Novel Regional Activity Representation With Constrained Canonical Correlation Analysis for Brain Connectivity Network Estimation.
IEEE Transactions on Medical Imaging(2020)
摘要
Inferring brain connectivity networks from fMRI data can take place at the Region of Interest (ROI) or voxel level. With most ROI-based approaches, the signals from same-ROI voxels are simply averaged, neglecting any inhomogeneity in each ROI and assuming that the same voxels will interact with different ROIs in a similar manner. In this paper, we propose a novel method of representing ROI activity and estimating brain connectivity that takes into account the regionally-specific nature of brain activity, the spatial location of concentrated activity, and activity in other ROIs. The proposed method is able to integrate intrinsic regional structures into a network modelling framework, which we call local activity constrained canonical correlation analysis (LA-cCCA). We evaluated LA-cCCA on both simulated and real fMRI data. The simulation results demonstrated that LA-cCCA had improved accuracy of the estimated brain connectivity networks compared to the average-signal or Principal Component Analysis (PCA)-based correlation methods and the Canonical Correlation Analysis (CCA) method. We further examined the performance of LA-cCCA on real fMRI data set from the Human Connectome Project. LA-cCCA outperformed the other three approaches in terms of connectivity reproducibility. The proposed method explores the potentials of regional activity representation and is a reliable model for connectivity network estimation. It may serve as a promising tool for studying both the healthy and diseased brain.
更多查看译文
关键词
Brain modeling,Functional magnetic resonance imaging,Correlation,Clustering algorithms,Mathematical model,Analytical models
AI 理解论文
溯源树
样例
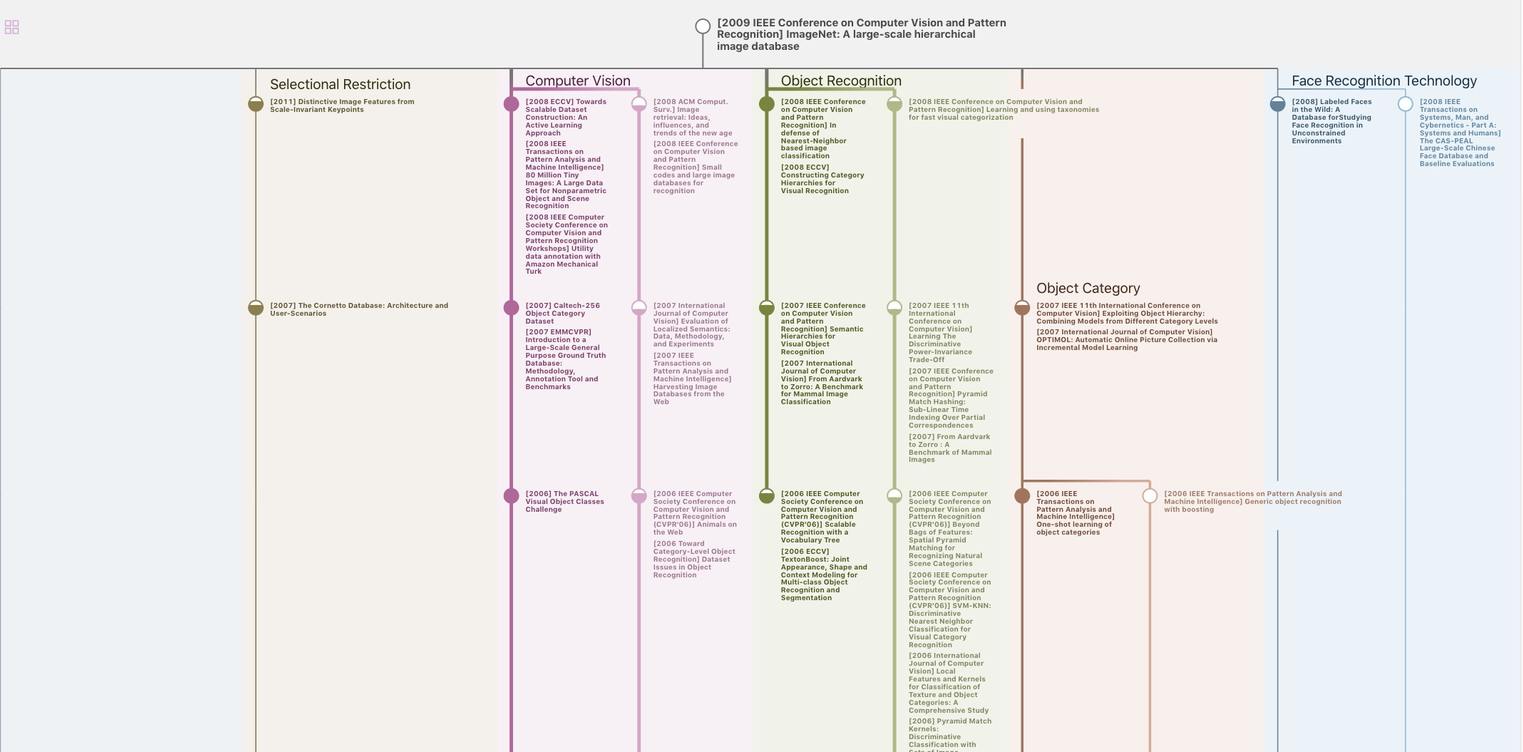
生成溯源树,研究论文发展脉络
Chat Paper
正在生成论文摘要