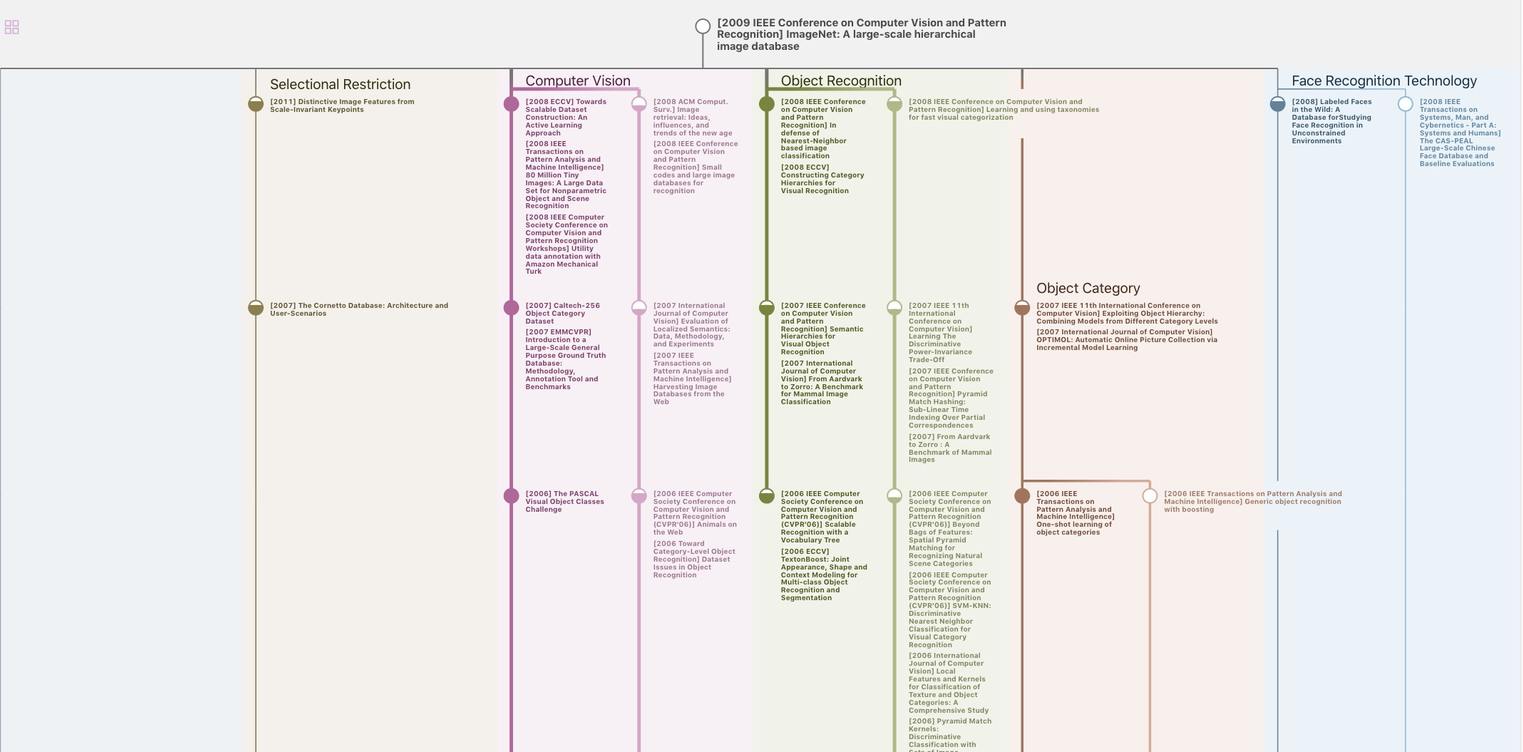
生成溯源树,研究论文发展脉络
Chat Paper
正在生成论文摘要