Alpine: Active Link Prediction Using Network Embedding
APPLIED SCIENCES-BASEL(2021)
摘要
Many real-world problems can be formalized as predicting links in a partially observed network. Examples include Facebook friendship suggestions, the prediction of protein-protein interactions, and the identification of hidden relationships in a crime network. Several link prediction algorithms, notably those recently introduced using network embedding, are capable of doing this by just relying on the observed part of the network. Often, whether two nodes are linked can be queried, albeit at a substantial cost (e.g., by questionnaires, wet lab experiments, or undercover work). Such additional information can improve the link prediction accuracy, but owing to the cost, the queries must be made with due consideration. Thus, we argue that an active learning approach is of great potential interest and developed ALPINE (Active Link Prediction usIng Network Embedding), a framework that identifies the most useful link status by estimating the improvement in link prediction accuracy to be gained by querying it. We proposed several query strategies for use in combination with ALPINE, inspired by the optimal experimental design and active learning literature. Experimental results on real data not only showed that ALPINE was scalable and boosted link prediction accuracy with far fewer queries, but also shed light on the relative merits of the strategies, providing actionable guidance for practitioners.
更多查看译文
关键词
active learning, link prediction, network embedding, partially observed networks, optimal experimental design
AI 理解论文
溯源树
样例
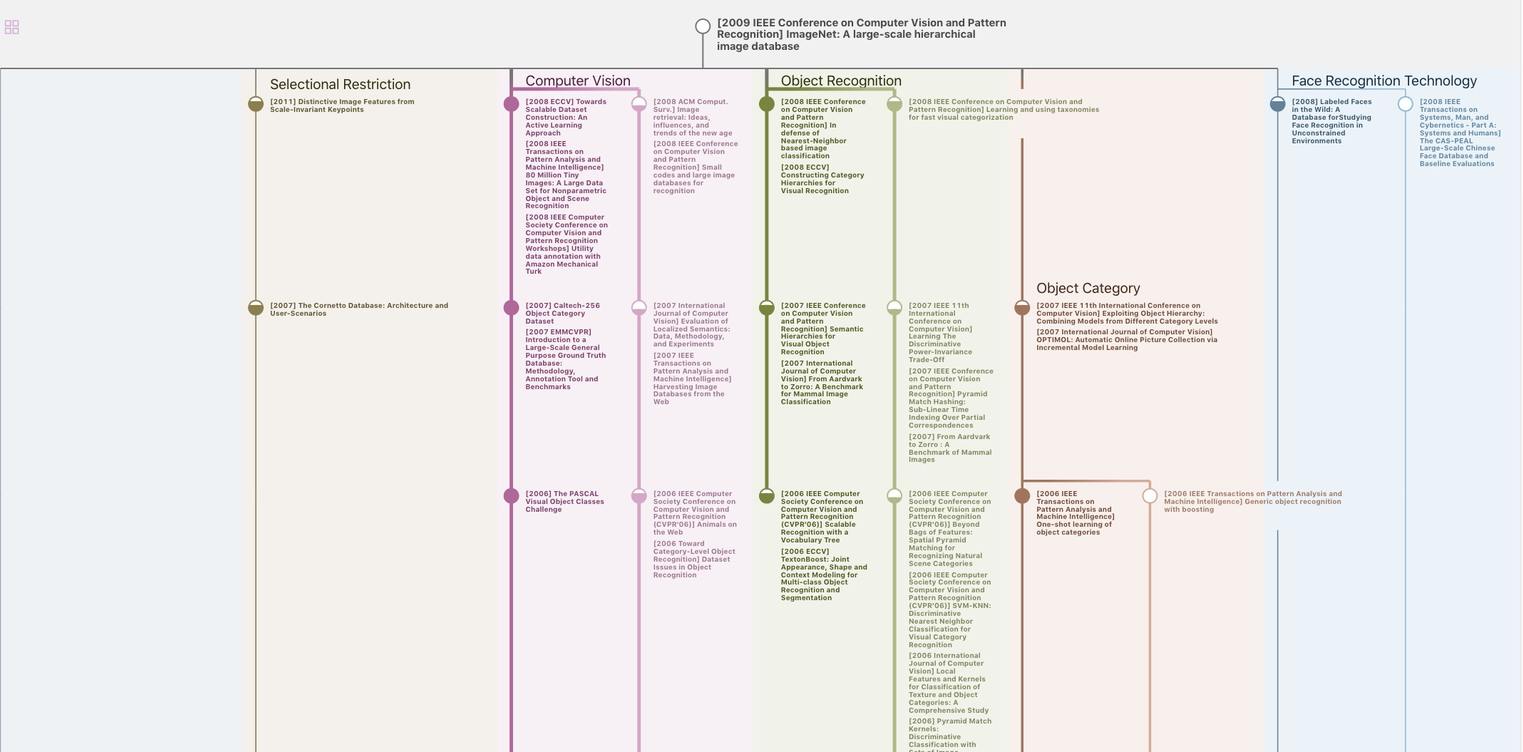
生成溯源树,研究论文发展脉络
Chat Paper
正在生成论文摘要