Acoustic anomaly detection via latent regularized gaussian mixture generative adversarial networks
arxiv(2020)
摘要
Acoustic anomaly detection aims at distinguishing abnormal acoustic signals from the normal ones. It suffers from the class imbalance issue and the lacking in the abnormal instances. In addition, collecting all kinds of abnormal or unknown samples for training purpose is impractical and timeconsuming. In this paper, a novel Gaussian Mixture Generative Adversarial Network (GMGAN) is proposed under semi-supervised learning framework, in which the underlying structure of training data is not only captured in spectrogram reconstruction space, but also can be further restricted in the space of latent representation in a discriminant manner. Experiments show that our model has clear superiority over previous methods, and achieves the state-of-the-art results on DCASE dataset.
更多查看译文
关键词
acoustic anomaly detection,generative adversarial networks,gaussian mixture
AI 理解论文
溯源树
样例
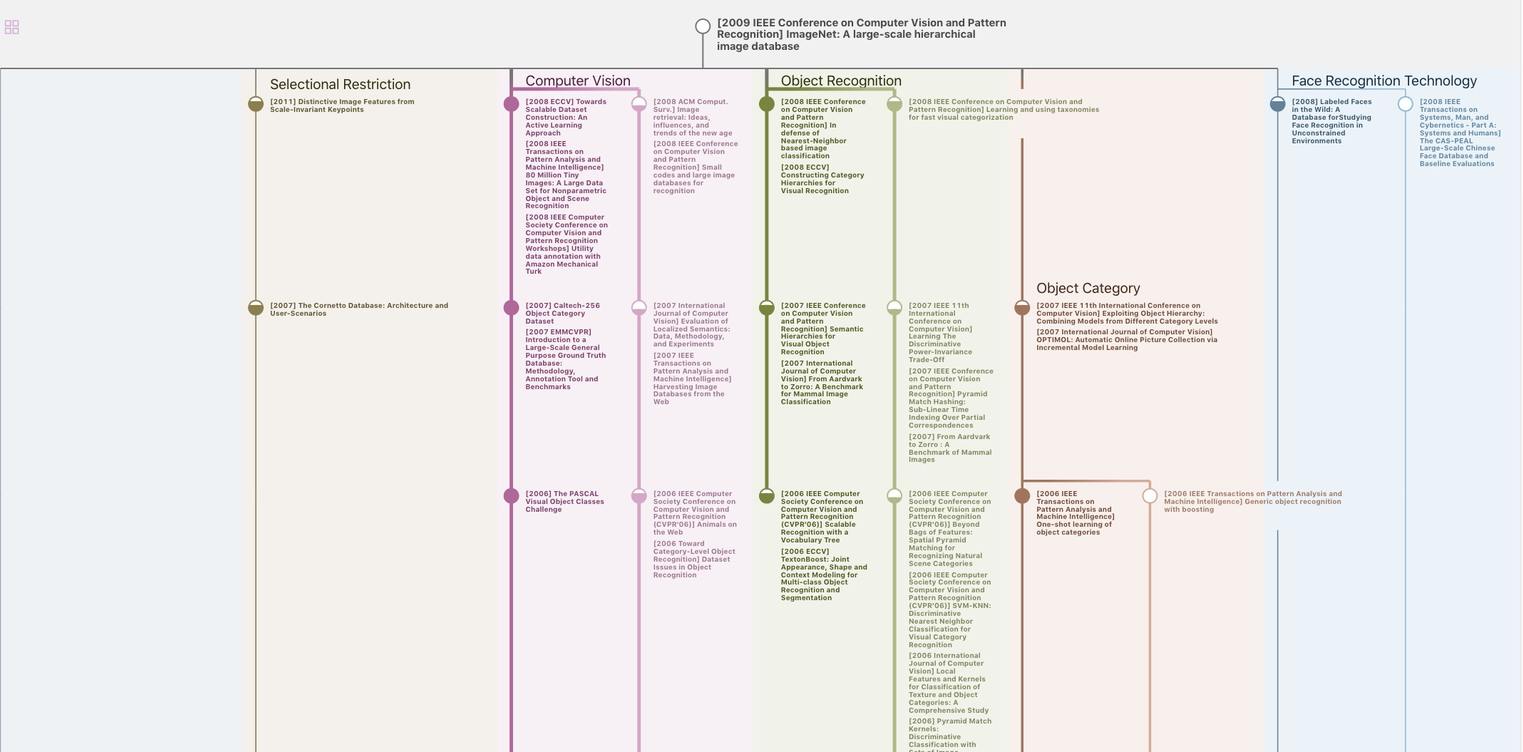
生成溯源树,研究论文发展脉络
Chat Paper
正在生成论文摘要